A Structure-Guided Molecular Network Strategy for Global Untargeted Metabolomics Data Annotation
ANALYTICAL CHEMISTRY(2023)
摘要
Large-scale metabolite annotation is a bottleneck inuntargetedmetabolomics. Here, we present a structure-guided molecular networkstrategy (SGMNS) for deep annotation of untargeted ultra-performanceliquid chromatography-high resolution mass spectrometry (MS) metabolomicsdata. Different from the current network-based metabolite annotationmethod, SGMNS is based on a global connectivity molecular network(GCMN), which was constructed by molecular fingerprint similarityof chemical structures in metabolome databases. Neighbor metaboliteswith similar structures in GCMN are expected to produce similar spectra.Network annotation propagation of SGMNS is performed using known metabolitesas seeds. The experimental MS/MS spectra of seeds are assigned tocorresponding neighbor metabolites in GCMN as their "pseudo"spectra; the propagation is done by searching predicted retentiontimes, MS1, and "pseudo" spectra againstmetabolite features in untargeted metabolomics data. Then, the annotatedmetabolite features were used as new seeds for annotation propagationagain. Performance evaluation of SGMNS showed its unique advantagesfor metabolome annotation. The developed method was applied to annotatesix typical biological samples; a total of 701, 1557, 1147, 1095,1237, and 2041 metabolites were annotated from the cell, feces, plasma(NIST SRM 1950), tissue, urine, and their pooled sample, respectively,and the annotation accuracy was >83% with RSD <2%. The resultsshow that SGMNS fully exploits the chemical space of the existingmetabolomes for metabolite deep annotation and overcomes the shortcomingof insufficient reference MS/MS spectra.
更多查看译文
关键词
molecular network strategy,structure-guided
AI 理解论文
溯源树
样例
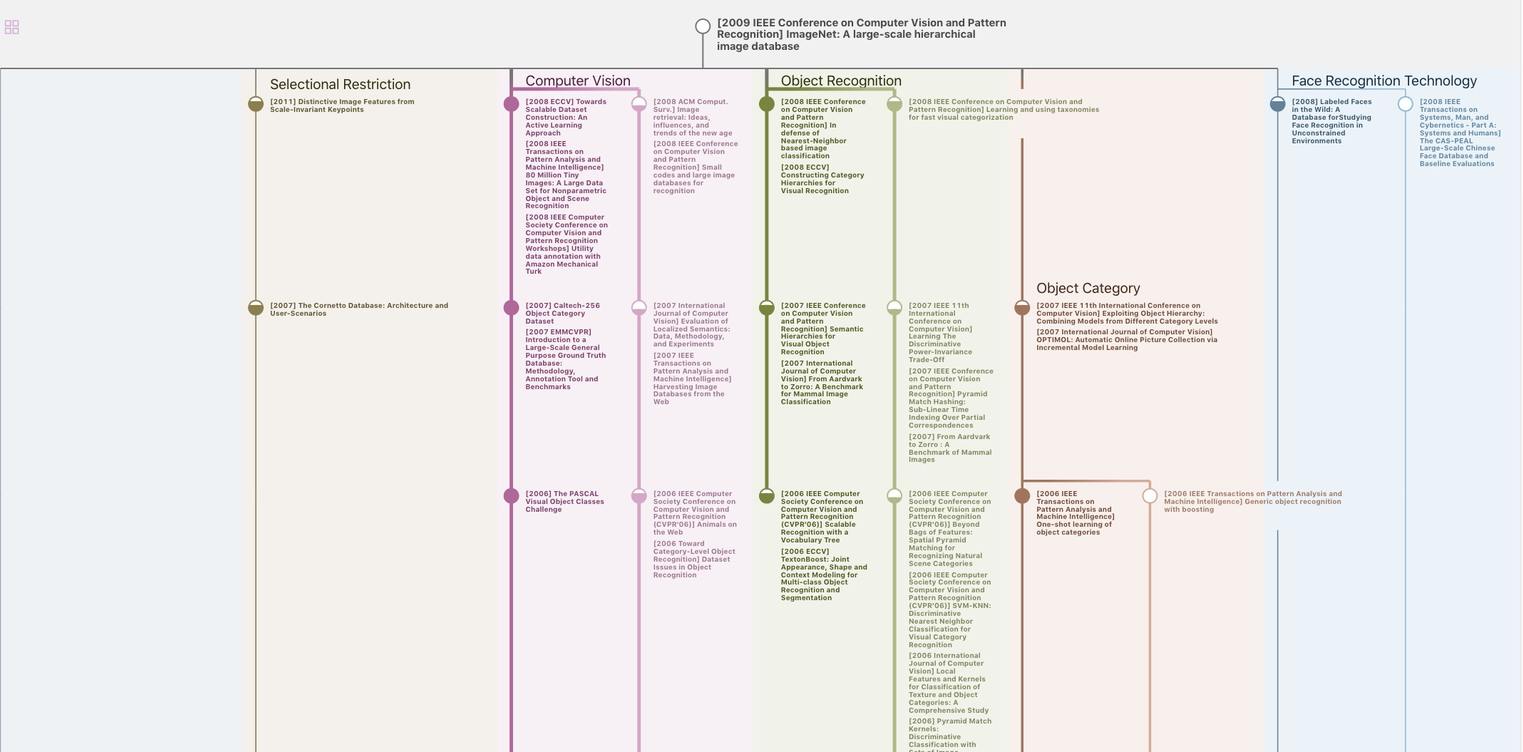
生成溯源树,研究论文发展脉络
Chat Paper
正在生成论文摘要