Cardiac surgery risk prediction using ensemble machine learning to incorporate legacy risk scores: A benchmarking study
DIGITAL HEALTH(2023)
摘要
ObjectiveThe introduction of new clinical risk scores (e.g. European System for Cardiac Operative Risk Evaluation (EuroSCORE) II) superseding original scores (e.g. EuroSCORE I) with different variable sets typically result in disparate datasets due to high levels of missingness for new score variables prior to time of adoption. Little is known about the use of ensemble learning to incorporate disparate data from legacy scores. We tested the hypothesised that Homogenenous and Heterogeneous Machine Learning (ML) ensembles will have better performance than ensembles of Dynamic Model Averaging (DMA) for combining knowledge from EuroSCORE I legacy data with EuroSCORE II data to predict cardiac surgery risk. MethodsUsing the National Adult Cardiac Surgery Audit dataset, we trained 12 different base learner models, based on two different variable sets from either EuroSCORE I (LogES) or EuroScore II (ES II), partitioned by the time of score adoption (1996-2016 or 2012-2016) and evaluated on holdout set (2017-2019). These base learner models were ensembled using nine different combinations of six ML algorithms to produce homogeneous or heterogeneous ensembles. Performance was assessed using a consensus metric. ResultsXgboost homogenous ensemble (HE) was the highest performing model (clinical effectiveness metric (CEM) 0.725) with area under the curve (AUC) (0.8327; 95% confidence interval (CI) 0.8323-0.8329) followed by Random Forest HE (CEM 0.723; AUC 0.8325; 95%CI 0.8320-0.8326). Across different heterogenous ensembles, significantly better performance was obtained by combining siloed datasets across time (CEM 0.720) than building ensembles of either 1996-2011 (t-test adjusted, p = 1.67x10(-6)) or 2012-2019 (t-test adjusted, p = 1.35x10(-193)) datasets alone. ConclusionsBoth homogenous and heterogenous ML ensembles performed significantly better than DMA ensemble of Bayesian Update models. Time-dependent ensemble combination of variables, having differing qualities according to time of score adoption, enabled previously siloed data to be combined, leading to increased power, clinical interpretability of variables and usage of data.
更多查看译文
关键词
Cardiac surgery, risk prediction, machine learning, mortality, ensemble learning, dynamic model averaging, legacy scores, multi-modal data
AI 理解论文
溯源树
样例
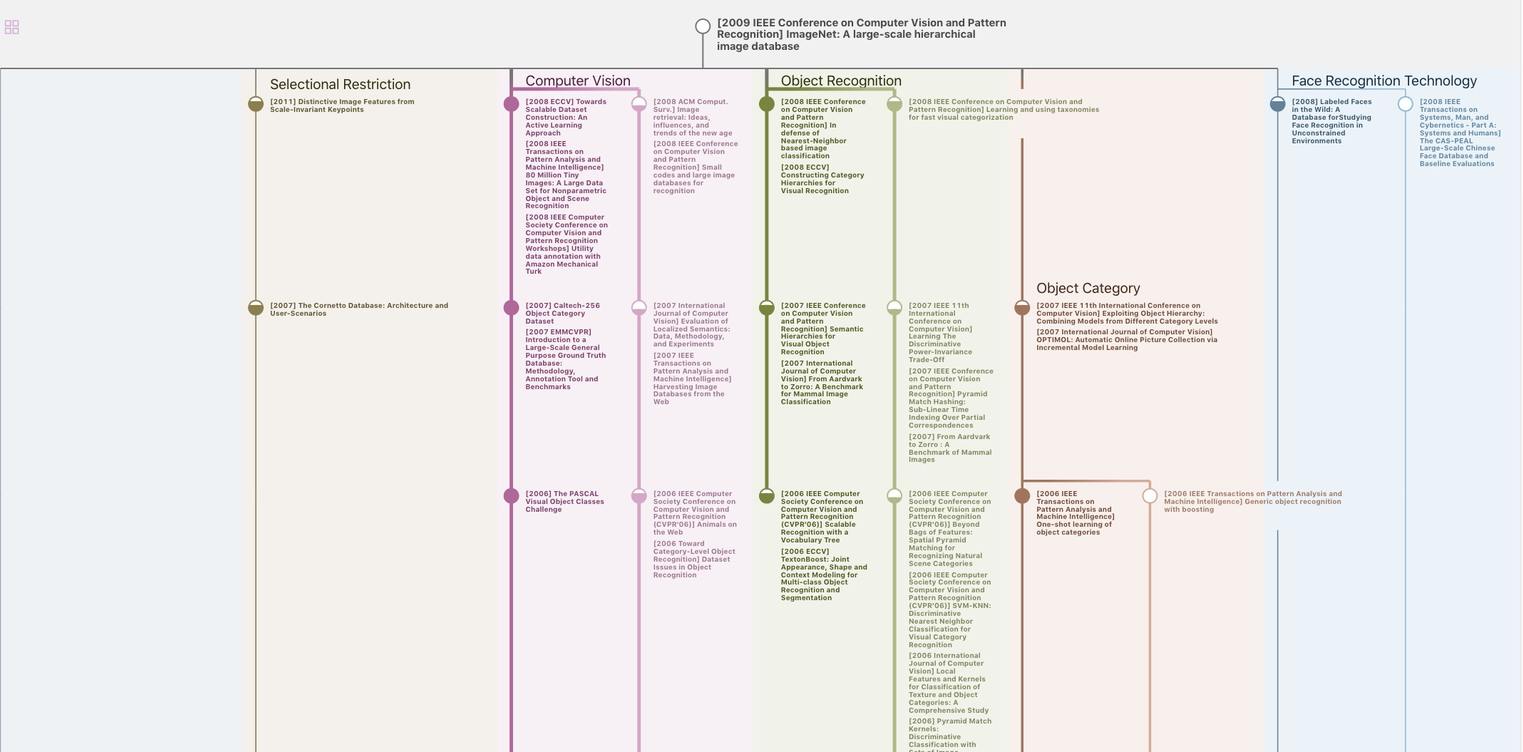
生成溯源树,研究论文发展脉络
Chat Paper
正在生成论文摘要