Spatiotemporal Modeling Encounters 3D Medical Image Analysis: Slice-Shift UNet with Multi-View Fusion
CoRR(2023)
摘要
As a fundamental part of computational healthcare, Computer Tomography (CT) and Magnetic Resonance Imaging (MRI) provide volumetric data, making the development of algorithms for 3D image analysis a necessity. Despite being computationally cheap, 2D Convolutional Neural Networks can only extract spatial information. In contrast, 3D CNNs can extract three-dimensional features, but they have higher computational costs and latency, which is a limitation for clinical practice that requires fast and efficient models. Inspired by the field of video action recognition we propose a new 2D-based model dubbed Slice SHift UNet (SSH-UNet) which encodes three-dimensional features at 2D CNN's complexity. More precisely multi-view features are collaboratively learned by performing 2D convolutions along the three orthogonal planes of a volume and imposing a weights-sharing mechanism. The third dimension, which is neglected by the 2D convolution, is reincorporated by shifting a portion of the feature maps along the slices' axis. The effectiveness of our approach is validated in Multi-Modality Abdominal Multi-Organ Segmentation (AMOS) and Multi-Atlas Labeling Beyond the Cranial Vault (BTCV) datasets, showing that SSH-UNet is more efficient while on par in performance with state-of-the-art architectures.
更多查看译文
关键词
3d,image analysis
AI 理解论文
溯源树
样例
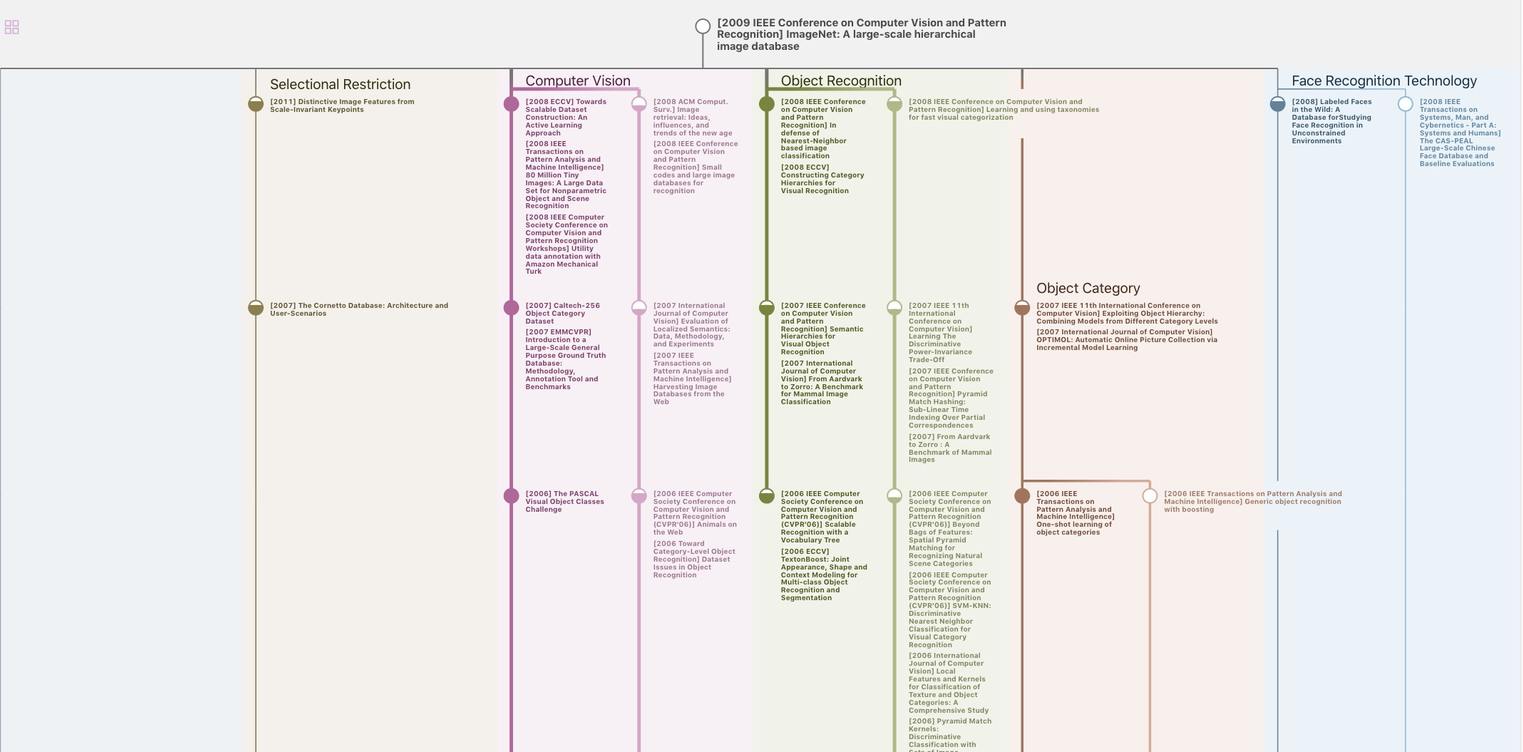
生成溯源树,研究论文发展脉络
Chat Paper
正在生成论文摘要