Epsilon*: Privacy Metric for Machine Learning Models
CoRR(2023)
摘要
We introduce Epsilon*, a new privacy metric for measuring the privacy risk of a single model instance prior to, during, or after deployment of privacy mitigation strategies. The metric does not require access to the training data sampling or model training algorithm. Epsilon* is a function of true positive and false positive rates in a hypothesis test used by an adversary in a membership inference attack. We distinguish between quantifying the privacy loss of a trained model instance and quantifying the privacy loss of the training mechanism which produces this model instance. Existing approaches in the privacy auditing literature provide lower bounds for the latter, while our metric provides a lower bound for the former by relying on an (${\epsilon}$,${\delta}$)-type of quantification of the privacy of the trained model instance. We establish a relationship between these lower bounds and show how to implement Epsilon* to avoid numerical and noise amplification instability. We further show in experiments on benchmark public data sets that Epsilon* is sensitive to privacy risk mitigation by training with differential privacy (DP), where the value of Epsilon* is reduced by up to 800% compared to the Epsilon* values of non-DP trained baseline models. This metric allows privacy auditors to be independent of model owners, and enables all decision-makers to visualize the privacy-utility landscape to make informed decisions regarding the trade-offs between model privacy and utility.
更多查看译文
关键词
privacy metric,machine learning,models
AI 理解论文
溯源树
样例
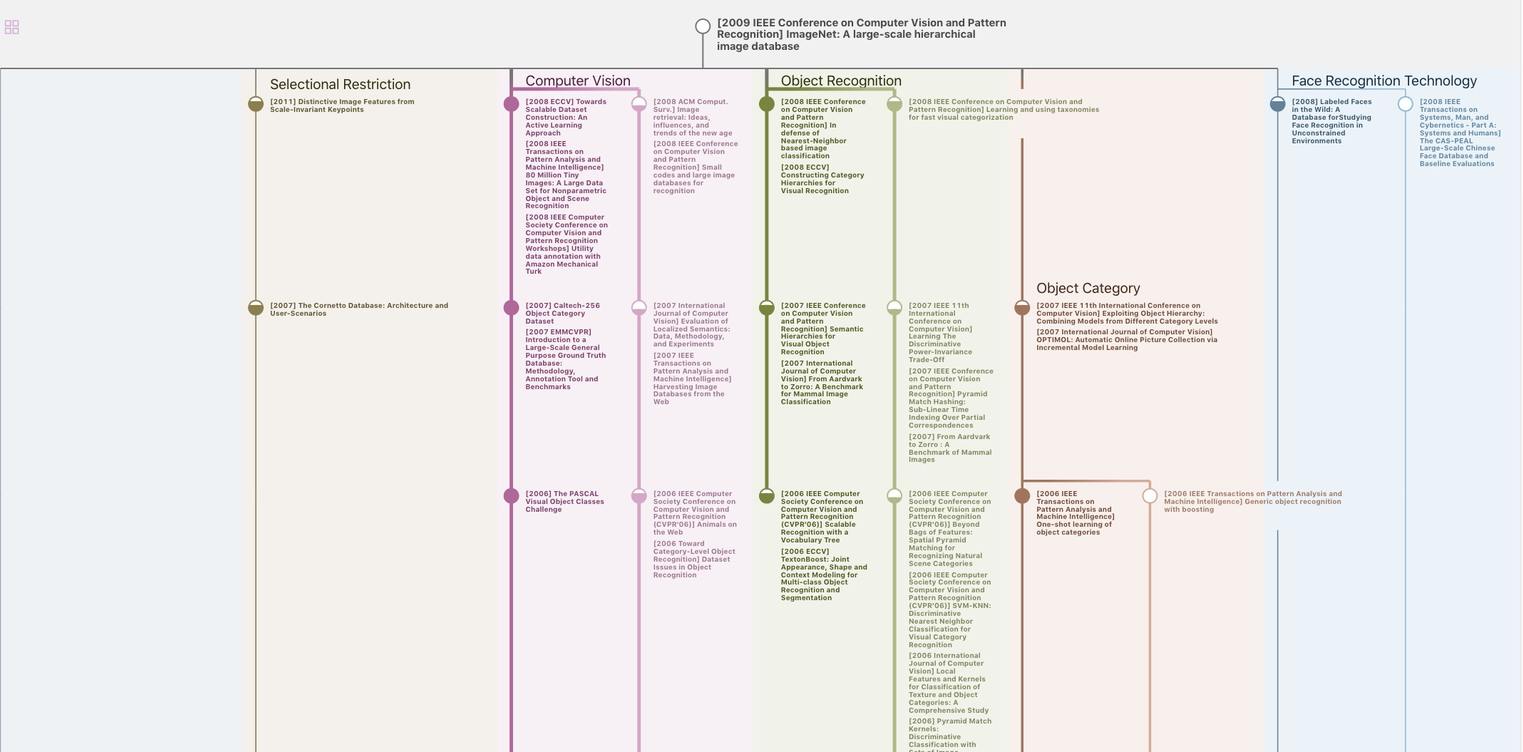
生成溯源树,研究论文发展脉络
Chat Paper
正在生成论文摘要