On the improvement of RPMNet for deep learning-based point cloud registration using a modified loss function
2023 9th International Conference on Applied System Innovation (ICASI)(2023)
摘要
Point cloud registration involves the task of finding the alignment between pairs of point clouds. Recently, deep learning-based approaches have been shown to achieve more accurate results due to the enhanced feature extraction and correspondence matching mechanisms. Most of the deep learning-based approaches estimate the registration parameters directly without explicitly estimating correspondences. Instead, the correspondences are learned implicitly while networks are trained, and the loss functions used are mostly related to errors of registration parameters. RPMNet, one of the state-of-the-art registration methods, includes an additional component in the loss function to maximize the sum of inlier probabilities in order to prevent the problem of labeling most points as outliers. In this paper, we propose to improve the RPMNet through the modification of the loss function by replacing the sum of inlier probabilities with the sum of maximal matching probabilities, with the purpose of increasing the probability of correct correspondence and thus enhancing the registration performance. Simulation results demonstrate that an order of magnitude improvement can be achieved by the proposed method.
更多查看译文
关键词
point cloud registration,deep learning,RPMNet
AI 理解论文
溯源树
样例
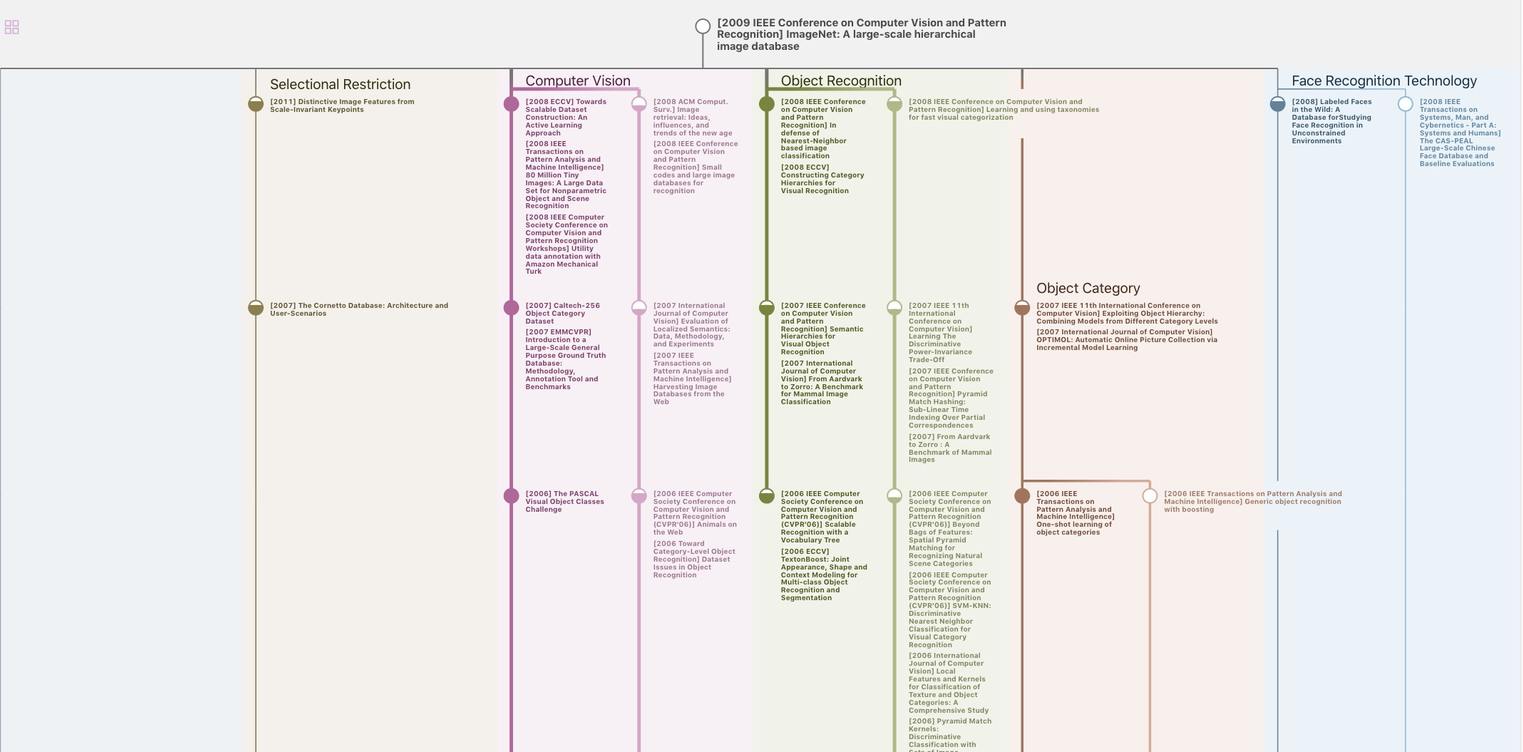
生成溯源树,研究论文发展脉络
Chat Paper
正在生成论文摘要