Enhanced Heart Failure Prediction Using Feature Selection-based Machine Learning Models
2023 Advances in Science and Engineering Technology International Conferences (ASET)(2023)
摘要
Recently, Heart Failure (HF) has been commonly known as a chronic condition and is one of the most dangerous diseases that people can acquire, therefore, several automated diagnostic methods based on Machine Learning (ML) have been developed to overcome the drawbacks of traditional heart diseases diagnosing approaches. Adopting these machine learning methods provides effective and trustworthy solutions for heart failure diagnosis. In this paper, several classification models, namely Naive Bayes (NB), Random Forest (RF), Decision Tree (DT), Radial Basis Function Network (RBFN), and Bagging, are utilized in order to provide cardiovascular disease patients with early detection of heart failure. Heart Failure Clinical Records dataset from Kaggle is utilized to enhance the accuracy of heart failure prediction. In addition, two feature selection methods are applied to select the optimal features in order to decrease computational time, speed up the classification process, and enhance the accuracy of prediction. Finally, several parameters, including accuracy, precision, recall, and F1 score, are presented to compare the performance of each classifier. The experimental results showed that by selecting the three most significant features out of 12, the performance of heart failure prediction would be enhanced with an accuracy of 81.9% using Naive Bayes model.
更多查看译文
关键词
Heart failure prediction,Feature selection,Naive Bayes,Random Forest,Decision Tree,Radial Basis Function Network,Bagging
AI 理解论文
溯源树
样例
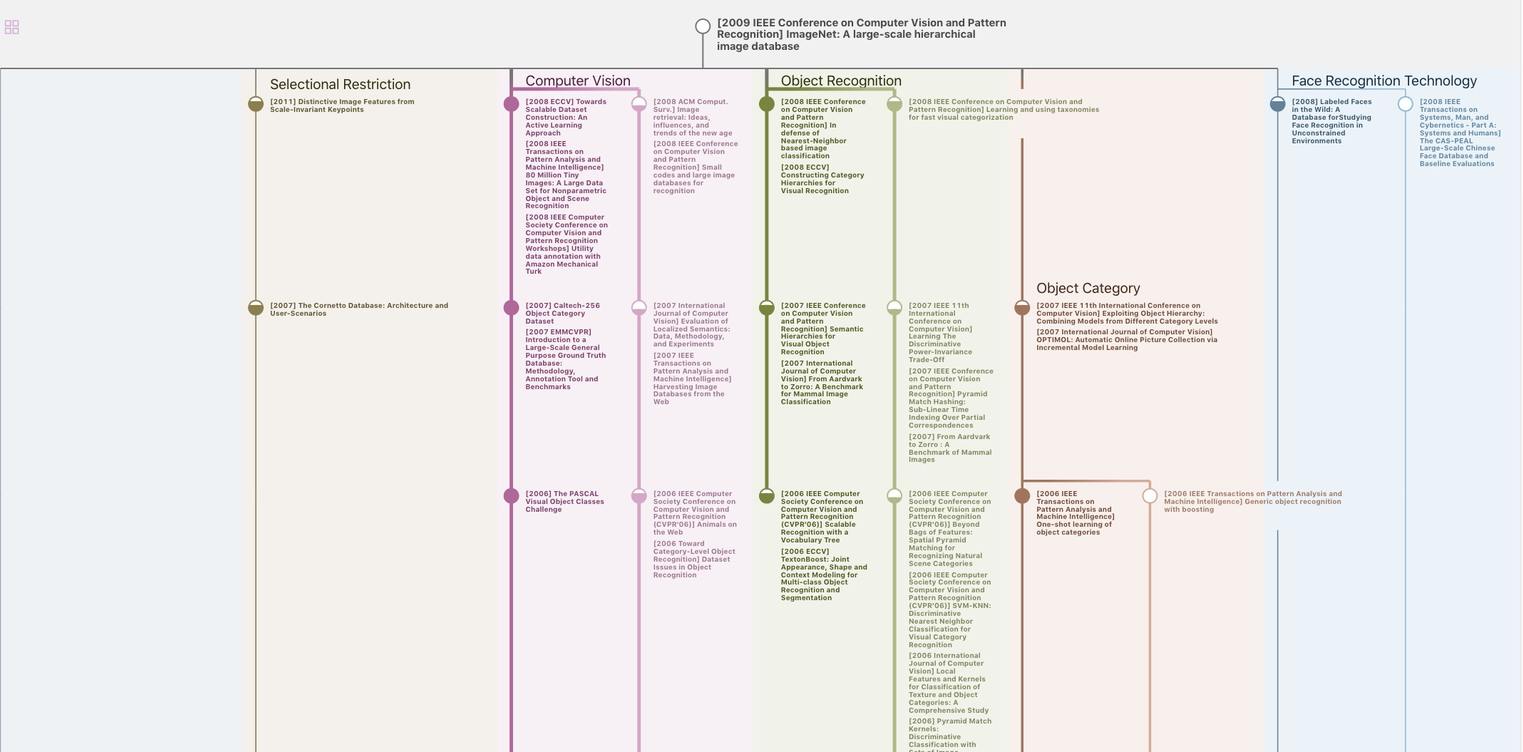
生成溯源树,研究论文发展脉络
Chat Paper
正在生成论文摘要