Spectral co-Clustering in Rank-deficient Multi-layer Stochastic co-Block Models
arXiv (Cornell University)(2023)
摘要
Modern network analysis often involves multi-layer network data in which the nodes are aligned, and the edges on each layer represent one of the multiple relations among the nodes. Current literature on multi-layer network data is mostly limited to undirected relations. However, direct relations are more common and may introduce extra information. In this paper, we study the community detection (or clustering) in multi-layer directed networks. To take into account the asymmetry, we develop a novel spectral-co-clustering-based algorithm to detect co-clusters, which capture the sending patterns and receiving patterns of nodes, respectively. Specifically, we compute the eigen-decomposition of the debiased sum of Gram matrices over the layer-wise adjacency matrices, followed by the k-means, where the sum of Gram matrices is used to avoid possible cancellation of clusters caused by direct summation. We provide theoretical analysis of the algorithm under the multi-layer stochastic co-block model, where we relax the common assumption that the cluster number is coupled with the rank of the model. After a systematic analysis of the eigen-vectors of population version algorithm, we derive the misclassification rates which show that multi-layers would bring benefit to the clustering performance. The experimental results of simulated data corroborate the theoretical predictions, and the analysis of a real-world trade network dataset provides interpretable results.
更多查看译文
关键词
co-clustering,rank-deficient,multi-layer,co-block
AI 理解论文
溯源树
样例
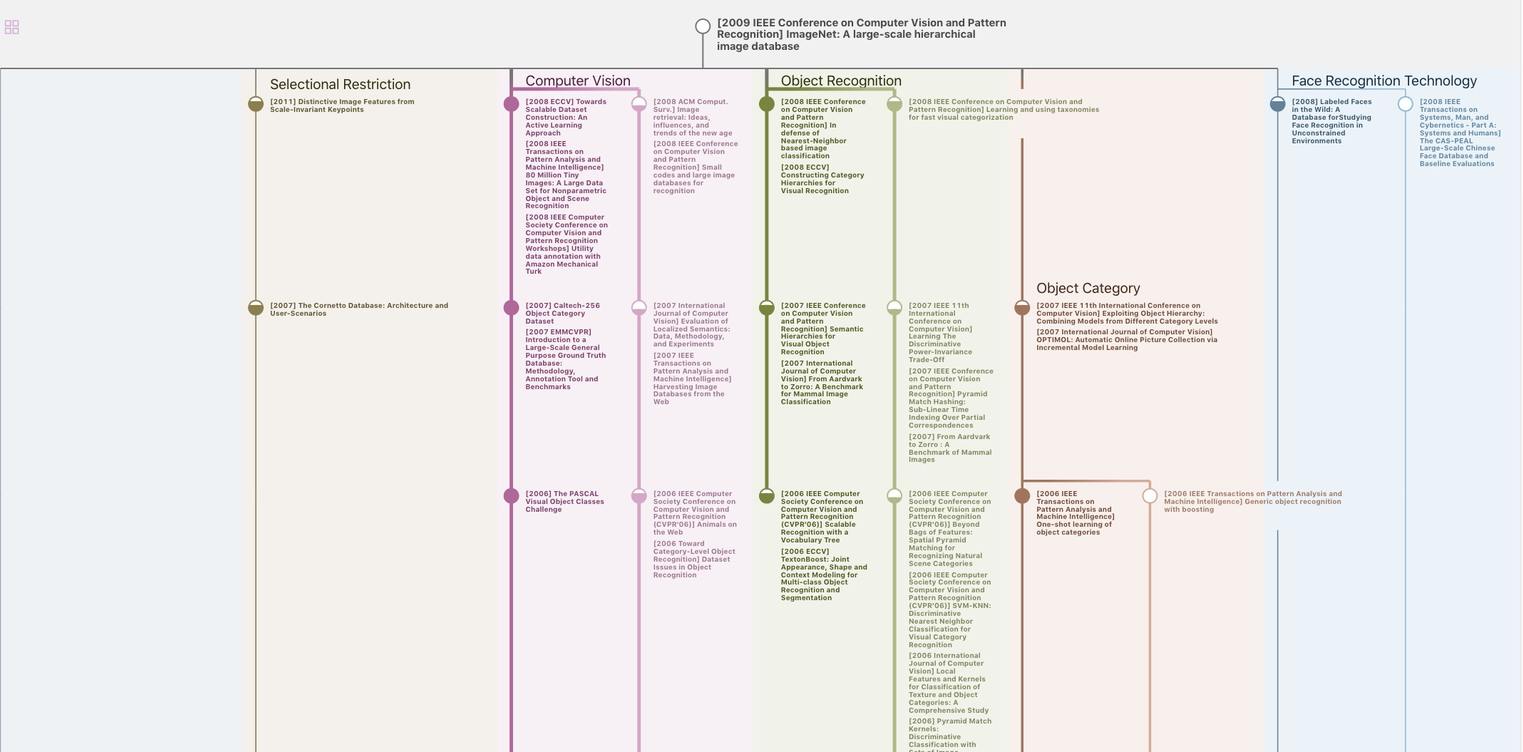
生成溯源树,研究论文发展脉络
Chat Paper
正在生成论文摘要