Quantum Descriptors for Predicting and Understanding the Structure-Activity Relationships of Michael Acceptor Warheads.
Journal of chemical information and modeling(2023)
摘要
Predictive modeling and understanding of chemical warhead reactivities have the potential to accelerate targeted covalent drug discovery. Recently, the carbanion formation free energies as well as other ground-state electronic properties from density functional theory (DFT) calculations have been proposed as predictors of glutathione reactivities of Michael acceptors; however, no clear consensus exists. By profiling the thiol-Michael reactions of a diverse set of singly- and doubly-activated olefins, including several model warheads related to afatinib, here we reexamined the question of whether low-cost electronic properties can be used as predictors of reaction barriers. The electronic properties related to the carbanion intermediate were found to be strong predictors, e.g., the change in the C charge accompanying carbanion formation. The least expensive reactant-only properties, the electrophilicity index, and the C charge also show strong rank correlations, suggesting their utility as quantum descriptors. A second objective of the work is to clarify the effect of the β-dimethylaminomethyl (DMAM) substitution, which is incorporated in the warheads of several FDA-approved covalent drugs. Our data suggest that the β-DMAM substitution is cationic at neutral pH in solution and promotes acrylamide's intrinsic reactivity by enhancing the charge accumulation at C upon carbanion formation. In contrast, the inductive effect of the β-trimethylaminomethyl substitution is diminished due to steric hindrance. Together, these results reconcile the current views of the intrinsic reactivities of acrylamides and contribute to large-scale predictive modeling and an understanding of the structure-activity relationships of Michael acceptors for rational TCI design.
更多查看译文
关键词
michael acceptor warheads,quantum,structure–activity
AI 理解论文
溯源树
样例
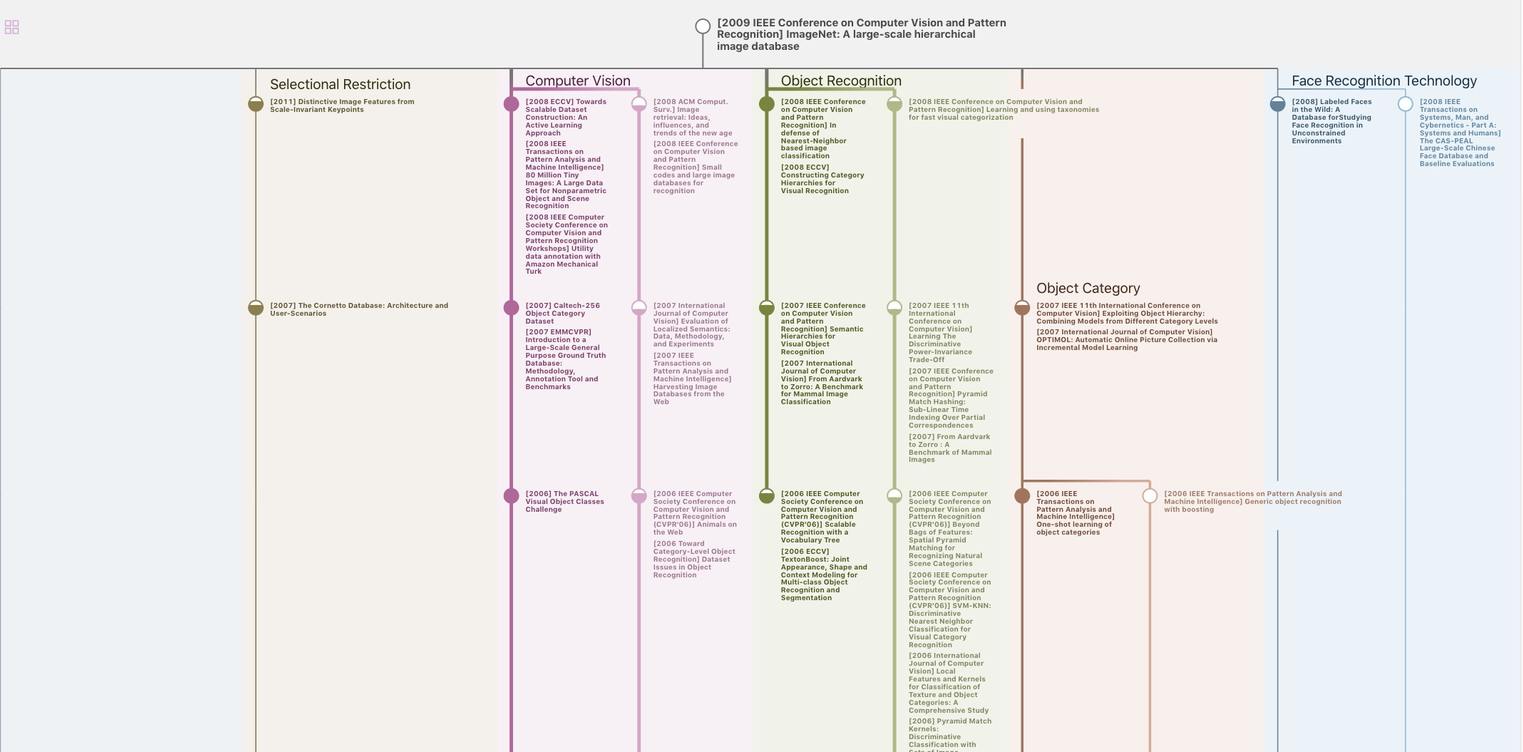
生成溯源树,研究论文发展脉络
Chat Paper
正在生成论文摘要