Noise-aware Local Model Training Mechanism for Federated Learning
ACM Transactions on Intelligent Systems and Technology(2023)
摘要
As a new paradigm in training intelligent models, federated learning is widely used to train a global model without requiring local data to be uploaded from end devices. However, there are often mislabeled samples (i.e., noisy samples) in the dataset, which will cause the model update to deviate from the correct direction during the training process, thus reducing the convergence accuracy of the global model. Existing works employ noisy label correction techniques to reduce the impact of noisy samples on model updates by correcting labels; however, such methods necessitate the use of prior knowledge and additional communication costs, which cannot be directly applied to federated learning due to data privacy concerns and limited communication resources. Therefore, this paper proposes a noise-aware local model training method that corrects the noisy labels directly at the end device under the constraints of federated learning. By constructing a label correction model, a joint optimization problem is formally defined for optimizing both the label correction model and the client-side local training model (e.g., classification model). As a solution to this optimization problem, we propose a robustness training algorithm using label correction, along with a cross-validation data sampling algorithm that updates both models simultaneously. It is verified through experiments that the mechanism can effectively improve the model convergence accuracy on noisy datasets in federated learning scenarios.
更多查看译文
关键词
Federated learning,mislabeled samples,noise-aware,meta learning,label correction
AI 理解论文
溯源树
样例
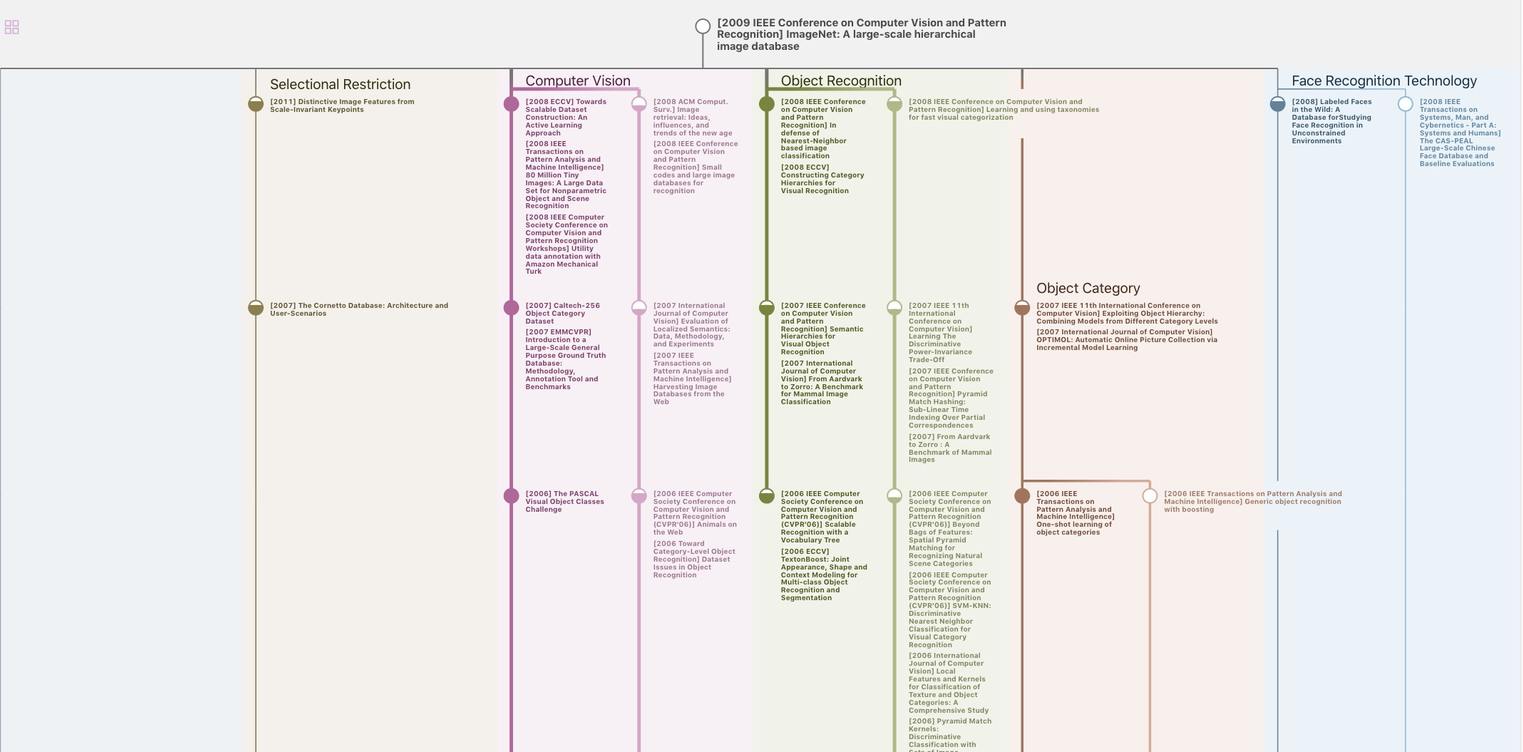
生成溯源树,研究论文发展脉络
Chat Paper
正在生成论文摘要