An event-triggering algorithm for decentralized stochastic optimization over networks
Journal of the Franklin Institute(2023)
摘要
In this paper, we study the problem of decentralized optimization to minimize a finite sum of local convex cost functions over an undirected network. Compared with the existing works, we focus on improving the communication efficiency of the stochastic gradient tracking method and propose an effective event-triggering decentralized stochastic gradient tracking algorithm, namely, ET-DSGT. ET-DSGT utilizes the event-triggering mechanism in which each agent only broadcasts its estimators at the event time to effectively avoid real-time communication, thus improving communication efficiency. In addition, we present a theoretical analysis to show that ET-DSGT with a decaying step-size can converge to the exact global minimum. Moreover, we show that for each agent, the time interval between two successive triggering times is greater than the iteration interval under certain conditions. Finally, we provide several simulations to demonstrate the effectiveness of ET-DSGT. & COPY; 2023 The Franklin Institute. Published by Elsevier Inc. All rights reserved.
更多查看译文
关键词
decentralized stochastic optimization,networks,event-triggering
AI 理解论文
溯源树
样例
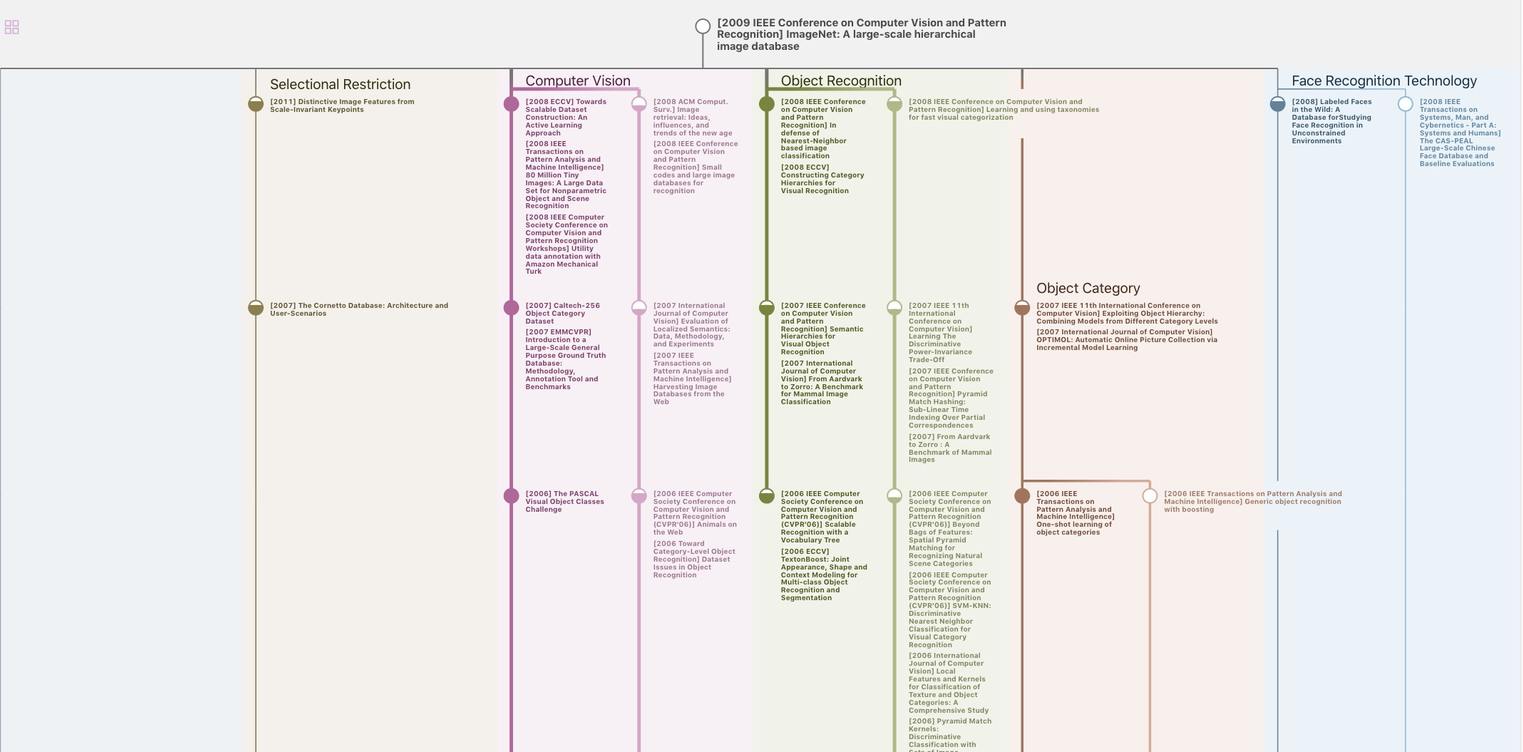
生成溯源树,研究论文发展脉络
Chat Paper
正在生成论文摘要