A hybrid CLSTM-GPR model for forecasting particulate matter (PM2.5)
Atmospheric Pollution Research(2023)
摘要
PM2.5 concentration is closely related to air pollution and human health, which should be predicted accurately and reliably. In this study, we proposed a hybrid model combining convolution neural network (CNN), long-short term memory network (LSTM), and gaussian process regression (GPR), called CLSTM-GPR model to fully extract the spatial-temporal information from the PM2.5 data series to achieve precise point prediction and dependable interval prediction. To demonstrate the model's quality and dependability, the CLSTM-GPR model was applied to PM2.5 concentration prediction at two monitoring stations, and comparisons were made with CNN-GPR, LSTM-GPR, and GPR models at the same time to evaluate the point prediction accuracy and interval prediction applicability. The CLSTM-GPR model presented the best overall prediction results with R increasing by over 4.38%, R2 increasing by over 8.96%, MAE decreasing by over 5.14%, RMSE decreasing by over 4.68%, and MC decreasing by more than 17.28% compared to other models. The results show that the CLSTM-GPR model is able to produce highly accurate point predictions and appropriate prediction intervals for PM2.5 concentration prediction. Thus, the CLSTM-GPR model has great potential for predicting PM2.5 concentrations. Also, this is the first application of the CLSTM-GPR model for PM2.5 concentration prediction. Overall, this study highlights the potential of the proposed model and demonstrates its further application in PM2.5 concentration prediction.
更多查看译文
关键词
particulate matter,clstm-gpr
AI 理解论文
溯源树
样例
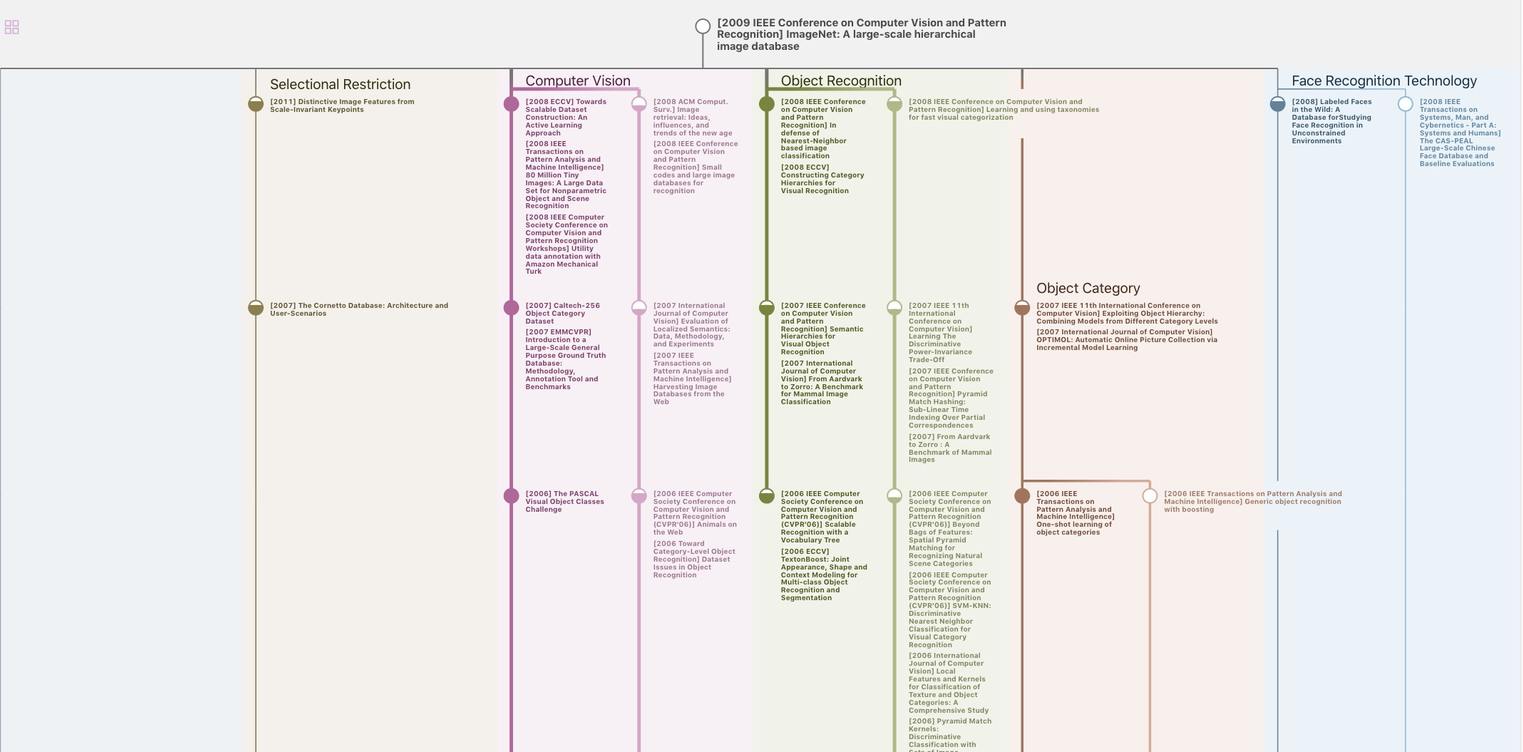
生成溯源树,研究论文发展脉络
Chat Paper
正在生成论文摘要