Concept-drift detection index based on fuzzy formal concept analysis for fake news classifiers
Technological Forecasting and Social Change(2023)
摘要
Unpredictable changes in the underlying distribution of the streaming data over time are known as concept drift. The development of procedures and techniques for drift detection, interpretation, and adaptation is central to concept-drift research. Data research has demonstrated that machine learning in a concept-drift environment produces poor learning results if drift is not handled. This study focuses on defining the concept-drift detection index to predict when the performance of a machine learning model for text-stream classifiers is low. It proposes an index that relies on the Fuzzy Formal Concept Analysis theory. The index exploits the formal lattice to understand whether new incoming facts (e.g., news) are well represented in the training data used to build the machine-learning model. Fake news was deemed ideal for testing this new measure because its typical application scenario required handling a stream of unstructured content and concept-drift awareness. Experiments on three news datasets revealed a relevant correlation (i.e., 73.9 %, 80.8 %, and 81 %) between the Accuracy of Random Forest (RF), Naïve Bayes (NB), and Passive Aggressive (PA) models, respectively, and the proposed index. This strong correlation suggests that the new index can avoid incorrect classifications and help in retraining decisions.
更多查看译文
关键词
Concept drift, Machine learning, Fuzzy formal concept analysis, Fake news, Text classification
AI 理解论文
溯源树
样例
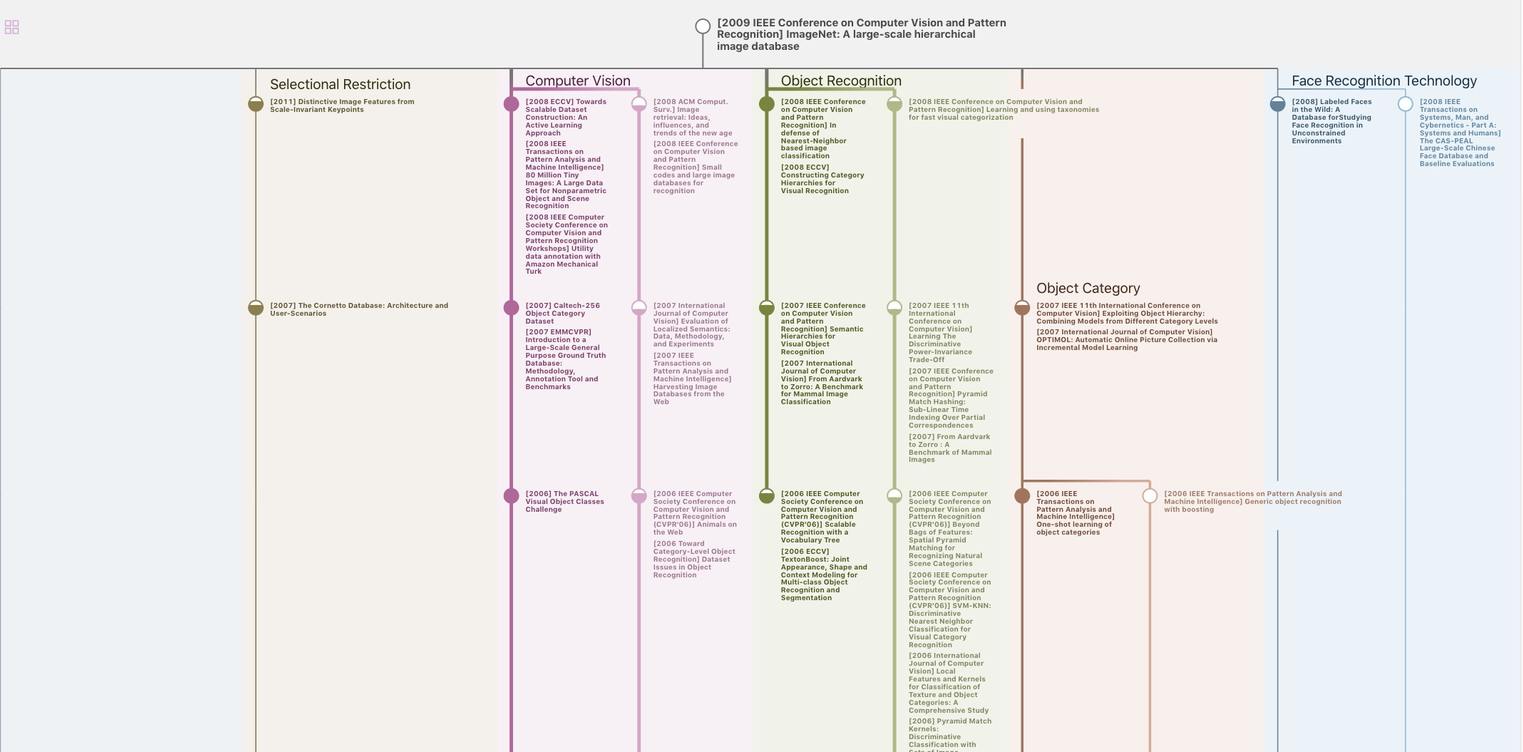
生成溯源树,研究论文发展脉络
Chat Paper
正在生成论文摘要