Building Volumetric Beliefs for Dynamic Environments Exploiting Map-Based Moving Object Segmentation
IEEE Robotics Autom. Lett.(2023)
摘要
Mobile robots that navigate in unknown environments need to be constantly aware of the dynamic objects in their surroundings for mapping, localization, and planning. It is key to reason about moving objects in the current observation and at the same time to also update the internal model of the static world to ensure safety. In this paper, we address the problem of jointly estimating moving objects in the current 3D LiDAR scan and a local map of the environment. We use sparse 4D convolutions to extract spatio-temporal features from scan and local map and segment all 3D points into moving and non-moving ones. Additionally, we propose to fuse these predictions in a probabilistic representation of the dynamic environment using a Bayes filter. This volumetric belief models, which parts of the environment can be occupied by moving objects. Our experiments show that our approach outperforms existing moving object segmentation baselines and even generalizes to different types of LiDAR sensors. We demonstrate that our volumetric belief fusion can increase the precision and recall of moving object segmentation and even retrieve previously missed moving objects in an online mapping scenario.
更多查看译文
关键词
Mapping, computer vision for transportation, intelligent transportation systems
AI 理解论文
溯源树
样例
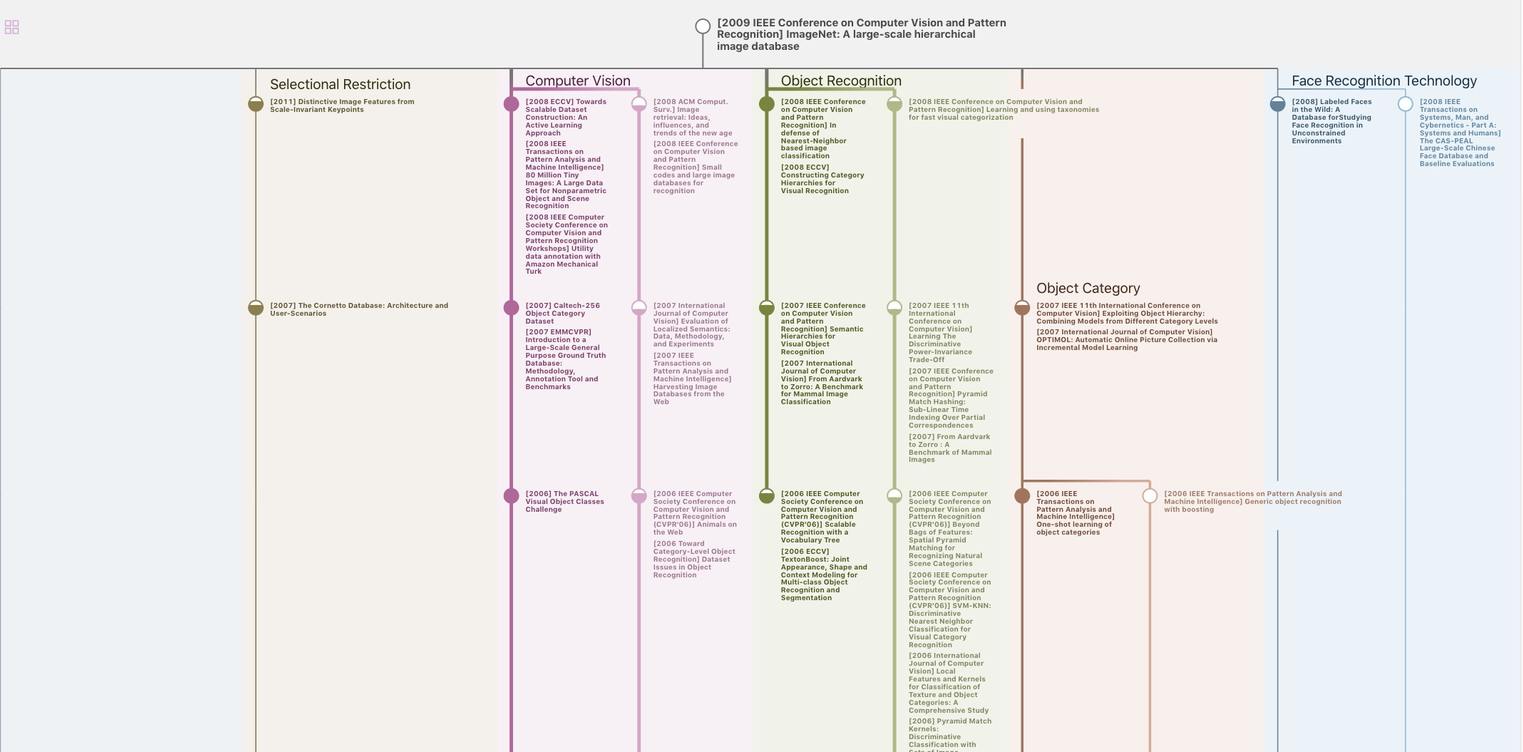
生成溯源树,研究论文发展脉络
Chat Paper
正在生成论文摘要