Research on Small Sample Multi-Target Grasping Technology Based on Transfer Learning
Sensors (Basel, Switzerland)(2023)
摘要
This article proposes a CBAM-ASPP-SqueezeNet model based on the attention mechanism and atrous spatial pyramid pooling (CBAM-ASPP) to solve the problem of robot multi-target grasping detection. Firstly, the paper establishes and expends a multi-target grasping dataset, as well as introduces and uses transfer learning to conduct network pre-training on the single-target dataset and slightly modify the model parameters using the multi-target dataset. Secondly, the SqueezeNet model is optimized and improved using the attention mechanism and atrous spatial pyramid pooling module. The paper introduces the attention mechanism network to weight the transmitted feature map in the channel and spatial dimensions. It uses a variety of parallel operations of atrous convolution with different atrous rates to increase the size of the receptive field and preserve features from different ranges. Finally, the CBAM-ASPP-SqueezeNet algorithm is verified using the self-constructed, multi-target capture dataset. When the paper introduces transfer learning, the various indicators converge after training 20 epochs. In the physical grabbing experiment conducted by Kinova and SIASUN Arm, a network grabbing success rate of 93% was achieved.
更多查看译文
关键词
grab detection, attention mechanism, SqueezeNet, multi-object detection, deep learning
AI 理解论文
溯源树
样例
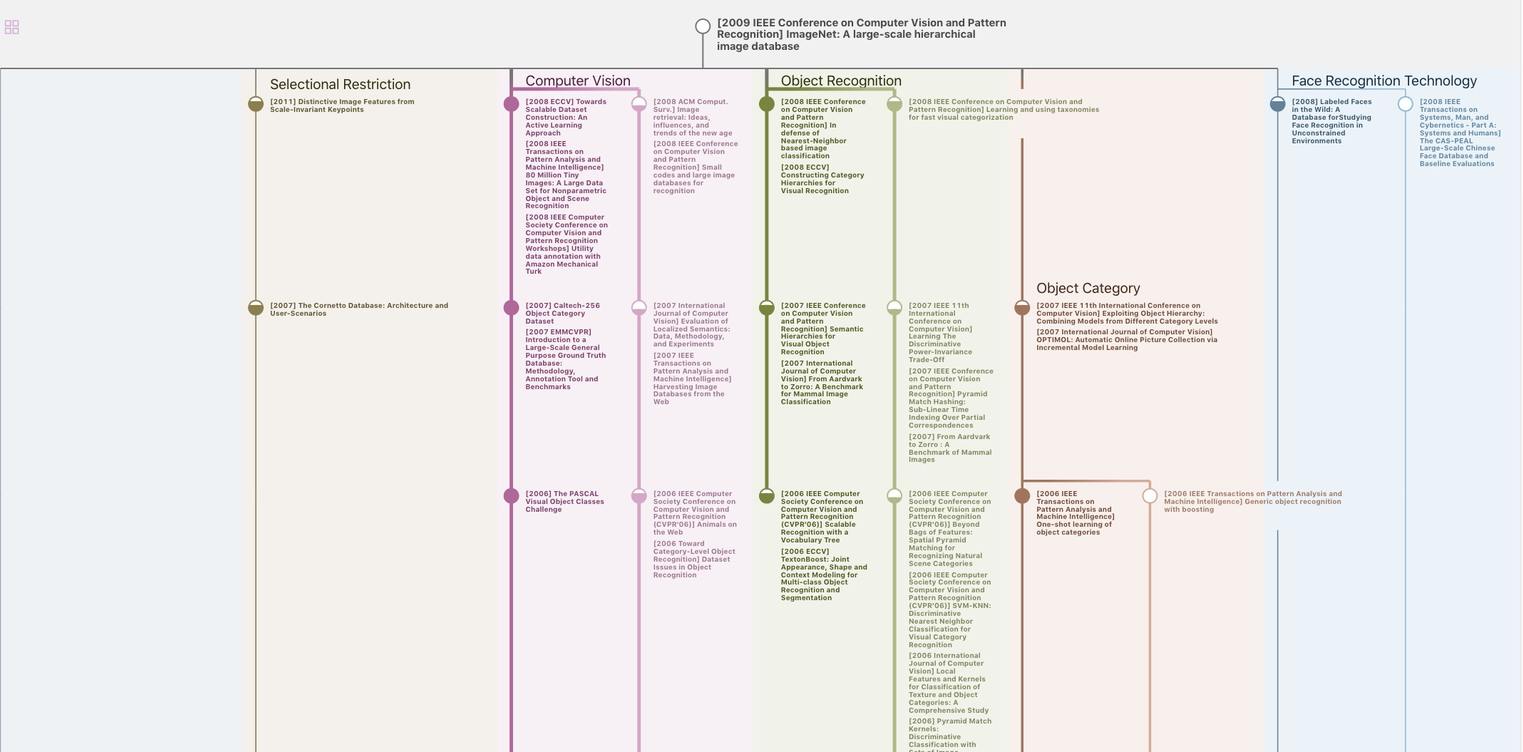
生成溯源树,研究论文发展脉络
Chat Paper
正在生成论文摘要