Theoretical Convergence Guaranteed Resource-Adaptive Federated Learning with Mixed Heterogeneity.
KDD(2023)
摘要
In this paper, we propose an adaptive learning paradigm for resource-constrained cross-device federated learning, in which heterogeneous local submodels with varying resources can be jointly trained to produce a global model. Different from existing studies, the sub-model structures of different clients are formed by arbitrarily assigned neurons according to their local resources. Along this line, we first design a general resource-adaptive federated learning algorithm, namely RA-Fed, and rigorously prove its convergence with asymptotically optimal rate O (1/root T* TQ) under loose assumptions. Furthermore, to address both submodels heterogeneity and data heterogeneity challenges under non-uniform training, we come up with a new server aggregation mechanism RAM-Fed with the same theoretically proved convergence rate. Moreover, we shed light on several key factors impacting convergence, such as minimum coverage rate, data heterogeneity level, submodel induced noises. Finally, we conduct extensive experiments on two types of tasks with three widely used datasets under different experimental settings. Compared with the state-of-the-arts, our methods improve the accuracy up to 10% on average. Particularly, when submodels jointly train with 50% parameters, RAM-Fed achieves comparable accuracy to FedAvg trained with the full model.
更多查看译文
关键词
Federated learning,Limited resources,Heterogeneity
AI 理解论文
溯源树
样例
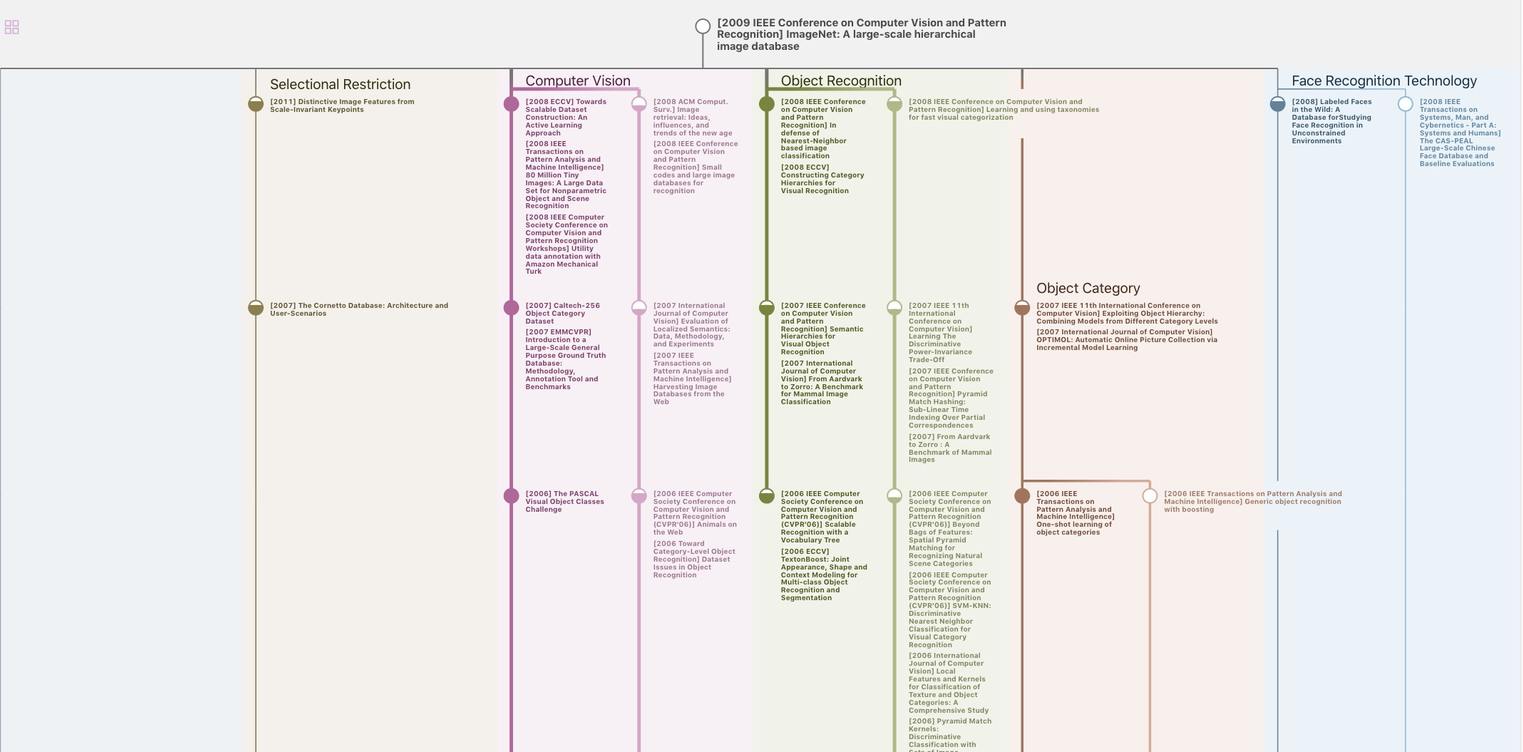
生成溯源树,研究论文发展脉络
Chat Paper
正在生成论文摘要