Towards Variance Reduction for Reinforcement Learning of Industrial Decision-making Tasks: A Bi-Critic based Demand-Constraint Decoupling Approach.
KDD(2023)
摘要
Learning to plan and schedule receives increasing attention due to its efficiency in problem-solving and potential to outperform heuristics. In particular, actor-critic-based reinforcement learning (RL) has been widely adopted for uncertain environments. Yet one standing challenge for applying RL to real-world industrial decision-making problems is the high variance during training. Existing efforts design novel value functions to alleviate the issue but still suffer. In this paper, we address this issue from the perspective of adjusting the actor-critic paradigm. We start by making an observation ignored in many industrial problems-the environmental dynamics for an agent consist of two parts physically independent of each other: the exogenous task demand over time and the hard constraint for action. And we theoretically show that decoupling these two effects in the actor-critic technique would reduce variance. Accordingly, we propose to decouple and model them separately in the state transition of the Markov decision process (MDP). In the demand-encoding process, the temporal task demand, e.g., the passengers for elevator scheduling is encoded followed by a critic for scoring. While in the constraint-encoding process, an actor-critic module is adopted for action embedding, and the two critics are then used for a revised advantaged function calculation. Experimental results show that our method can adaptively handle different dynamic planning and scheduling tasks and outperform recent learning-based models and traditional heuristic algorithms.
更多查看译文
关键词
industrial decision-making problem,reinforcement learning,variance of gradient estimates reduction
AI 理解论文
溯源树
样例
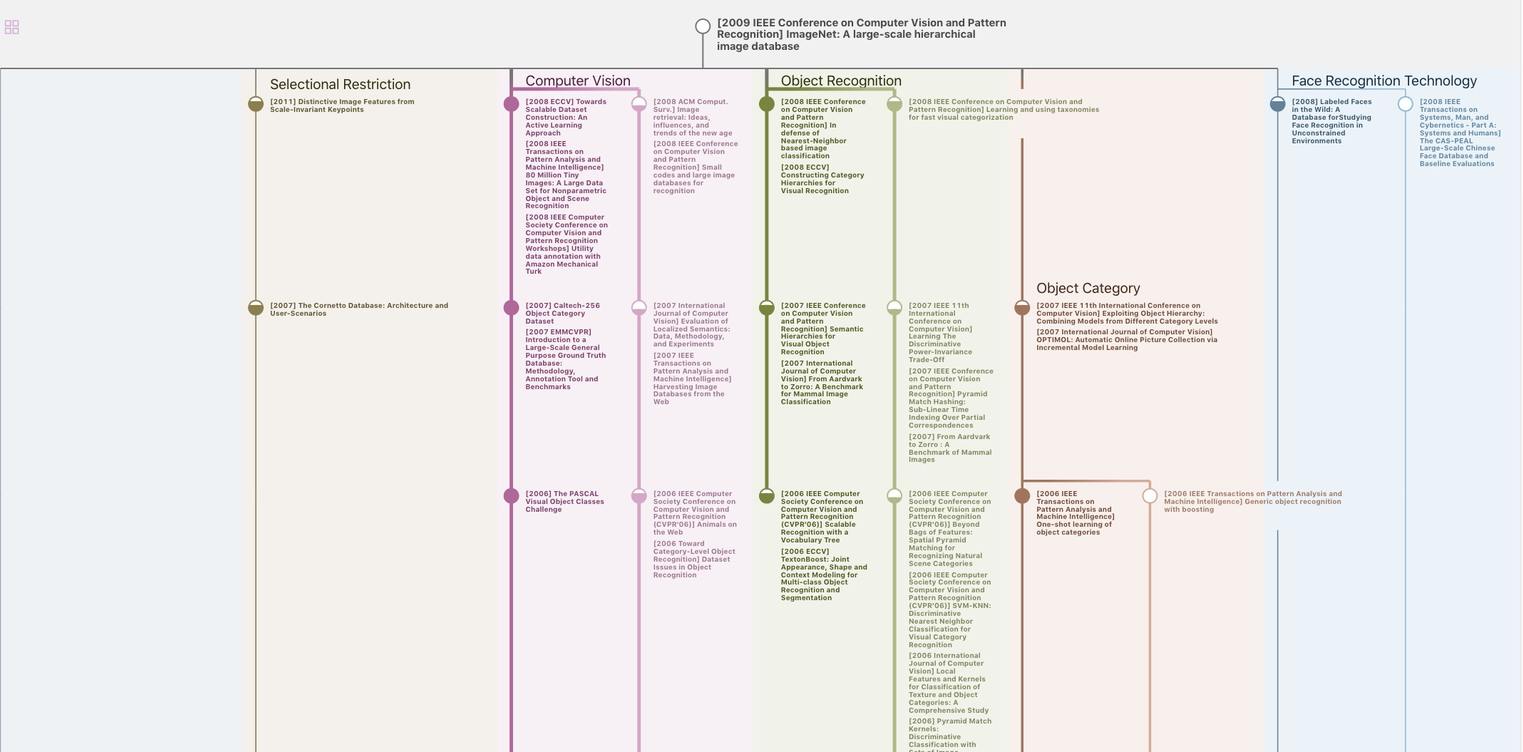
生成溯源树,研究论文发展脉络
Chat Paper
正在生成论文摘要