DeepFittingNet: A deep neural network-based approach for simplifying cardiac T1 and T2 estimation with improved robustness
Magnetic resonance in medicine(2023)
摘要
PurposeTo develop and evaluate a deep neural network (DeepFittingNet) for T-1/T-2 estimation of the most commonly used cardiovascular MR mapping sequences to simplify data processing and improve robustness. Theory and MethodsDeepFittingNet is a 1D neural network composed of a recurrent neural network (RNN) and a fully connected (FCNN) neural network, in which RNN adapts to the different number of input signals from various sequences and FCNN subsequently predicts A, B, and T-x of a three-parameter model. DeepFittingNet was trained using Bloch-equation simulations of MOLLI and saturation-recovery single-shot acquisition (SASHA) T-1 mapping sequences, and T-2-prepared balanced SSFP (T-2-prep bSSFP) T-2 mapping sequence, with reference values from the curve-fitting method. Several imaging confounders were simulated to improve robustness. The trained DeepFittingNet was tested using phantom and in-vivo signals, and compared to the curve-fitting algorithm. ResultsIn testing, DeepFittingNet performed T-1/T-2 estimation of four sequences with improved robustness in inversion-recovery T-1 estimation. The mean bias in phantom T-1 and T-2 between the curve-fitting and DeepFittingNet was smaller than 30 and 1 ms, respectively. Excellent agreements between both methods was found in the left ventricle and septum T-1/T-2 with a mean bias <6 ms. There was no significant difference in the SD of both the left ventricle and septum T-1/T-2 between the two methods. ConclusionDeepFittingNet trained with simulations of MOLLI, SASHA, and T-2-prep bSSFP performed T-1/T-2 estimation tasks for all these most used sequences. Compared with the curve-fitting algorithm, DeepFittingNet improved the robustness for inversion-recovery T-1 estimation and had comparable performance in terms of accuracy and precision.
更多查看译文
关键词
<scp>deepfittingnet</scp>
AI 理解论文
溯源树
样例
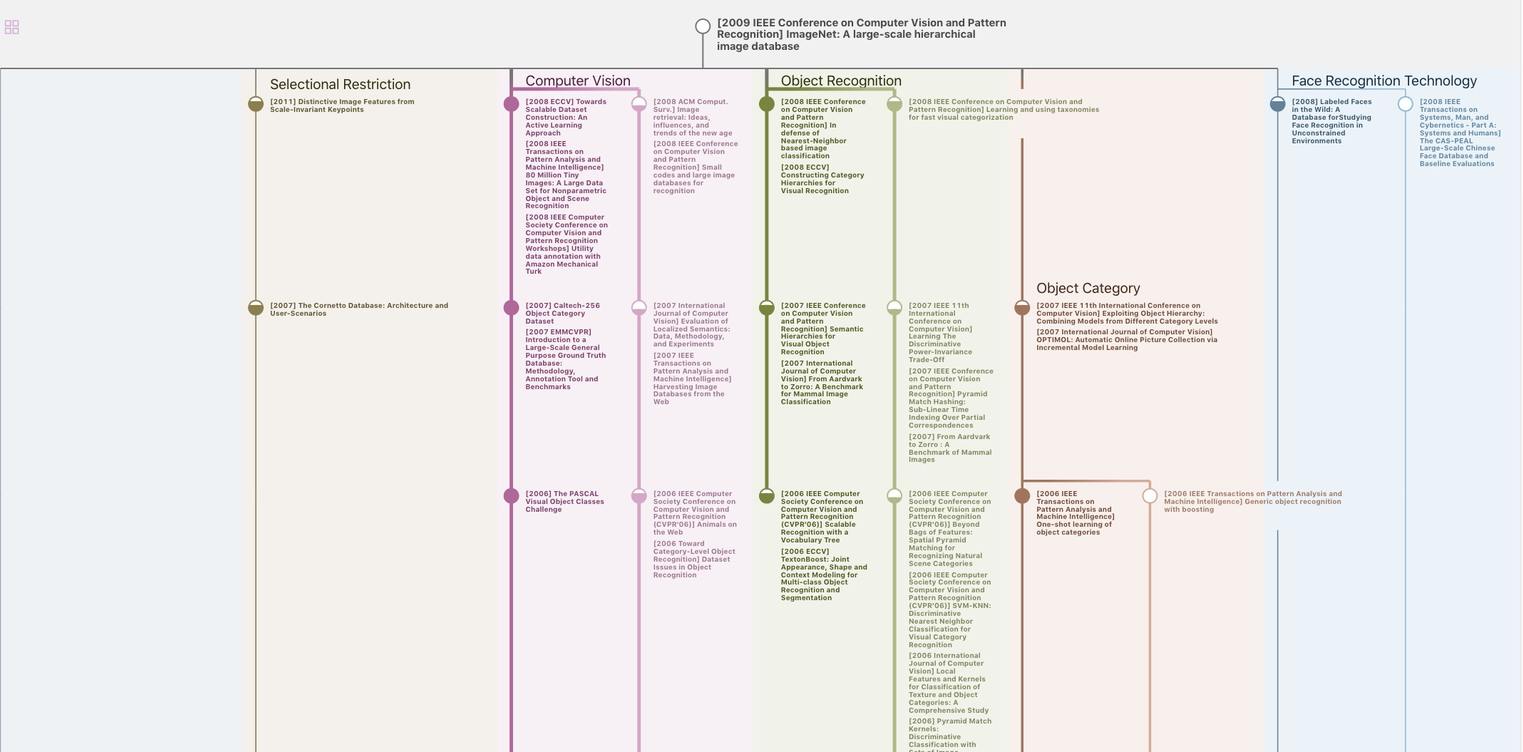
生成溯源树,研究论文发展脉络
Chat Paper
正在生成论文摘要