Cross-Lingual Universal Dependency Parsing Only From One Monolingual Treebank.
IEEE transactions on pattern analysis and machine intelligence(2023)
摘要
Syntactic parsing is a highly linguistic processing task whose parser requires training on treebanks from the expensive human annotation. As it is unlikely to obtain a treebank for every human language, in this work, we propose an effective cross-lingual UD parsing framework for transferring parser from only one source monolingual treebank to any other target languages without treebank available. To reach satisfactory parsing accuracy among quite different languages, we introduce two language modeling tasks into the training process of dependency parsing as multi-tasking. Assuming only unlabeled data from target languages plus the source treebank can be exploited together, we adopt a self-training strategy for further performance improvement in terms of our multi-task framework. Our proposed cross-lingual parsers are implemented for English, Chinese, and 29 UD treebanks. The empirical study shows that our cross-lingual parsers yield promising results for all target languages, approaching the parser performance which is trained in its own target treebank.
更多查看译文
关键词
Training, Task analysis, Data models, Syntactics, Annotations, Transfer learning, Silver, Universal dependency parsing, few-shot parsing, zero-shot parsing, cross-lingual language processing, self-training
AI 理解论文
溯源树
样例
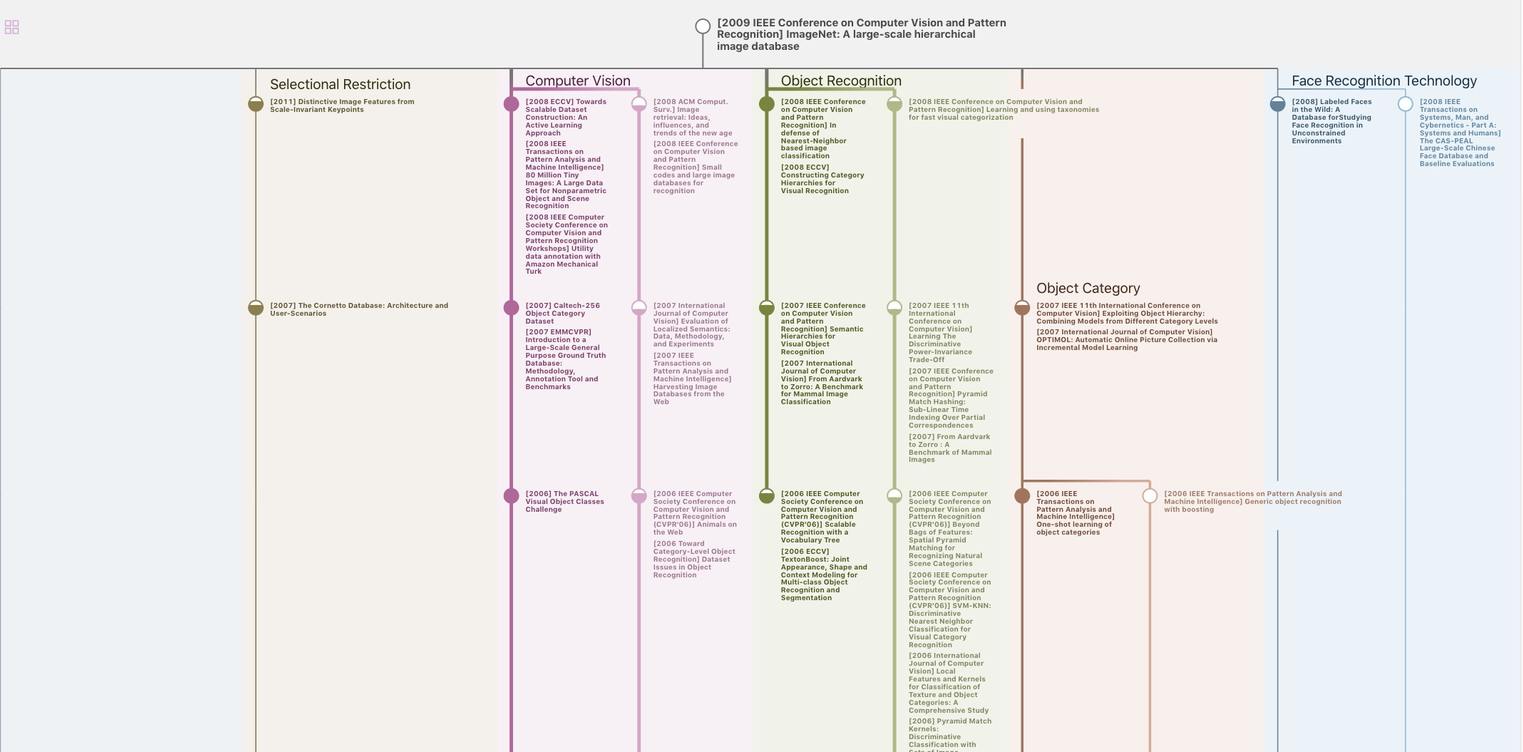
生成溯源树,研究论文发展脉络
Chat Paper
正在生成论文摘要