Facial Expression Recognition on the High Aggregation Subgraphs.
IEEE transactions on image processing : a publication of the IEEE Signal Processing Society(2023)
摘要
With the development of deep learning technology, the performance of facial expression recognition (FER) has been significantly improved. The current main challenge comes from the confusion of facial expressions caused by the highly nonlinear changes of facial expressions. However, the existing FER methods based on Convolutional Neural Networks (CNN) often ignore the underlying relationship between expressions which is crucial to meliorate the performance of recognition for confusable expressions. And the methods based on Graph Convolutional Networks (GCN) can capture the relationship between vertices, but the aggregation degree of subgraphs generated by these methods is low. They are easy to include unconfident neighbors, which increases the learning difficulty of the network. To solve the above problems, this paper proposes a method to recognize facial expressions on the high aggregation subgraphs (HASs) by combing the advantages of CNN extracting features and GCN modeling complex graph patterns. Specifically, we formulate FER as a vertex prediction problem. Considering the importance of high-order neighbors and higher efficiency, we utilize vertex confidence to find high-order neighbors. Then we construct the HASs based on the top embedding features of these high-order neighbors. And we utilize the GCN to perform reasoning and infer the class of vertices for HASs without a large number of overlapping subgraphs. Our method captures the underlying relationship between expressions on the HASs and improves the accuracy and efficiency of FER. Experimental results on both the in-the-lab datasets and the in-the-wild datasets show that our method achieves higher recognition accuracy than several state-of-the-art methods. This highlights the benefit of the underlying relationship between expressions for FER.
更多查看译文
关键词
Facial Recognition,Head,Neural Networks, Computer,Facial Expression
AI 理解论文
溯源树
样例
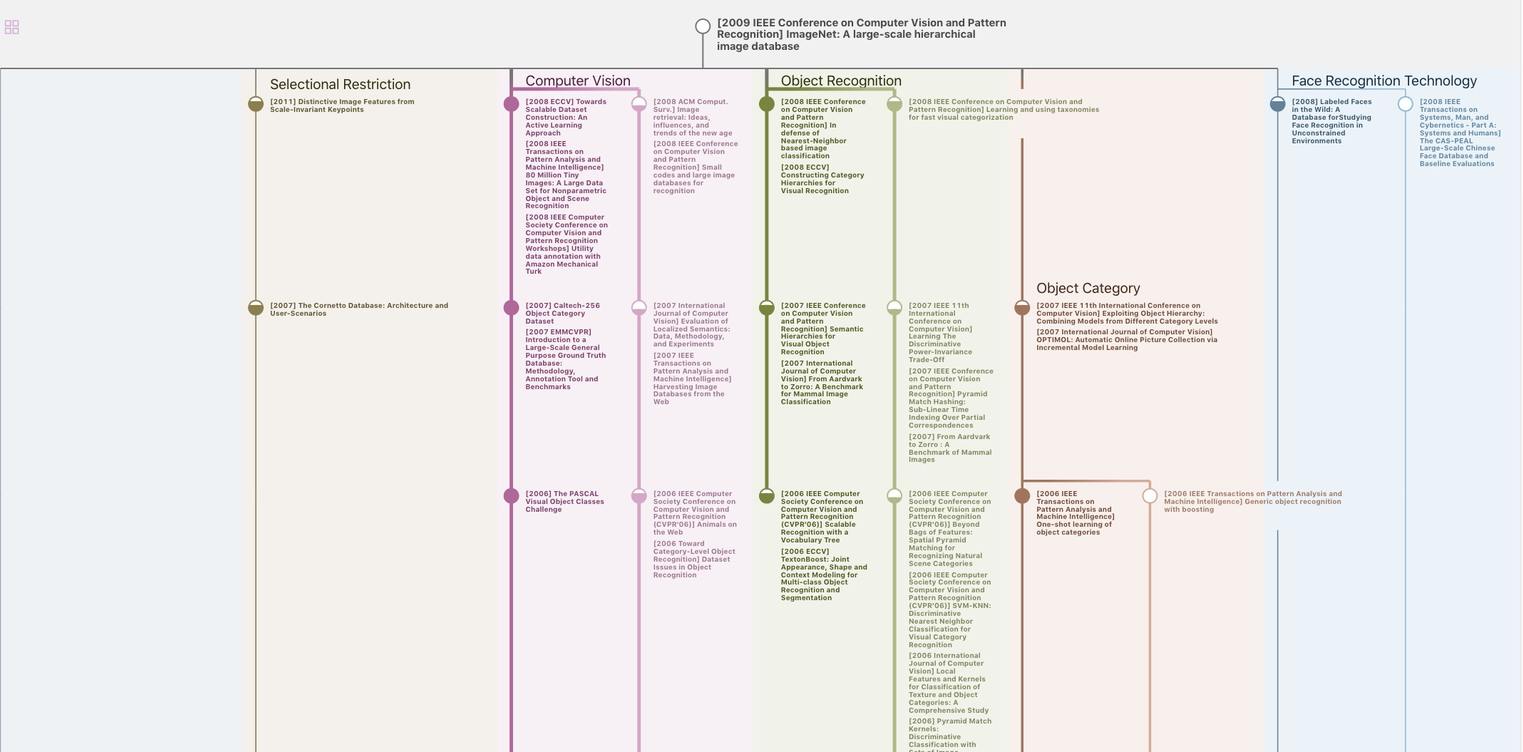
生成溯源树,研究论文发展脉络
Chat Paper
正在生成论文摘要