High-frequency monitoring of China’s green growth-at-risk
Environmental science and pollution research international(2023)
摘要
With industrialization and urbanization, China faces enormous challenges from energy security and environmental issues. To address these challenges, it is imperative to establish a green accounting system for economic growth and to measure the uncertainty of China’s green GDP (GGDP) growth from a risk management perspective. With this in mind, we follow the idea of growth-at-risk (GaR) to propose the concept of green GaR (GGaR) and extend it to the mixed-frequency data environment. Specifically, we first measure China’s annual GGDP using the System of Environmental Economic Accounting (SEEA), then construct China’s monthly green financial index by a mixed-frequency dynamic factor model (MF-DFM), and finally monitor China’s GGaR from 2008M1 to 2021M12 with the mixed data sampling-quantile regression (MIDAS-QR) method. The main findings are as follows: First, the proportion of China’s GGDP to traditional GDP gradually increases from 81.97% in 2008 to 89.34% in 2021, which illustrates that the negative environmental externalities caused by China’s economic growth are gradually decreasing. Second, the high-frequency GGaR has favorable predictive performance and is significantly superior to the common-frequency GGaR at most quantiles. Third, the high-frequency GGaR has good nowcasting performance, and its 90% and 95% confidence intervals include true value for all prediction horizons. Furthermore, it can provide early warning of economic downturns through probability density prediction. Overall, our main contribution lies in constructing a quantitative assessment and high-frequency monitoring of China’s GGDP growth risk, which provides an effective tool for investors and companies to predict risk, and a reference for the Chinese government to better formulate sustainable development strategies.
更多查看译文
关键词
Green GDP,Green finance,Growth-at-risk,MIDAS-QR,Skewed t-distribution,Nowcasting
AI 理解论文
溯源树
样例
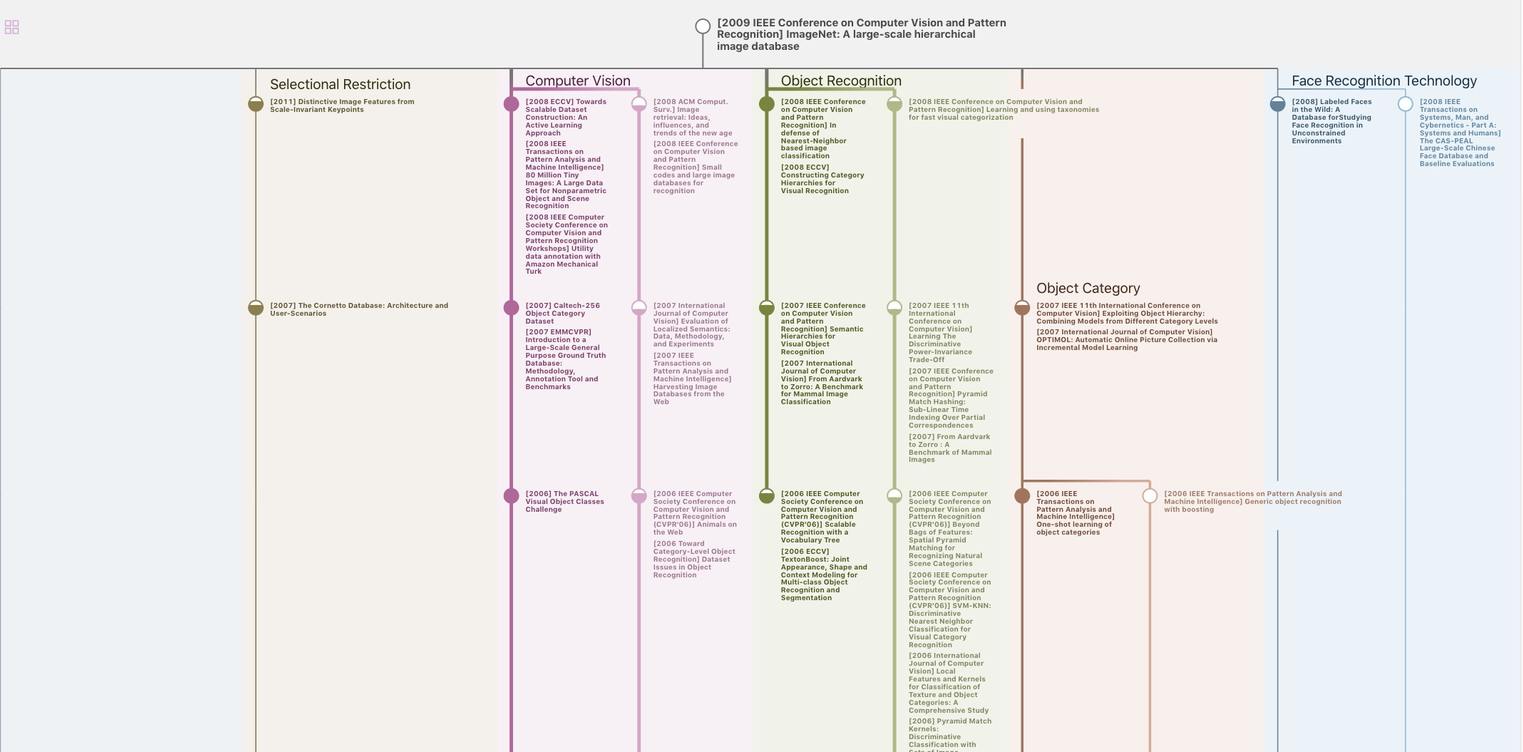
生成溯源树,研究论文发展脉络
Chat Paper
正在生成论文摘要