Clinical predictive factors and prediction models for end-stage renal disease in Chinese patients with type 2 diabetes mellitus.
Clinical and translational medicine(2023)
摘要
Dear Editor Diabetes mellitus (DM) has become a significant chronic condition that seriously affects human health.1 Nowadays, China has become the country with the largest number of DM patients worldwide, of which more than 90% are type 2 diabetes mellitus (T2DM).2 The increasing prevalence of DM exacerbates the incidence of end-stage renal disease (ESRD).3 T2DM-related ESRD not only reduces survival rate and health-related quality of life but also places a significant cost on patients as well as society.4-6 To identify clinical predictive factors and develop prediction models for ESRD risk in T2DM patients, we used the study population extracted from the China Renal Data System, a database containing the information of more than seven million patients attended at 19 hospitals in the Chinese mainland, as previously described.7 ESRD, including an eGFR of 15 mL/min/1.73 m2 or less, or the commencement of dialysis or kidney transplantation due to ESRD, was classified as the outcome. Eventually, clinical data of adult patients with T2DM were collected from 17 hospitals. Using a randomized approach, 55 824 patients with T2DM from 10 medical centers were included in the derivation cohort, and 25 745 patients from seven additional medical institutions were included for external validation. The patient selection flowchart is shown in Figure S1. After a median of 384 (123, 900) days of follow-up, there were 1,527 (2.74%) outcomes in the derivation cohort (n = 55,824). Table S1 summarizes the clinical features at baseline. Spearman correlation analysis was conducted to identify the correlation between continuous variables (Figure S2), and variables with higher average correlation (correlation ≥ 0.5) were removed. Univariate Cox regression analysis was used to select potential predictors (p < 0.1), as demonstrated in Table S2. All potential predictors were, therefore, fitted into a multivariable Cox regression model, utilizing step-wise backward selection (p < 0.05). Ten clinical predictive factors, including age, hypertension, diabetes retinopathy (DR), hemoglobin (HGB), serum albumin (ALB), serum creatinine (Scr), serum uric acid, Low-density lipoprotein cholesterol (LDL-C), serum fibrinogen, and urinary protein were selected into the final model (Table S3). We constructed three clinical prediction models using various combinations of predictors selected by multivariable Cox regression (Table 1). Model 1 (full model), including all of the 10 clinical predictive factors, achieved the highest discrimination (area under the curve [AUC]: 0.926, 95% confidence interval [CI]: 0.919–0.934); model 2 (laboratory model), including all the laboratory indicators, with an AUC of 0.924 (95% CI: 0.917–0.932); model 3 (simplified model) incorporated five easily accessible predictors, including age, hypertension, HGB, Scr, and urinary protein, with an AUC of 0.916 (95% CI: 0.908–0.924) (Figure 1A). In addition, these models attained satisfactory calibration, as shown in Figure S3A-C. Internal validation using bootstrapping also achieved a robust discrimination, with an AUC of 0.914–0.927, as shown in Table 1. 0.926 (0.919, 0.934) 0.924 (0.917, 0.932) .916 (0.908, 0.924) 0.927 (0.920, 0.935) 0.925 (0.917, 0.932) 0.914 (0.906, 0.922) 0.882 (0.871, 0.894) 0.877 (0.866, 0.889) 0.868 (0.856, 0.881) In the external validation cohort (n = 25,745), during a median follow-up of 321 (90, 758) days, there were 1,084 (4.21%) outcomes. Table S4 presents the baseline clinical features. Based on the receiver-operating characteristic (ROC) curves, the prediction models achieved an AUC ranging from 0.868 to 0.882 (Figure 1B). As seen in Figure S3D-F, these models also attained satisfactory calibration. Also, we illustrated model 3 (simplified model) as a nomogram (Figure 1C). Additionally, we determined the appropriate cut-off values for the seven continuous variables included in model 1 (full model) using a minimum p-value method (Table S5). We then employed restricted cubic spline (RCS) to describe the relationship between these clinical predictors and outcomes, as illustrated in Figure 1D–J. Based on the findings of RCS curves and the minimum p-value method, as well as the clinical application practicality, the seven continuous variables in model 1 were categorized into different categories. Then, multivariate cox regression was conducted, and a score was awarded to each variable based on the hazard ratio (HR) presented in Table S6. Finally, a risk score was developed as follows: age (year; ≥ 56 scores 0 and < 56 scores 1), hypertension (yes scores 2 and no scores 0), DR (yes scores 1 and no scores 0), HGB (g/L; ≥ 108 scores 0 and < 108 scores 2), serum ALB (g/L; ≥ 33 scores 0 and < 33 scores 2), Scr (μmol/L; < 115 scores 0 and ≥ 115 scores 5), serum uric acid (μmol/L; < 435 scores 0 and ≥ 435 scores 1), LDL-C (mmol/L; < 4 scores 0 and ≥ 4 scores 1), serum fibrinogen (g/L; < 4 scores 0 and ≥ 4 scores 1) and urinary protein (0 – ± scores 0, 1+−2+ scores 4 and 3+−4+ scores 8), as shown in Table 2. Furthermore, we categorized the patients with T2DM into four risk groups according to the RCS curve (Figure 2A), including the low-risk (total points < 8), moderate-risk (8 ≤ total points < 15), high-risk (15 ≤ total points < 20) and very high-risk group (20 ≤ total points < 24), as shown in Figure 2B. As depicted by Kaplan–Meier survival curves (Figure 2C,D), in the derivation cohort, the HRs of developing ESRD in the moderate-, high-, and very high-risk groups were 18.64 (95% CI: 15.97–21.76), 80.62 (95% CI: 69.91--94.33) and 178.16 (95% CI: 148.66--213.52) in comparison with the low-risk group (p < 0.001); for patients in the validation cohort, the HRs in the moderate-, high-, and very high-risk groups were 10.95 (95% CI: 9.347–12.82), 44.18 (95% CI: 37.51–52.05) and 89.59 (95% CI: 72.47–110.74) in comparison with the low-risk group (p < 0.001). In conclusion, using a large multi-center retrospective cohort in the Chinese mainland, we identified 10 clinical predictive factors and developed models to predict ESRD in T2DM patients, which showed excellent prediction performance. To the best of our knowledge, we have established models to predict ESRD based on the largest population of T2DM patients in the Chinese Mainland. These prediction models were further provided as simple bedside tools, including a risk score and a nomogram, which could be extensively applied to assess T2DM patients’ ESRD risk in clinical practice, to aid clinical decision-making and sensible resource allocation. The authors wish to thank the clinicians and healthcare professionals at the participating centers in the CRDS Study. Yongxiang Gao and the team of Digital Health China Technologies Co., LTD deserve special gratitude for their assistance with data extraction. This study was supported by the National Key Research and Development Program of China to Prof. Bicheng Liu as PI (grant number: 2018YFC1314000). The authors declare no conflict of interest. The data that support the findings of this study are accessible from the corresponding author upon request. The data are not publicly available owing to ethical or privacy concerns. Please note: The publisher is not responsible for the content or functionality of any supporting information supplied by the authors. Any queries (other than missing content) should be directed to the corresponding author for the article.
更多查看译文
AI 理解论文
溯源树
样例
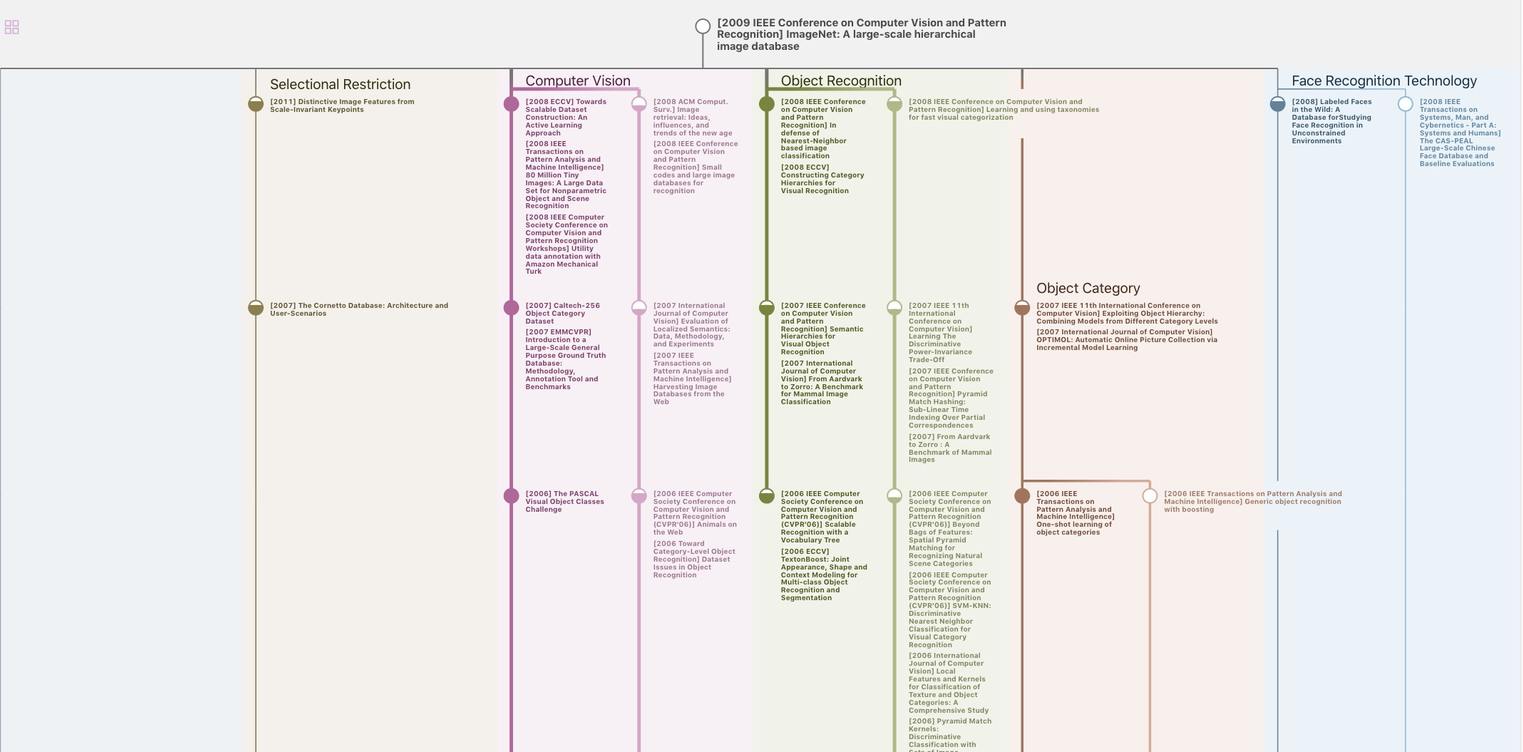
生成溯源树,研究论文发展脉络
Chat Paper
正在生成论文摘要