The usefulness of machine learning analysis for predicting the presence of depression with the results of the Korea National Health and Nutrition Examination Survey.
Annals of palliative medicine(2023)
摘要
BACKGROUND:Depression is a major public health concern, with an estimated 10.8% of adults experiencing depression. Depression can have a significant impact on an individual's quality of life, social function, and productivity. Early diagnosis of depression is important in preventing its progression. Several tools, such as the Patient Health Questionnaire-9 (PHQ-9) and Beck Depression Inventory, are used to screen patients for depression. We investigated the potential of machine learning in predicting the presence of depression using the results of a national survey.
METHODS:We collected the data of 5,420 patients from the 2020 Korea National Health and Nutrition Examination. The presence of depression was defined as ≥5 PHQ-9. We categorized output variables into the presence of depression (PHQ-9, ≥5) and absence of depression (PHQ-9, <5). We used 20 variables related to sociodemographic characteristics, health behavior, and presence of chronic disease for the development of three machine learning algorithms [random forest, logistic regression, and deep neural network (DNN)]. Eighty-seven decision trees were used for the random forest model. Linear regression algorithm shows a linear relationship between various input and output variables. For the DNN model, three layers with 16-32-64 neurons, Adam optimizer, and rectified linear unit (ReLU) activation were used. Of the included samples, 70% and 30% were randomly divided into the training and test sets, respectively.
RESULTS:The area under the curve (AUC) of the test dataset for the random forest model was 0.803 [95% confidence interval (CI), 0.776-0.829], 0.812 (95% CI, 0.787-0.837) for the logistic regression model, and 0.805 (95% CI, 0.780-0.831) for the DNN model.
CONCLUSIONS:Our study demonstrated the potential of machine learning for the development of models for predicting the presence of depression based of various health-related data. Machine learning models can potentially overcome the limitations of traditional diagnostic methods for depression by incorporating a wide range of objective variables to accurately identify patients with depression, thus avoiding the subjectivity and potential diagnostic errors associated with the subjective interpretation of symptoms observed by a clinician. Further efforts to increase the accuracy of machine learning models by utilizing more variables and data needed to detect depression.
更多查看译文
关键词
depression,korea national health,machine learning
AI 理解论文
溯源树
样例
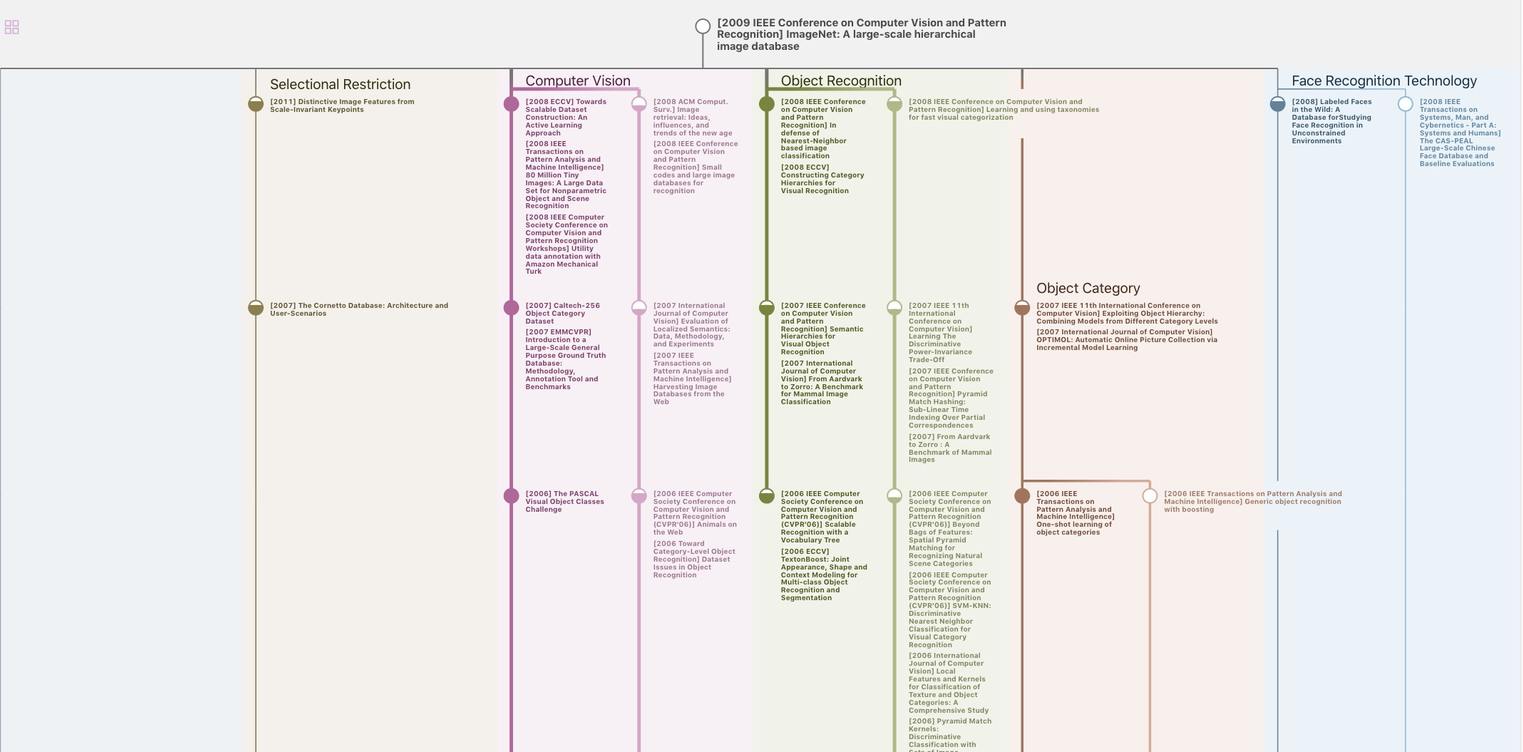
生成溯源树,研究论文发展脉络
Chat Paper
正在生成论文摘要