Unsupervised registration for liver CT-MR images based on the multiscale integrated spatial-weight module and dual similarity guidance.
Computerized medical imaging and graphics : the official journal of the Computerized Medical Imaging Society(2023)
摘要
PURPOSE:Multimodal registration is a key task in medical image analysis. Due to the large differences of multimodal images in intensity scale and texture pattern, it is a great challenge to design distinctive similarity metrics to guide deep learning-based multimodal image registration. Besides, since the limitation of the small receptive field, existing deep learning-based methods are mainly suitable for small deformation, but helpless for large deformation. To address the above issues, we present an unsupervised multimodal image registration method based on the multiscale integrated spatial-weight module and dual similarity guidance.
METHODS:In this method, a U-shape network with our multiscale integrated spatial-weight module is embedded into a multi-resolution image registration architecture to achieve end-to-end large deformation registration, where the spatial-weight module can effectively highlight the regions with large deformation and aggregate discriminative features, and the multi-resolution architecture further helps to solve the optimization problem of the network in a coarse-to-fine pattern. Furthermore, we introduce a special loss function based on dual similarity, which represents both global gray-scale similarity and local feature similarity, to optimize the unsupervised multimodal registration network.
RESULTS:We verified the effectiveness of the proposed method on liver CT-MR images. Experimental results indicate that the proposed method achieves the optimal DSC value and TRE value of 92.70 ± 1.75(%) and 6.52 ± 2.94(mm), compared with other state-of-the-art registration algorithms.
CONCLUSION:The proposed method can accurately estimate the large deformation field by aggregating multiscale features, and achieve higher registration accuracy and fast registration speed. Comparative experiments also demonstrate the effectiveness and generalization ability of the algorithm.
更多查看译文
AI 理解论文
溯源树
样例
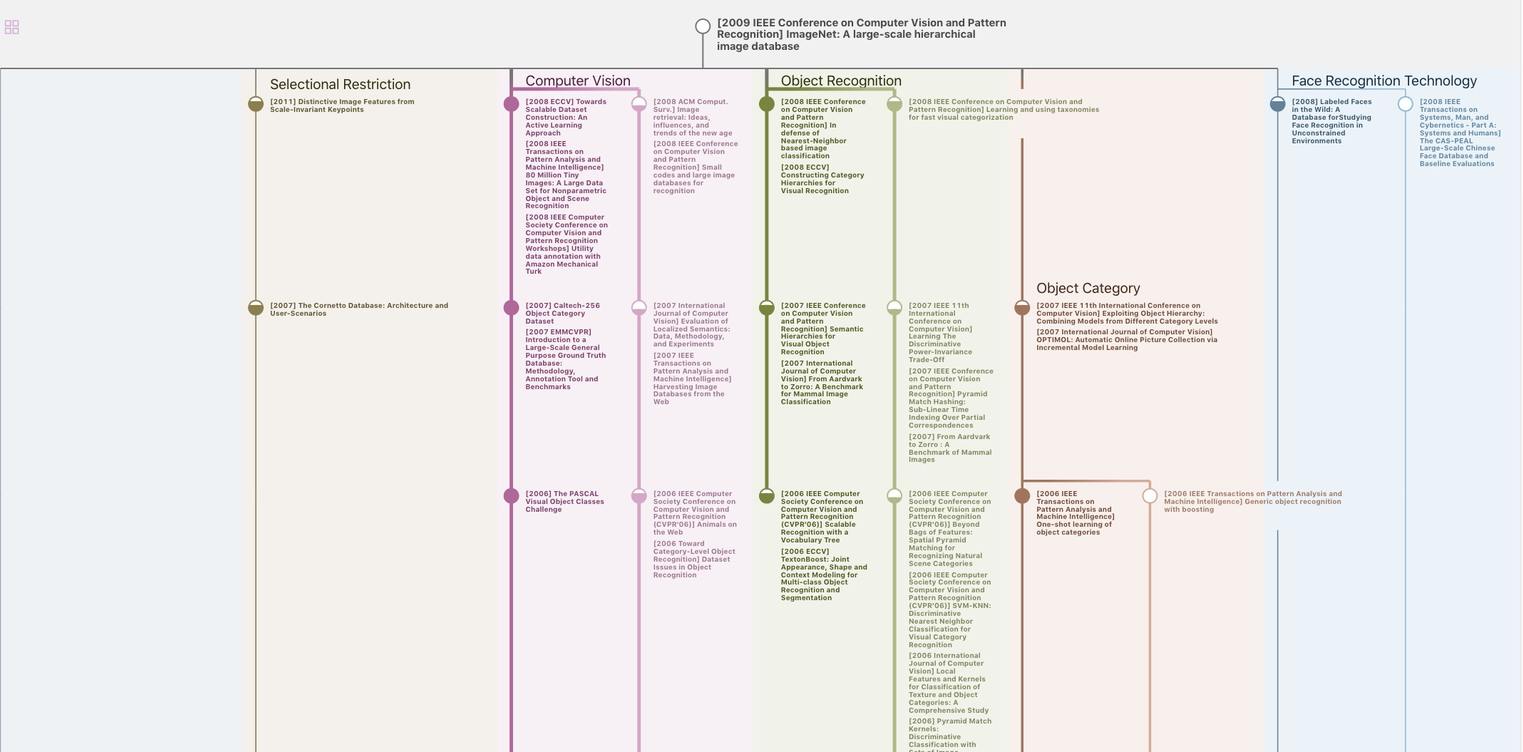
生成溯源树,研究论文发展脉络
Chat Paper
正在生成论文摘要