BRCA1-specific machine learning model predicts variant pathogenicity with high accuracy.
Physiological genomics(2023)
摘要
Identification of novel variants outpace their clinical annotation, which highlights the importance of developing accurate computational methods for risk assessment. Therefore, our aim was to develop a -specific machine learning model to predict pathogenicity of all types of variants and to apply this model and our previous specific model to assess variants of uncertain significance (VUS) among Qatari patients with breast cancer. We developed an XGBoost model that utilizes variant information such as position, frequency, consequence, as well as prediction scores from numerous in silico tools. We trained and tested the model with variants that were reviewed and classified by the Evidence-Based Network for the Interpretation of Germline Mutant Alleles (ENIGMA) consortium. Additionally, we tested the model's performance on an independent set of missense variants of uncertain significance with experimentally determined functional scores. The model performed excellently in predicting the pathogenicity of ENIGMA classified variants (accuracy 99.9%) and in predicting the functional consequence of the independent set of missense variants (accuracy 93.4%). Moreover, it predicted 2,115 potentially pathogenic variants among the 31,058 unreviewed variants in the exchange database. Using two BRCA-specific models, we did not identify any pathogenic variants among those found in patients in Qatar, but predicted four potentially pathogenic variants, which could be prioritized for functional validation.
更多查看译文
关键词
BRCA2, breast cancer, in silico predictions, ovarian cancer, VUS
AI 理解论文
溯源树
样例
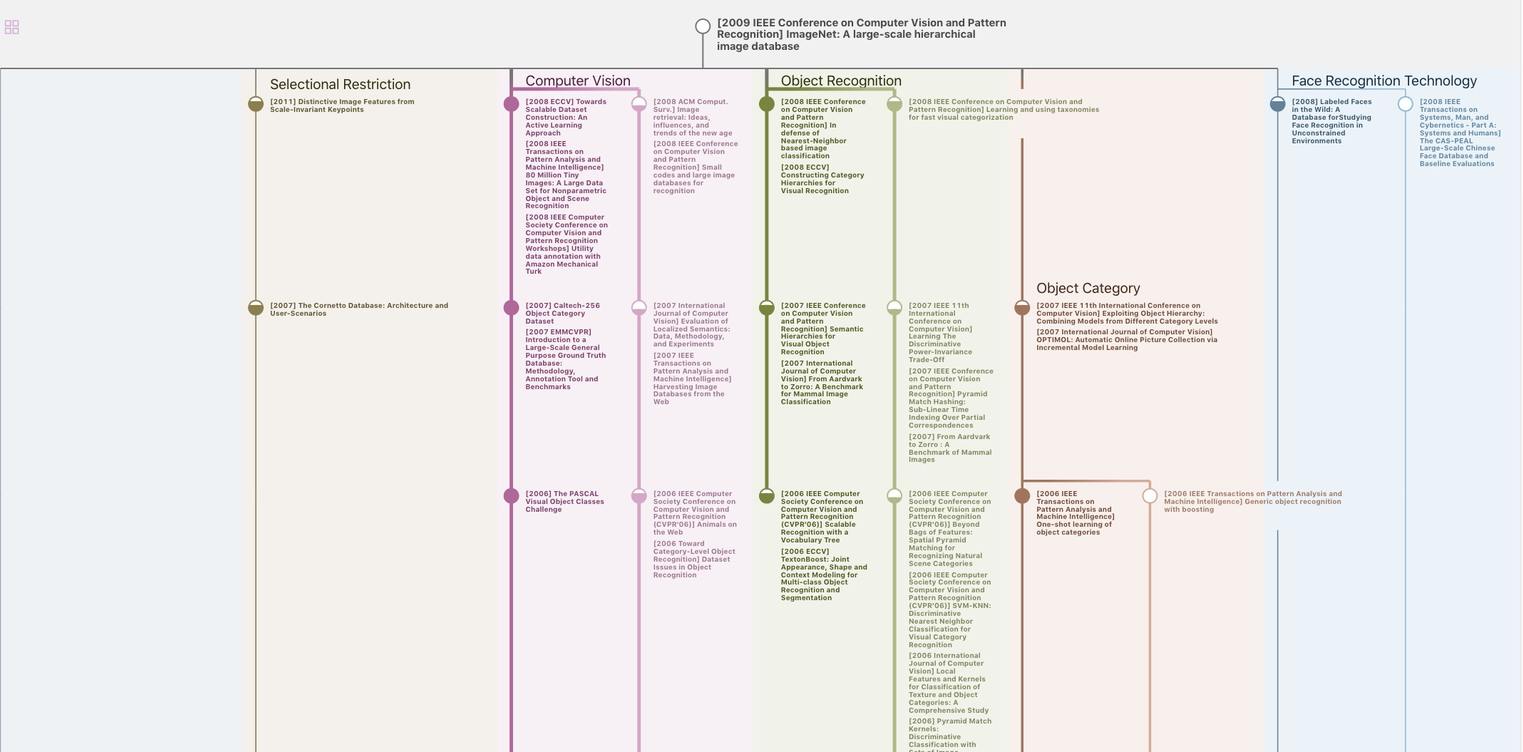
生成溯源树,研究论文发展脉络
Chat Paper
正在生成论文摘要