Adversarial feature hybrid framework for steganography with shifted window local loss.
Neural networks : the official journal of the International Neural Network Society(2023)
摘要
Image steganography is a long-standing image security problem that aims at hiding information in cover images. In recent years, the application of deep learning to steganography has the tendency to outperform traditional methods. However, the vigorous development of CNN-based steganalyzers still have a serious threat to steganography methods. To address this gap, we present an end-to-end adversarial steganography framework based on CNN and Transformer learned by shifted window local loss, called StegoFormer, which contains Encoder, Decoder, and Discriminator. Encoder is a hybrid model based on U-shaped network and Transformer block, which effectively integrates high-resolution spatial features and global self-attention features. In particular, Shuffle Linear layer is suggested, which can enhance the linear layer's competence to extract local features. Given the substantial error in the central patch of the stego image, we propose shifted window local loss learning to assist Encoder in generating accurate stego images via weighted local loss. Furthermore, Gaussian mask augmentation method is designed to augment data for Discriminator, which helps to improve the security of Encoder through adversarial training. Controlled experiments show that StegoFormer is superior to the existing advanced steganography methods in terms of anti-steganalysis ability, steganography effectiveness, and information restoration.
更多查看译文
AI 理解论文
溯源树
样例
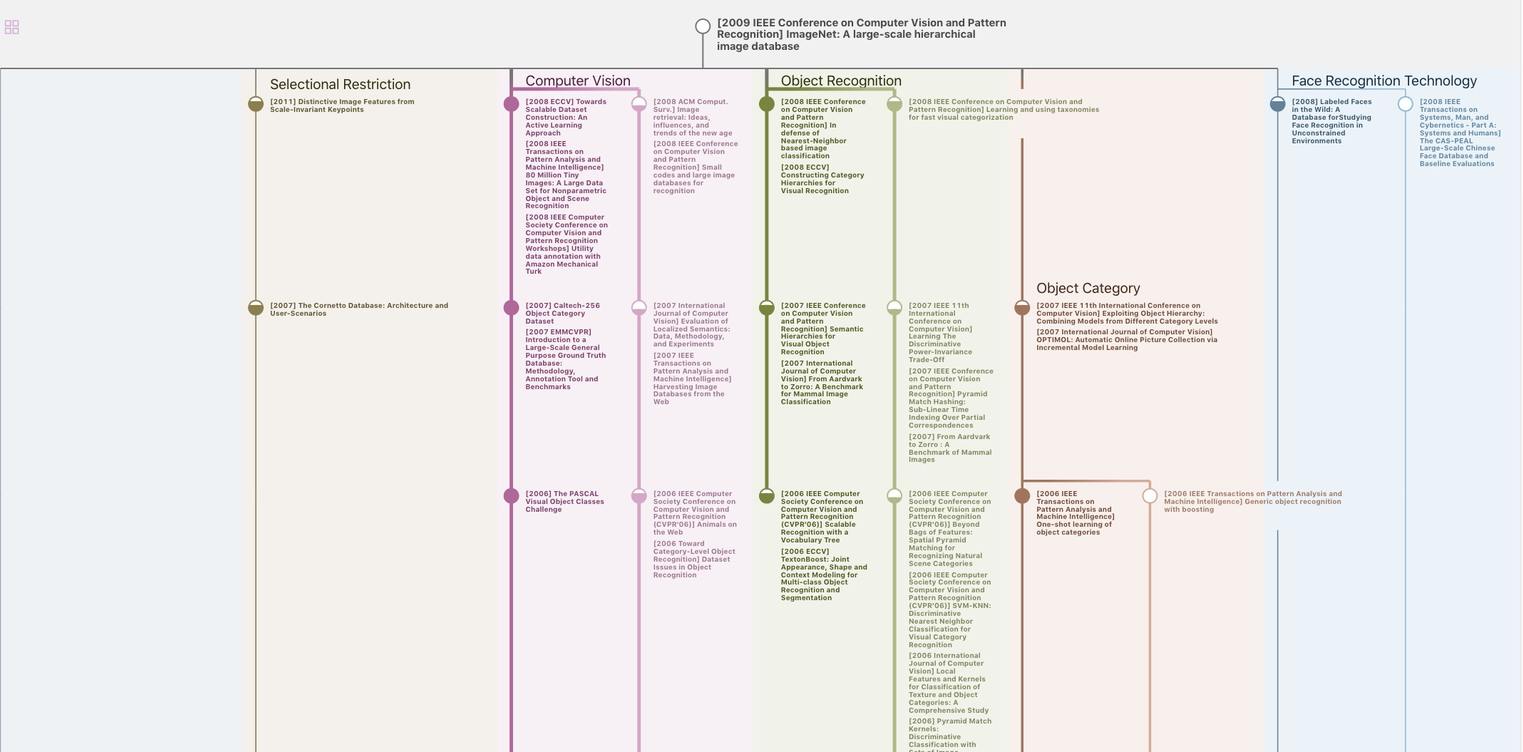
生成溯源树,研究论文发展脉络
Chat Paper
正在生成论文摘要