Global Adaptive Transformer for Cross-Subject Enhanced EEG Classification
IEEE transactions on neural systems and rehabilitation engineering : a publication of the IEEE Engineering in Medicine and Biology Society(2023)
摘要
Due to the individual difference, EEG signals from other subjects (source) can hardly be used to decode the mental intentions of the target subject. Although transfer learning methods have shown promising results, they still suffer from poor feature representation or neglect long-range dependencies. In light of these limitations, we propose Global Adaptive Transformer (GAT), an domain adaptation method to utilize source data for cross-subject enhancement. Our method uses parallel convolution to capture temporal and spatial features first. Then, we employ a novel attention-based adaptor that implicitly transfers source features to the target domain, emphasizing the global correlation of EEG features. We also use a discriminator to explicitly drive the reduction of marginal distribution discrepancy by learning against the feature extractor and the adaptor. Besides, an adaptive center loss is designed to align the conditional distribution. With the aligned source and target features, a classifier can be optimized to decode EEG signals. Experiments on two widely used EEG datasets demonstrate that our method outperforms state-of-the-art methods, primarily due to the effectiveness of the adaptor. These results indicate that GAT has good potential to enhance the practicality of BCI.
更多查看译文
关键词
Humans,Electroencephalography,Learning,Machine Learning,Software,Electric Power Supplies
AI 理解论文
溯源树
样例
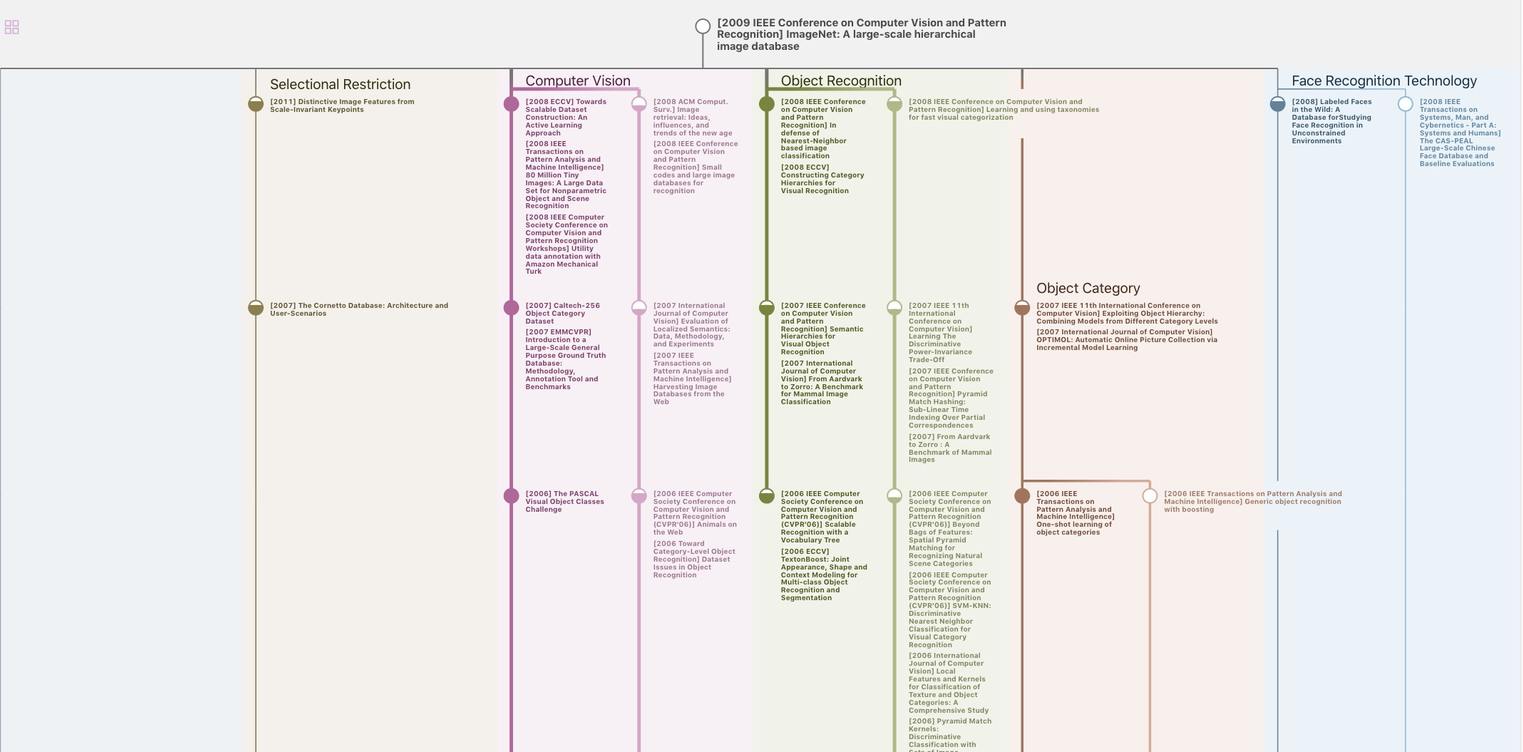
生成溯源树,研究论文发展脉络
Chat Paper
正在生成论文摘要