Land Cover Classification of UAV Remote Sensing Based on Transformer-CNN Hybrid Architecture.
Sensors (Basel, Switzerland)(2023)
摘要
High-precision land cover maps of remote sensing images based on an intelligent extraction method are an important research field for many scholars. In recent years, deep learning represented by convolutional neural networks has been introduced into the field of land cover remote sensing mapping. In view of the problem that a convolution operation is good at extracting local features but has limitations in modeling long-distance dependence relationships, a semantic segmentation network, DE-UNet, with a dual encoder is proposed in this paper. The Swin Transformer and convolutional neural network are used to design the hybrid architecture. The Swin Transformer pays attention to multi-scale global features and learns local features through the convolutional neural network. Integrated features take into account both global and local context information. In the experiment, remote sensing images from UAVs were used to test three deep learning models including DE-UNet. DE-UNet achieved the highest classification accuracy, and the average overall accuracy was 0.28% and 4.81% higher than UNet and UNet++, respectively. It shows that the introduction of a Transformer enhances the model fitting ability.
更多查看译文
关键词
deep learning, transformer, CNN, semantic segmentation, UAV remote sensing
AI 理解论文
溯源树
样例
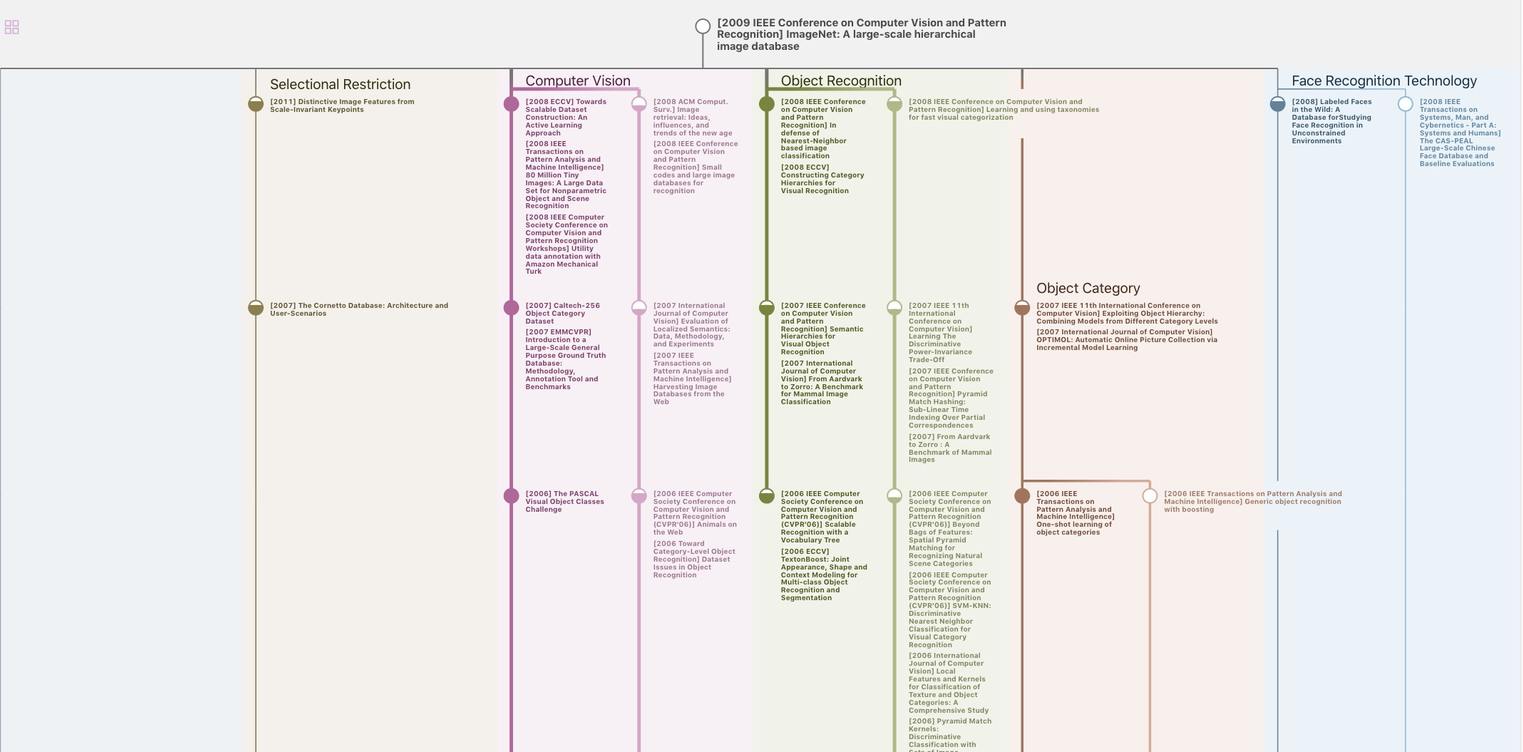
生成溯源树,研究论文发展脉络
Chat Paper
正在生成论文摘要