Increasing the Robustness of Deep Learning Models for Object Segmentation: A Framework for Blending Automatically Annotated Real and Synthetic Data
IEEE TRANSACTIONS ON CYBERNETICS(2024)
摘要
Recent problems in robotics can sometimes only be tackled using machine learning technologies, particularly those that utilize deep learning (DL) with transfer learning. Transfer learning takes advantage of pretrained models, which are later fine-tuned using smaller task-specific datasets. The fine-tuned models must be robust against changes in environmental factors such as illumination since, often, there is no guarantee for them to be constant. Although synthetic data for pretraining has been shown to enhance DL model generalization, there is limited research on its application for fine-tuning. One limiting factor is that the generation and annotation of synthetic datasets can be cumbersome and impractical for the purpose of fine-tuning. To address this issue, we propose two methods for automatically generating annotated image datasets for object segmentation, one for real-world and another for synthetic images. We also introduce a novel domain adaptation approach called filling the reality gap (FTRG), which can blend elements from real-world and synthetic scenes in a single image to achieve domain adaptation. We demonstrate through experimentation on a representative robot application that FTRG outperforms other domain adaptation techniques, such as domain randomization or photorealistic synthetic images, in creating robust models. Furthermore, we evaluate the benefits of using synthetic data for fine-tuning in transfer learning and continual learning with experience replay using our proposed methods and FTRG. Our findings indicate that fine-tuning with synthetic data can produce superior results compared to solely using real-world data.
更多查看译文
关键词
Synthetic data,Data models,Robots,Training,Adaptation models,Image segmentation,Annotations,Data annotation,deep learning (DL),digital twin,mixed reality,robotics,synthetic data
AI 理解论文
溯源树
样例
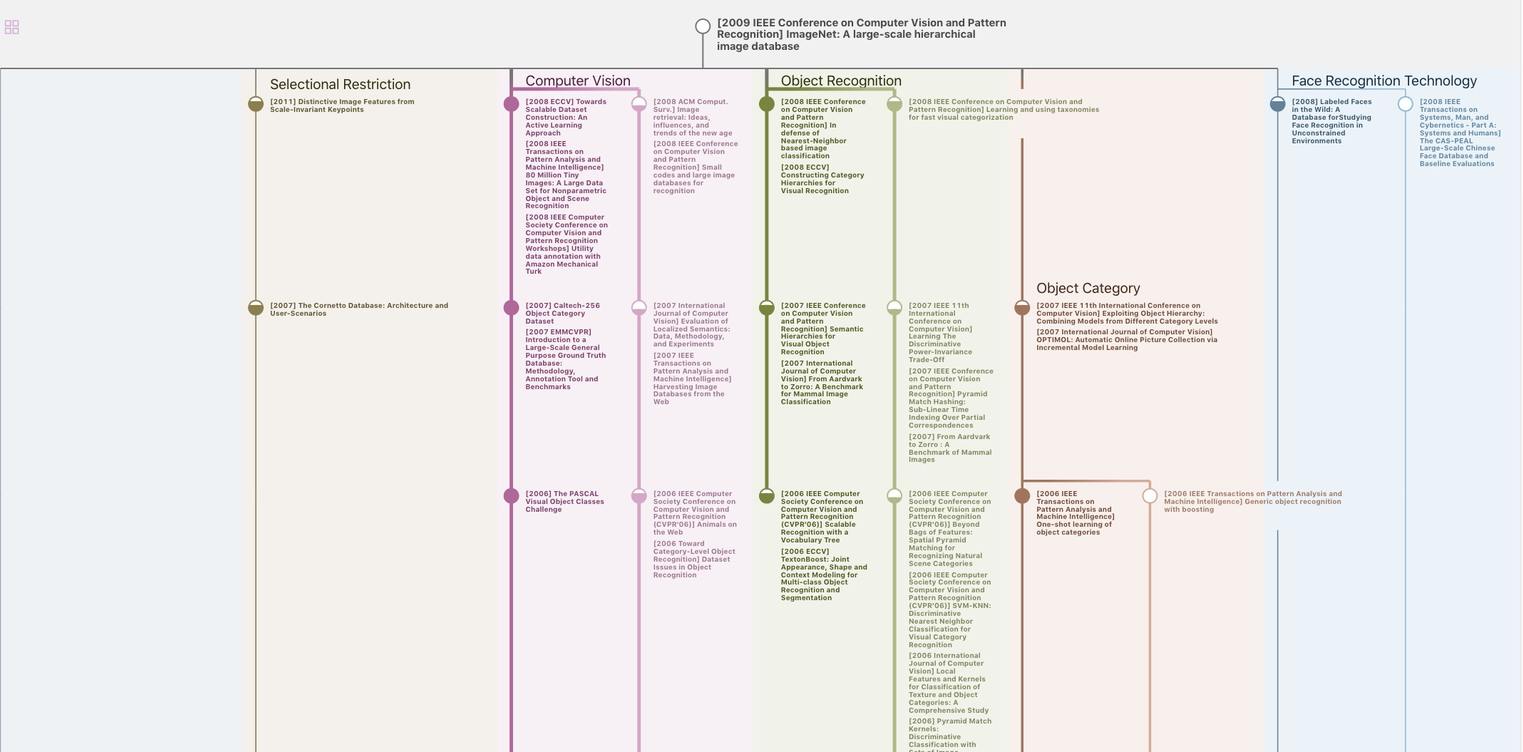
生成溯源树,研究论文发展脉络
Chat Paper
正在生成论文摘要