Towards Trajectory Forecasting from Detection.
IEEE transactions on pattern analysis and machine intelligence(2023)
摘要
Trajectory forecasting for traffic participants (e.g., vehicles) is critical for autonomous platforms to make safe plans. Currently, most trajectory forecasting methods assume that object trajectories have been extracted and directly develop trajectory predictors based on the ground truth trajectories. However, this assumption does not hold in practical situations. Trajectories obtained from object detection and tracking are inevitably noisy, which could cause serious forecasting errors to predictors built on ground truth trajectories. In this paper, we propose to predict trajectories directly based on detection results without relying on explicitly formed trajectories. Different from traditional methods which encode the motion cues of an agent based on its clearly defined trajectory, we extract the motion information only based on the affinity cues among detection results, in which an affinity-aware state update mechanism is designed to manage the state information. In addition, considering that there could be multiple plausible matching candidates, we aggregate the states of them. These designs take the uncertainty of association into account which relax the undesirable effect of noisy trajectory obtained from data association and improve the robustness of the predictor. Extensive experiments validate the effectiveness of our method and its generalization ability to different detectors or forecasting schemes.
更多查看译文
关键词
Trajectory forecasting, motion encoding, recurrent network, affinity measuring
AI 理解论文
溯源树
样例
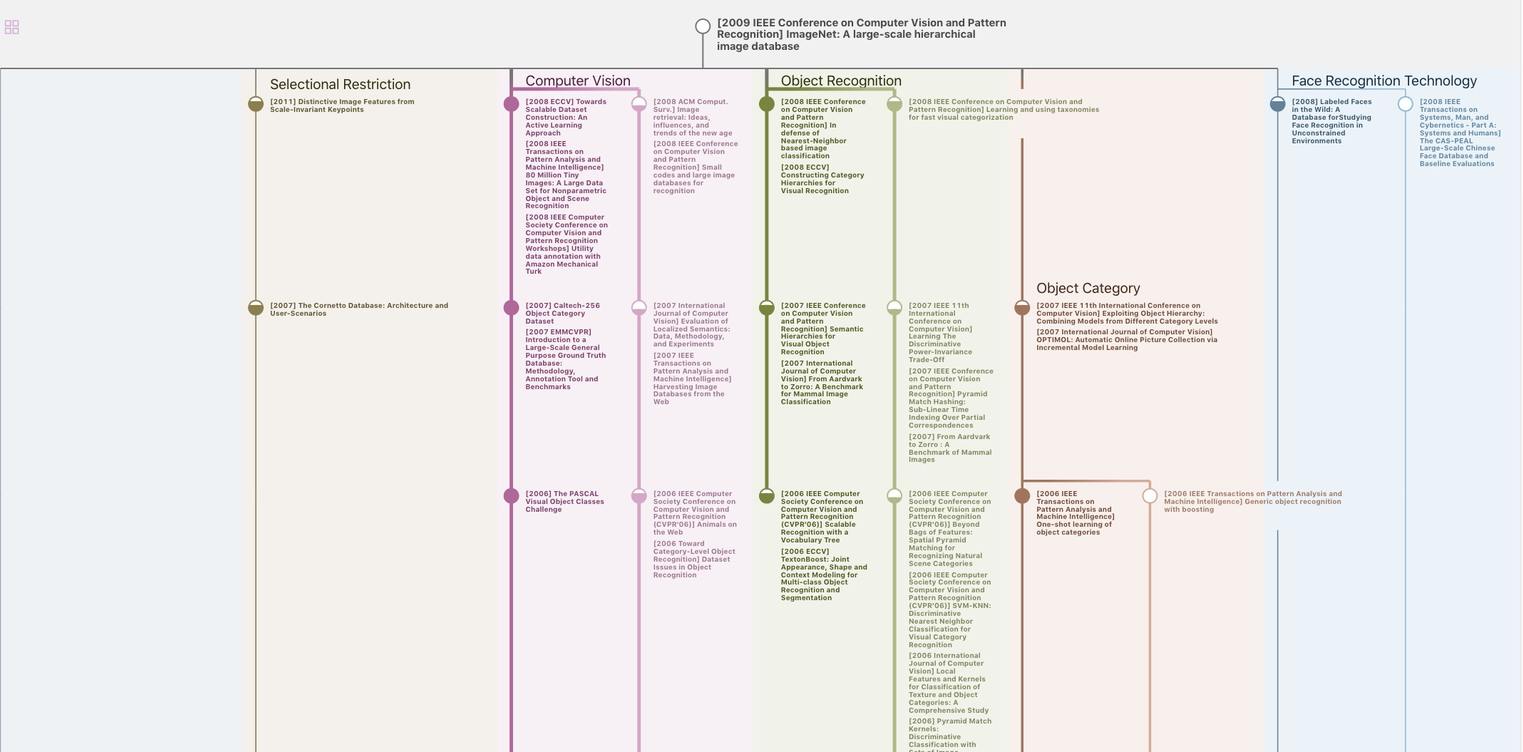
生成溯源树,研究论文发展脉络
Chat Paper
正在生成论文摘要