Human Activity Recognition Using Machine Learning Algorithms Based on IMU Data
2023 5th International Conference on Bio-engineering for Smart Technologies (BioSMART)(2023)
摘要
Enhancing Human Activity Recognition (HAR) using Inertial Measurement Unit (IMU) sensors has garnered significant attention in healthcare, particularly wearable devices. HAR plays a crucial role in prosthetic and wearable robotics by accurately identifying and responding to user movements, enabling personalized, adaptive, and intuitive control of these devices. IMU sensors capture both acceleration and angular velocity, delivering valuable data for HAR. These sensors find widespread use in prosthetic and wearable robotics, allowing for the detection and monitoring of user limb or body activities. Various Machine Learning algorithms can be leveraged further to advance HAR systems. These techniques hold promise in enhancing the accuracy and robustness of HAR systems, especially when dealing with complex and diverse movements. In this study, we implement four Machine Learning models, including Artificial Neural Networks (ANN), Decision Tree Classifiers (DTC), Random Forest Classifiers (RFC), and K-Nearest Neighbors (KNN). Our objective is to accurately recognize human activities such as walking upstairs, walking downstairs, walking normally, walking on the toe, walking on the heel, and performing sit-up tasks. Among these models, the RFC model exhibits superior performance compared to the others, achieving an impressive accuracy score of 97.67% across all evaluation metrics for activity recognition. This work is a foundational step in augmenting wearable robotics’ functionality, usability, and personalization, ultimately improving the quality of life for individuals with disabilities or injuries.
更多查看译文
关键词
Human Activity Recognition (HAR),Machine Learning,Inertial Measurement Unit (IMU),ANN,Random Forest,Decision Tree,KNN
AI 理解论文
溯源树
样例
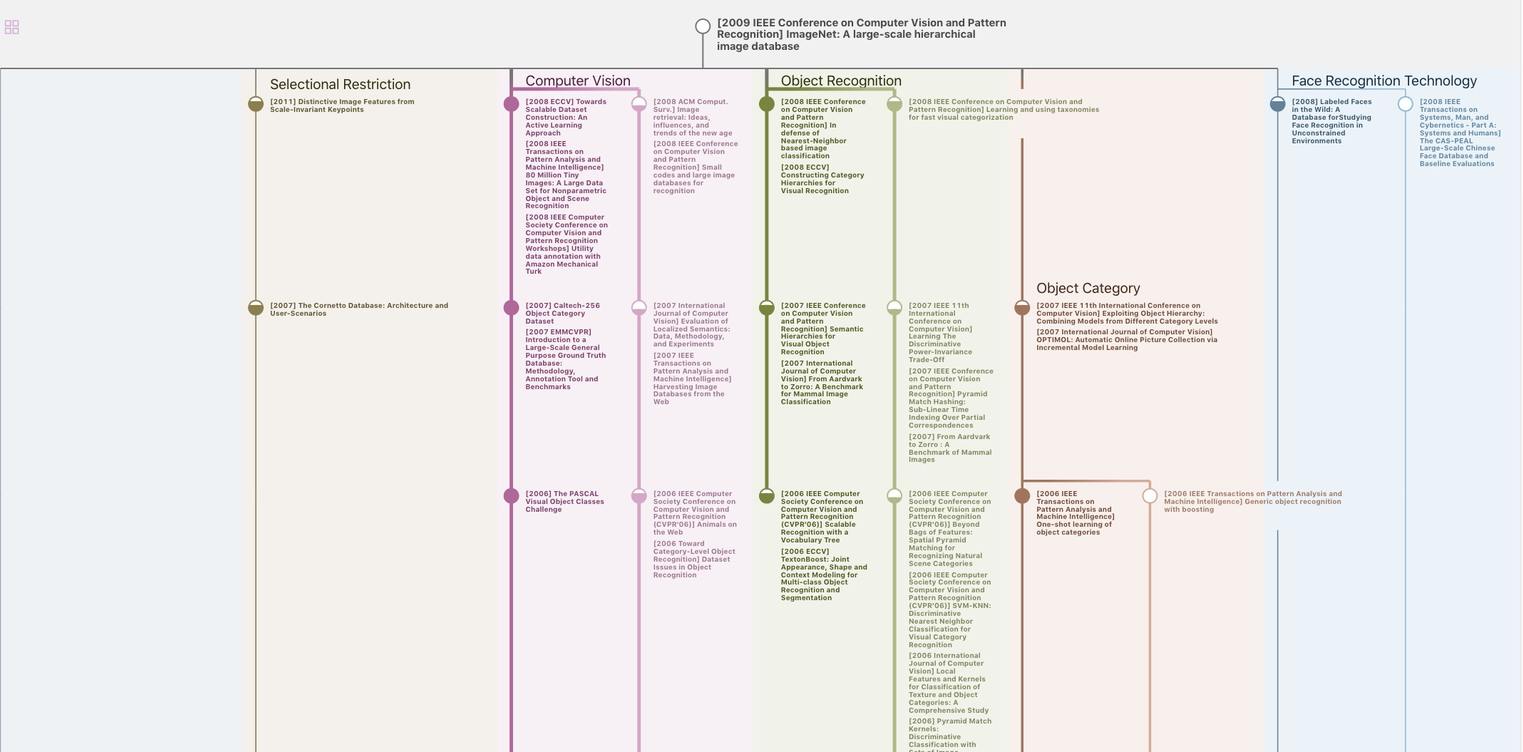
生成溯源树,研究论文发展脉络
Chat Paper
正在生成论文摘要