Goal-Conditioned Predictive Coding as an Implicit Planner for Offline Reinforcement Learning
NeurIPS(2023)
Abstract
Recent work has demonstrated the effectiveness of formulating decision making as a supervised learning problem on offline-collected trajectories. However, the benefits of performing sequence modeling on trajectory data is not yet clear. In this work we investigate if sequence modeling has the capability to condense trajectories into useful representations that can contribute to policy learning. To achieve this, we adopt a two-stage framework that first summarizes trajectories with sequence modeling techniques, and then employs these representations to learn a policy along with a desired goal. This design allows many existing supervised offline RL methods to be considered as specific instances of our framework. Within this framework, we introduce Goal-Conditioned Predicitve Coding (GCPC), an approach that brings powerful trajectory representations and leads to performant policies. We conduct extensive empirical evaluations on AntMaze, FrankaKitchen and Locomotion environments, and observe that sequence modeling has a significant impact on some decision making tasks. In addition, we demonstrate that GCPC learns a goal-conditioned latent representation about the future, which serves as an "implicit planner", and enables competitive performance on all three benchmarks.
MoreTranslated text
Key words
implicit planner,predictive coding,reinforcement learning,goal-conditioned
AI Read Science
Must-Reading Tree
Example
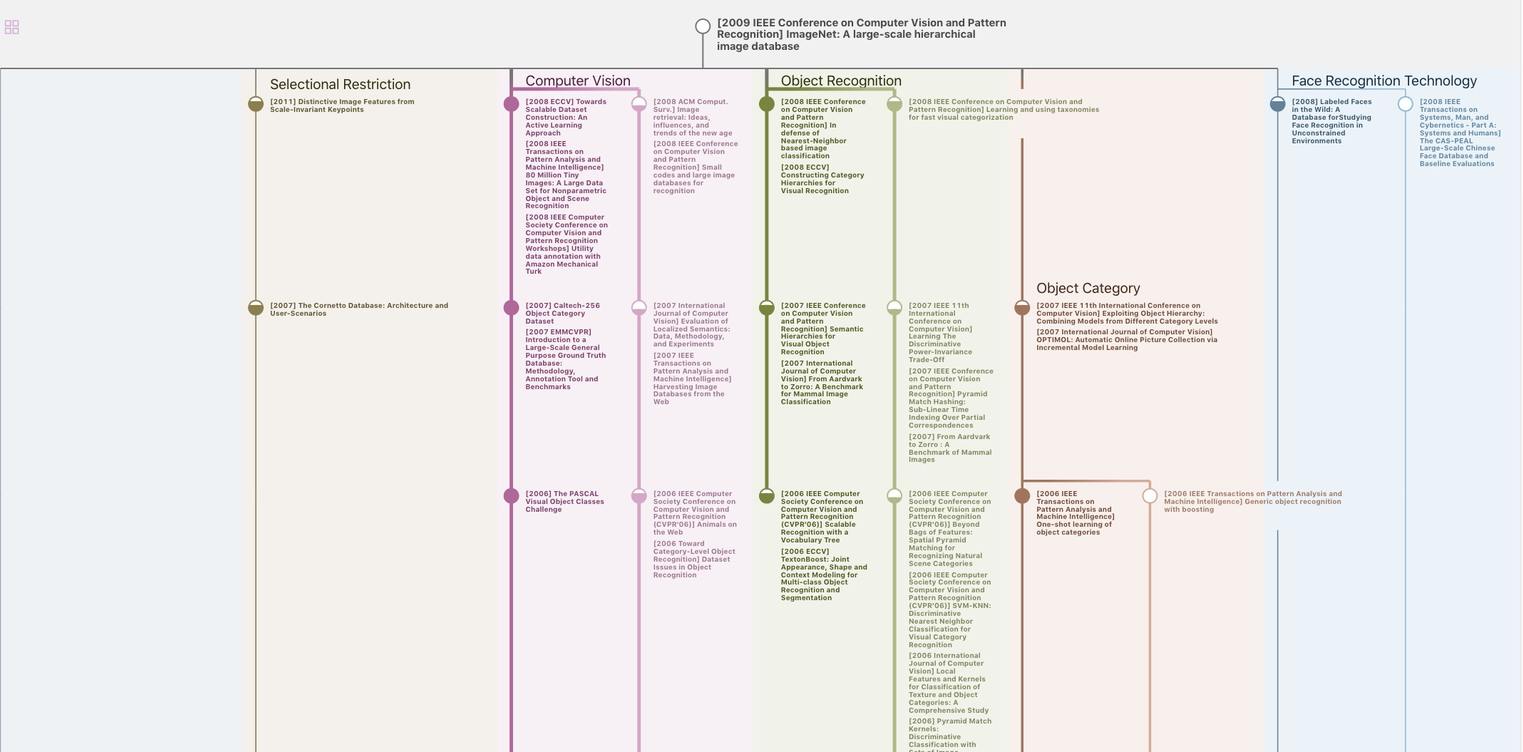
Generate MRT to find the research sequence of this paper
Chat Paper
Summary is being generated by the instructions you defined