The prediction of drug sensitivity by multi-omics fusion reveals the heterogeneity of drug response in pan-cancer
Computers in Biology and Medicine(2023)
摘要
Cancer drug response prediction based on genomic information plays a crucial role in modern pharmacogenomics, enabling individualized therapy. Given the expensive and complexity of biological experiments, computational methods serve as effective tools in predicting cancer drug sensitivity. In this study, we proposed a novel method called Multi-Omics Integrated Collective Variational Autoencoders (MOICVAE), which leverages integrated omics knowledge, including genomic and transcriptomic data, to fill in missing cancer-drug associations and enhance drug sensitivity prediction. Our method employs an encoder-decoder network to learn latent feature representations from cell lines. These learned feature vectors are then fed into a collective variational autoencoder network to train an association matrix. We evaluated MOICVAE on the GDSC and CCLE benchmark datasets using 10-fold cross-validation and achieved impressive AUCs of 0.856 and 0.808, respectively, outperforming state-of-the-art methods. Furthermore, on the TCGA dataset, consisting of 25 drugs across 7 cancer types, MOICVAE exhibited an average AUC of 0.91 in predicting drug sensitivity. Additionally, significant differences were observed in survival, tumor inflammatory assessment, and tumor microenvironment between the predicted drug-sensitive and drug-resistant groups. These results are consistent with predictions made on the METABRIC dataset. Moreover, we discovered that fusing omics data based on mRNA and CNV (copy number variations) yielded superior results in drug sensitivity prediction. MOICVAE not only achieved higher accuracy in drug sensitivity prediction but also provided additional value for combining immunotherapy with chemotherapy, offering patients with more precise treatment options. The code and dataset for MOICVAE are freely available at https://github.com/wanggnoc/MOICVAE.
更多查看译文
AI 理解论文
溯源树
样例
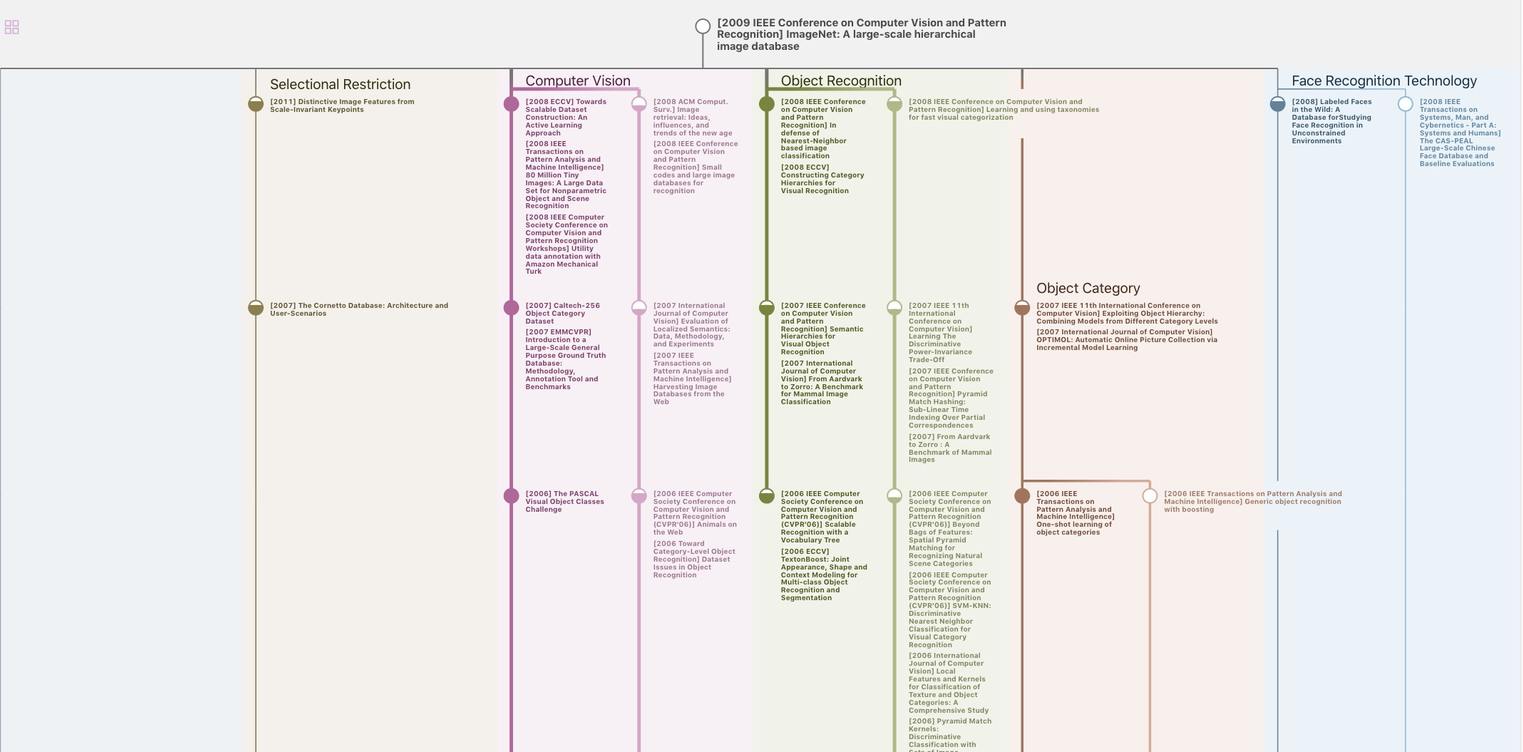
生成溯源树,研究论文发展脉络
Chat Paper
正在生成论文摘要