Structure invariance-driven collaborative contrastive network for EEG decoding
Biomedical Signal Processing and Control(2023)
摘要
With a large amount of augmented training data generated by data augmentation (DA) methods, deep neural networks (DNN) have achieved promising performance in decoding electroencephalogram (EEG) neural patterns for brain-computer interface (BCI). However, most current methods neglect the data-structure information available in different views of augmented EEG datasets. In particular, the invariance of data-structure information can improve model generalization ability but unexploited. In this paper, we develop the novel Structure Invariance-driven Collaborative Contrastive Network (SICCNet) for EEG recognition. It is a framework with both implicit and explicit constraints to exploit invariant data-structure information. SICCNet contains two structureguided contrastive learning subnets to perform collaborative contrasts between pairs of normalized embeddings derived from different augmented views. First, implicit constraint is introduced with the Inter-Segment Mixupbased (InSegMix) EEG data augmentation strategy to generate new EEG views without introducing extra noise. Explicit constraint is then introduced through the collaborative contrastive (co-contrastive) regularization scheme to exploit the invariant data-structure information from multiple views. The scheme can direct the model to focus on the invariant data-structure information and consequently enhance the discrimination ability of the model. Extensive experiments on three public motor-imagery (MI) based EEG datasets have demonstrated the outstanding performance of SICCNet when compared with other state-of-the-art methods, indicating its promising potential in improving BCI system.
更多查看译文
关键词
Electroencephalogram,Deep neural network,Data augmentation,Contrastive learning,Motor imagery
AI 理解论文
溯源树
样例
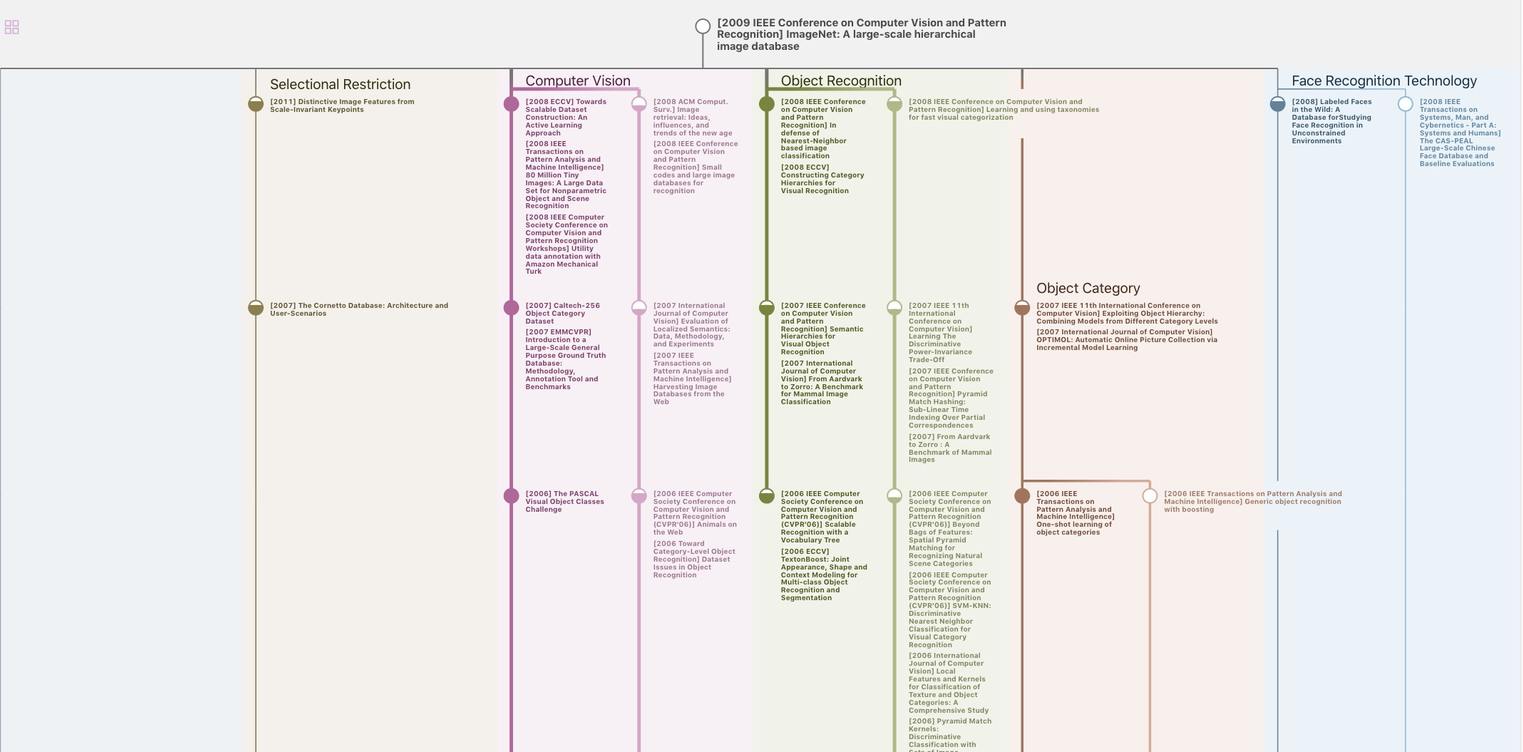
生成溯源树,研究论文发展脉络
Chat Paper
正在生成论文摘要