SimPPG: Self-supervised photoplethysmography-based heart-rate estimation via similarity-enhanced instance discrimination
Smart Health(2023)
摘要
Photoplethysmography (PPG) is an optical measurement technique to detect blood volume changes in the microvascular bed of target tissues and has been a widely used technique adopted by wearable devices to evaluate an individual’s health condition. However, as motion and noise artifacts continue to pose manifold challenges toward the task of remote estimation of this signal (popularly known as rPPG), an authentic approximation of rPPG signals is of immense interest and convenience. In this work, we present a self-supervised learning-based regression framework that can reliably estimate the heart rate by accurately approximating a PPG signal from a participant’s videos with sufficient coverage of their skin regions, in the presence of different types of local noises. The idea is that the PPG signals generated from the different parts of an individual will be nearly identical, while these signals from two different individuals may be comparably different. Though the severity of difference may vary based on the variance in their health conditions. Motivated by this intuition, we augment an rPPG signal extracted from a given facial landmark of an individual, using various other rPPG signals extracted from the neighboring facial landmarks of the same individual, to align their unique personalized patterns. Specifically, we develop a robust Similarity-aware PPG (SimPPG) based heart-rate estimation scheme that adopts the instance discrimination method within a self-supervised learning setting to perform two-fold objectives: (1) discriminating every positive pair (rPPGs from different local skin regions of a given individual) from all negative pairs (rPPGs from different local skin regions of different individuals in a batch); (2) enforcing the alignments in the individual’s respective heart-rate predictions with that of the corresponding ground truth PPG signals in parallel. Experiments using the large-scale UBFC-Phys dataset and our in-house data collection not only show a remarkable performance of SimPPG (MAEhr=1.89bpm, which is 77% improvement compared to the existing baseline and MSE=2.91 in approximating the PPG signal), but also show its effectiveness in terms of handling various skin features across demographics. In a limited data environment, SimPPG is reported to have used only a random 75% of the available training collection to attain a competitive performance compared to several state-of-the-art models.
更多查看译文
关键词
Remote photoplethysmography (rPPG) approximation,Heart-rate estimation,Evaluation,Self-supervised learning,Instance discrimination
AI 理解论文
溯源树
样例
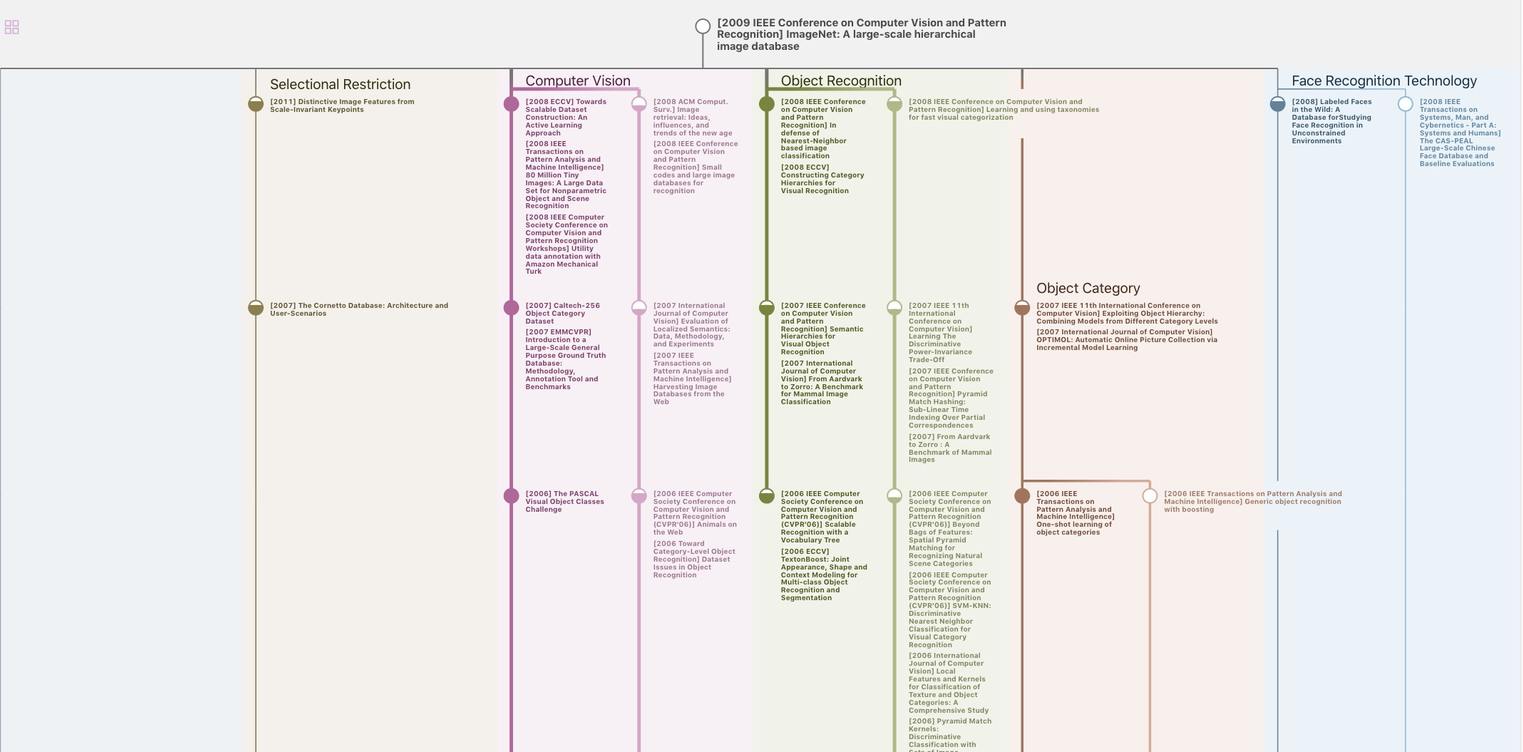
生成溯源树,研究论文发展脉络
Chat Paper
正在生成论文摘要