Exploring the time-lagged causality of process variables from injection molding machines
Procedia Computer Science(2023)
摘要
This paper analyses the process variable data from injection molding processes to identify the key causal interactions between influential and dependent process variables in different product categories using variable lagged transfer entropy measure. Variable lagged transfer entropy measurements can be applied for data with variable time lags as in our case. We use variable lagged transfer entropy measurements to construct directed networks by calculating significant pairs of process variables for each production process (of each material). The directed network provides influential and dependent process variables for different materials. Furthermore, the constructed network for each material is used in a machine learning model for feature selection for each material category, where the edges are the input features and the material categories are the output labels with which to classify the material categories. Our analysis shows that the edge features of the inferred networks can classify different material categories and identify key features representing time-lagged causal interactions of the process variables. Additionally, the aggregated network based on the causal interactions that are significantly consistent in all the samples (production data of each product) irrespective of material categories. The aggregated network shows only 10 process variables are key influencers of all production processes.
更多查看译文
关键词
process variables,causality,machines,injection,time-lagged
AI 理解论文
溯源树
样例
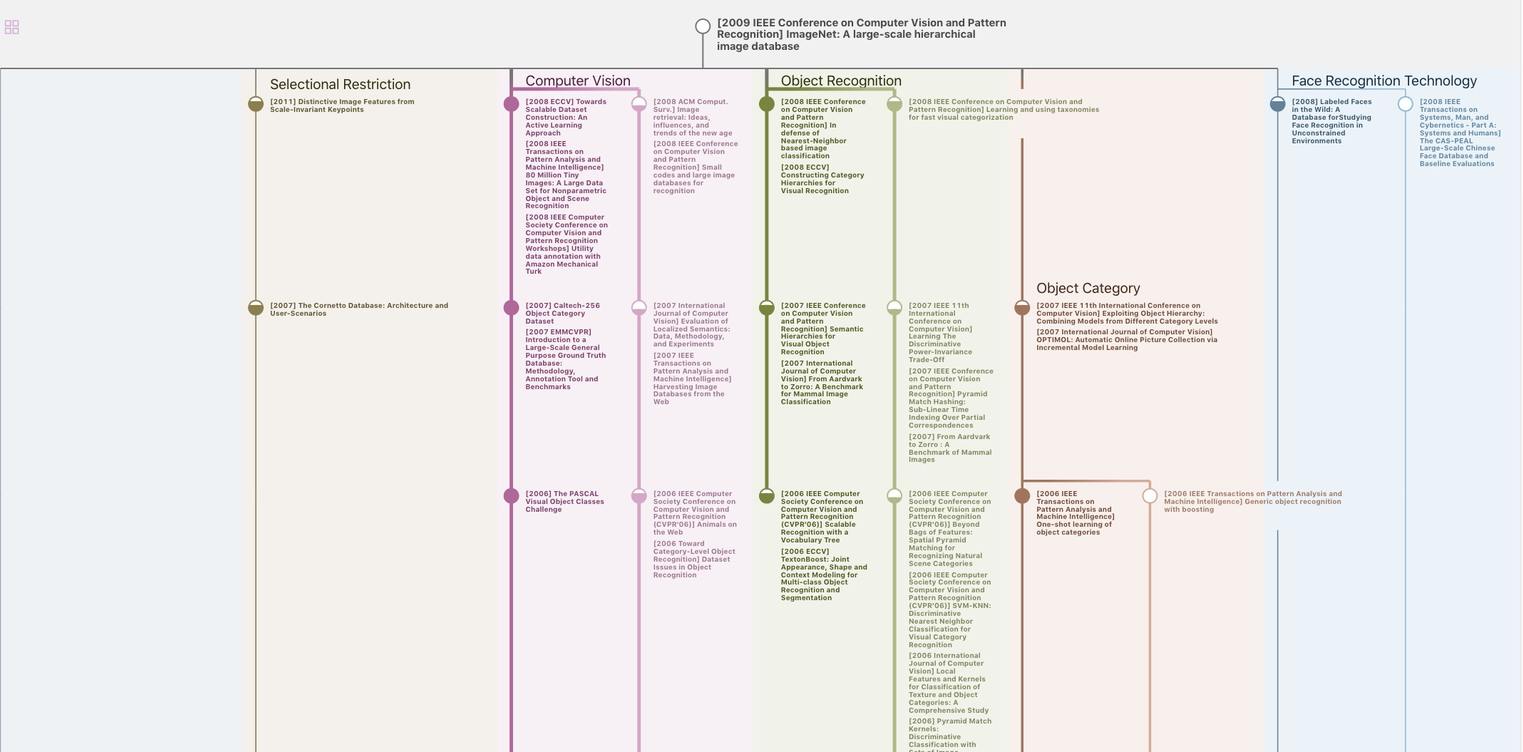
生成溯源树,研究论文发展脉络
Chat Paper
正在生成论文摘要