CPNet: Conditionally parameterized graph convolutional network for traffic forecasting
Physica A: Statistical Mechanics and its Applications(2023)
摘要
Traffic forecasting has attracted increasing attention to its vital role in intelligent transportation systems (ITS), many approaches have been proposed for improving the performance of traffic forecasting. Aiming at capturing complex spatial and temporal dependencies while maintaining efficient inference, we propose a novel model, named Conditionally Parameterized Graph Convolutional Network (CPNet), to model the dynamics of traffic data from spatial and temporal dimensions for traffic forecasting. Specifically, in the temporal dimension, we design a novel multi-scale temporal convolution module, which captures the temporal dynamics of traffic data from different scales. It is beneficial for both long and short-term forecasting. In the spatial dimension, we develop a conditional parameterized graph convolution module to exploit the spatial dependencies in different ranges. In addition, an attention layer is designed to model the nonlinear and dynamic features. Extensive experiments on four real-world datasets demonstrate the superiority of CPNet against the state-of-the-art baseline models.
更多查看译文
关键词
Traffic prediction,Temporal correlations,Spatial correlations,Attention mechanism,Graph convolution network
AI 理解论文
溯源树
样例
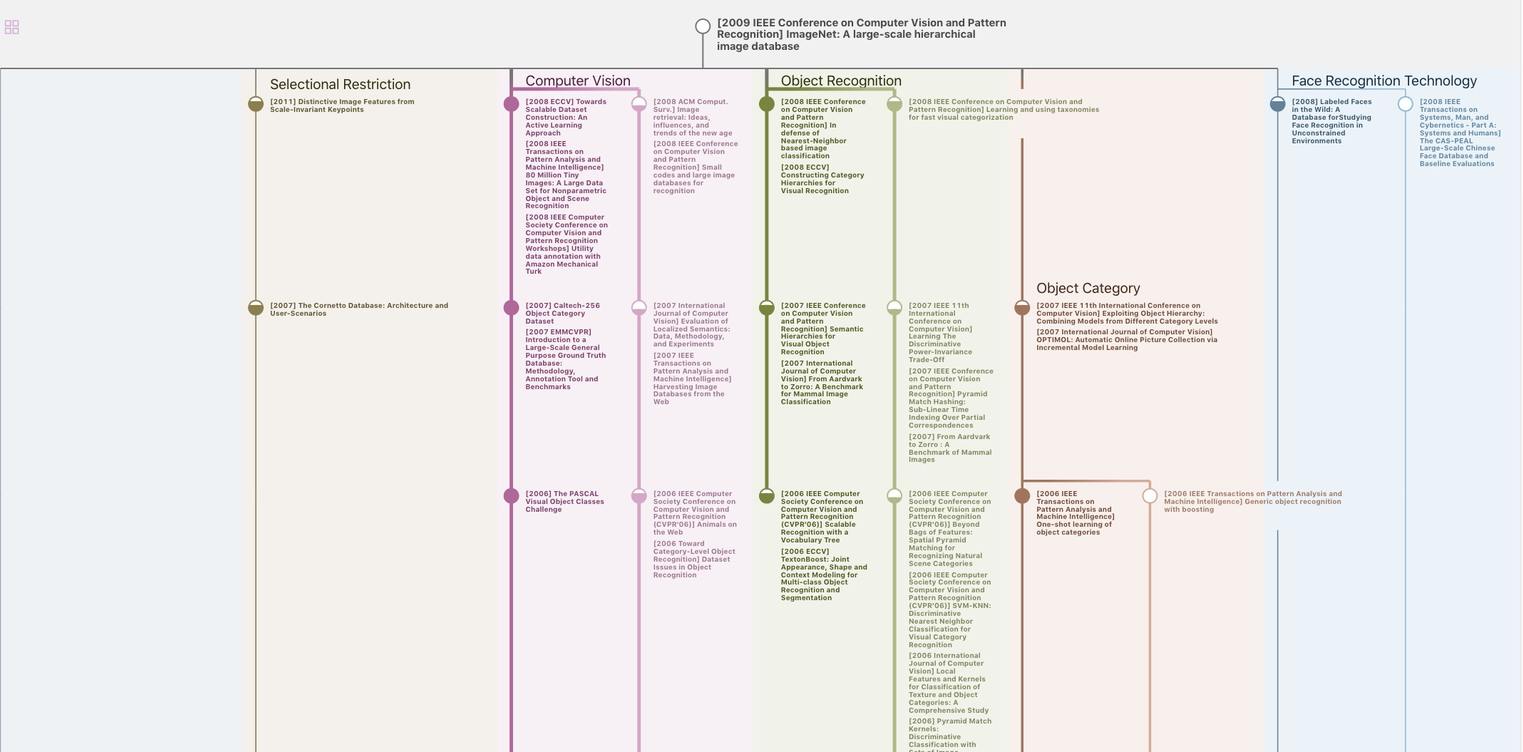
生成溯源树,研究论文发展脉络
Chat Paper
正在生成论文摘要