Quantifying time series complexity by multi-scale transition network approaches
Physica A: Statistical Mechanics and its Applications(2023)
摘要
Complex network approaches for nonlinear time series analysis are still under fast developments. In this work, we propose a set of entropy measures to characterize the multi-scale transition networks which are constructed from nonlinear time series. These entropy measures compare the distances between an empirical distribution P to a uniform distribution Pe, which are achieved via the multi-scale node transition matrix from different perspectives of out-link transitions, and in-link transitions, respectively. In addition, the entropy measures show convergence to zeros for white noise while non-zero values for deterministic chaotic processes. In correlated stochastic processes, the convergence rates are influenced by the correlation length. We show that entropy measures based on transition complexity are able to capture different dynamical states, i.e., tracking routes to chaos and dynamical hysteresis. In the experimental EEG analysis, we show that epileptic brain states are successfully distinguished from healthy control by all entropy measures. & COPY; 2023 Elsevier B.V. All rights reserved.
更多查看译文
关键词
Time series networks,Nonlinear dynamics,Dynamical hysteresis,Multi-scale entropy,Transition networks
AI 理解论文
溯源树
样例
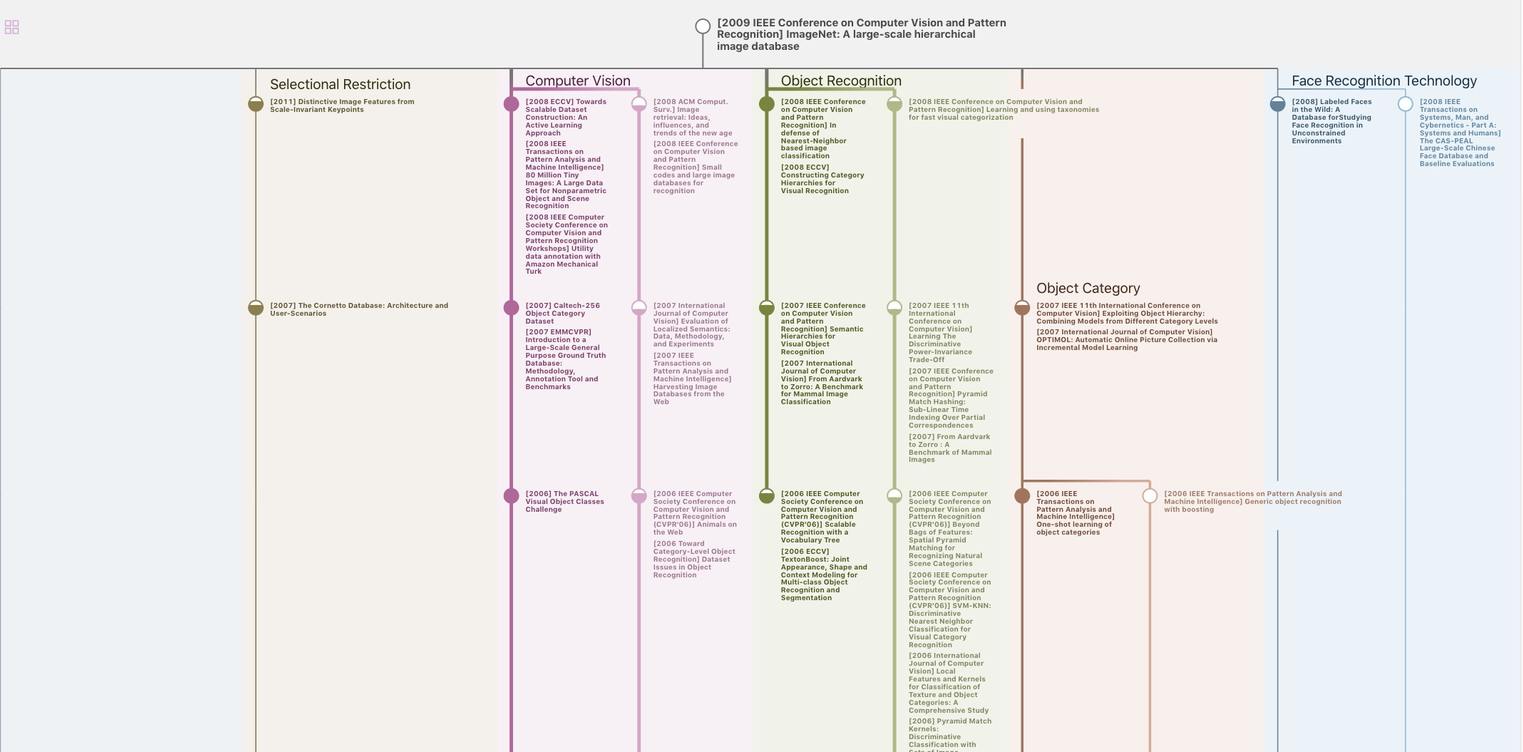
生成溯源树,研究论文发展脉络
Chat Paper
正在生成论文摘要