Multi-granularity knowledge distillation and prototype consistency regularization for class-incremental learning
Neural Networks(2023)
摘要
Deep neural networks (DNNs) are prone to the notorious catastrophic forgetting problem when learning new tasks incrementally. Class-incremental learning (CIL) is a promising solution to tackle the challenge and learn new classes while not forgetting old ones. Existing CIL approaches adopted stored representative exemplars or complex generative models to achieve good performance. However, storing data from previous tasks causes memory or privacy issues, and the training of generative models is unstable and inefficient. This paper proposes a method based on multi-granularity knowledge distillation and prototype consistency regularization (MDPCR) that performs well even when the previous training data is unavailable. First, we propose to design knowledge distillation losses in the deep feature space to constrain the incremental model trained on the new data. Thereby, multi-granularity is captured from three aspects: by distilling multi-scale self-attentive features, the feature similarity probability, and global features to maximize the retention of previous knowledge, effectively alleviating catastrophic forgetting. Conversely, we preserve the prototype of each old class and employ prototype consistency regularization (PCR) to ensure that the old prototypes and semantically enhanced prototypes produce consistent prediction, which excels in enhancing the robustness of old prototypes and reduces the classification bias. Extensive experiments on three CIL benchmark datasets confirm that MDPCR performs significantly better over exemplar-free methods and outperforms typical exemplar-based approaches.
更多查看译文
关键词
prototype consistency regularization,learning,knowledge,multi-granularity,class-incremental
AI 理解论文
溯源树
样例
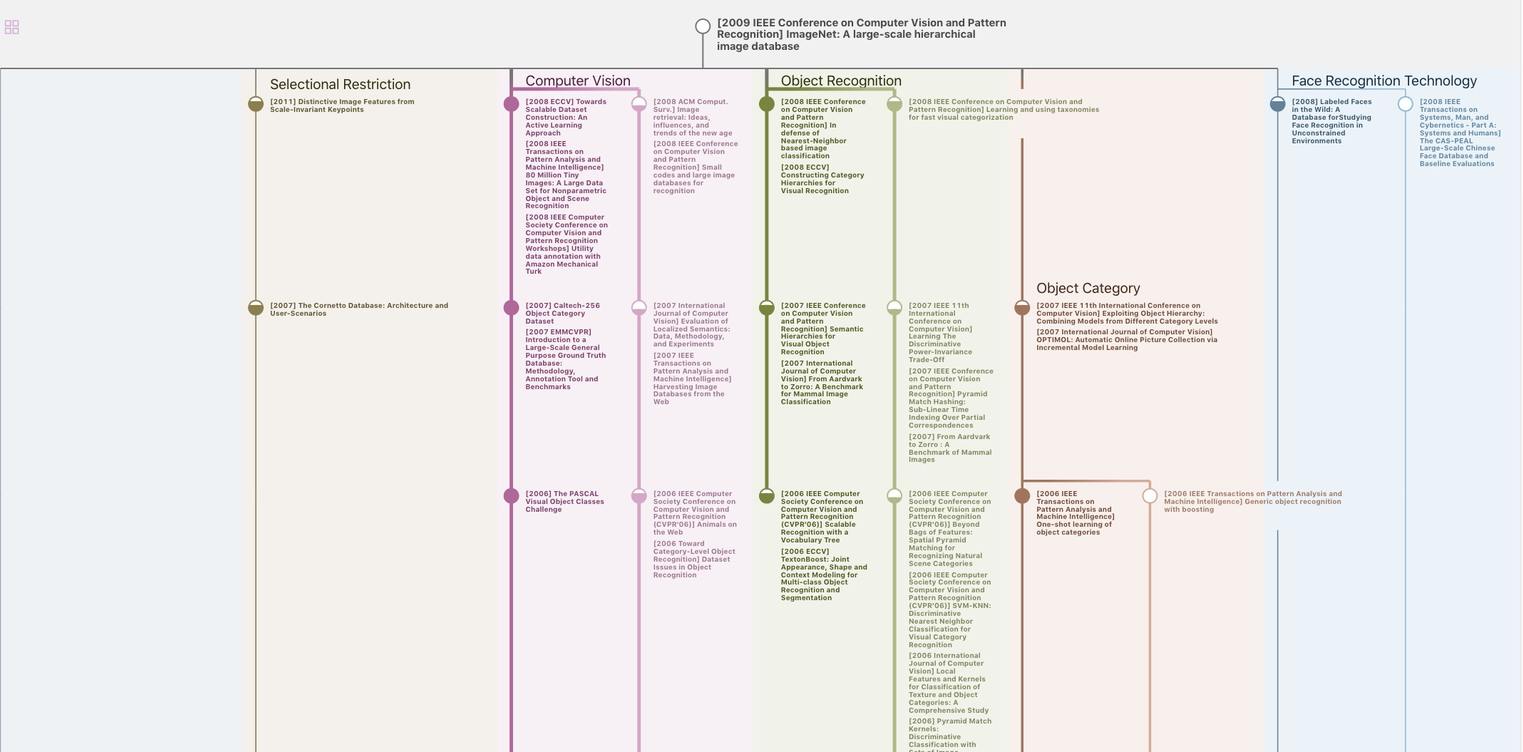
生成溯源树,研究论文发展脉络
Chat Paper
正在生成论文摘要