Machine learning model for preoperative assessment of ultrasound diathermy with implants
International Journal of Thermal Sciences(2023)
摘要
Ultrasound diathermy is a medical device commonly used in clinical practice to treat contractures, especially for patients with metal implants. However, the effects of thermotherapy tend to vary from person to person as it is dependent on the temperature distribution within soft tissues. As a result, a convenient tool for preoperative assessment is needed to avoid hyperthermia. In this study, a deep learning architecture based on the long short-term memory (LSTM) model as a workhorse is proposed to perform preoperative assessment for ultrasound diathermy with an operating frequency of 3 MHz and treatment duration of 60 s. The dataset was generated through finite element simulations validated by in-vitro experiments. Numerical examples are provided to demonstrate that the proposed model can accurately predict the temperature history curves at different locations along the acoustic axis for different heating profiles, hydrogel thicknesses, and implant thicknesses while maintaining a maximum root mean square error (RMSE) of less than 0.25 °C. It is noted that the peak temperature during ultrasound diathermy was found not to occur at the intersection point of the acoustic axis and the interface between the phantom and implant layers. The proposed model can also generate the temperature distribution in the phantom layer along the acoustic axis for various hydrogel thicknesses and implant thicknesses for preoperative assessment.
更多查看译文
关键词
ultrasound diathermy,preoperative assessment,implants,machine learning
AI 理解论文
溯源树
样例
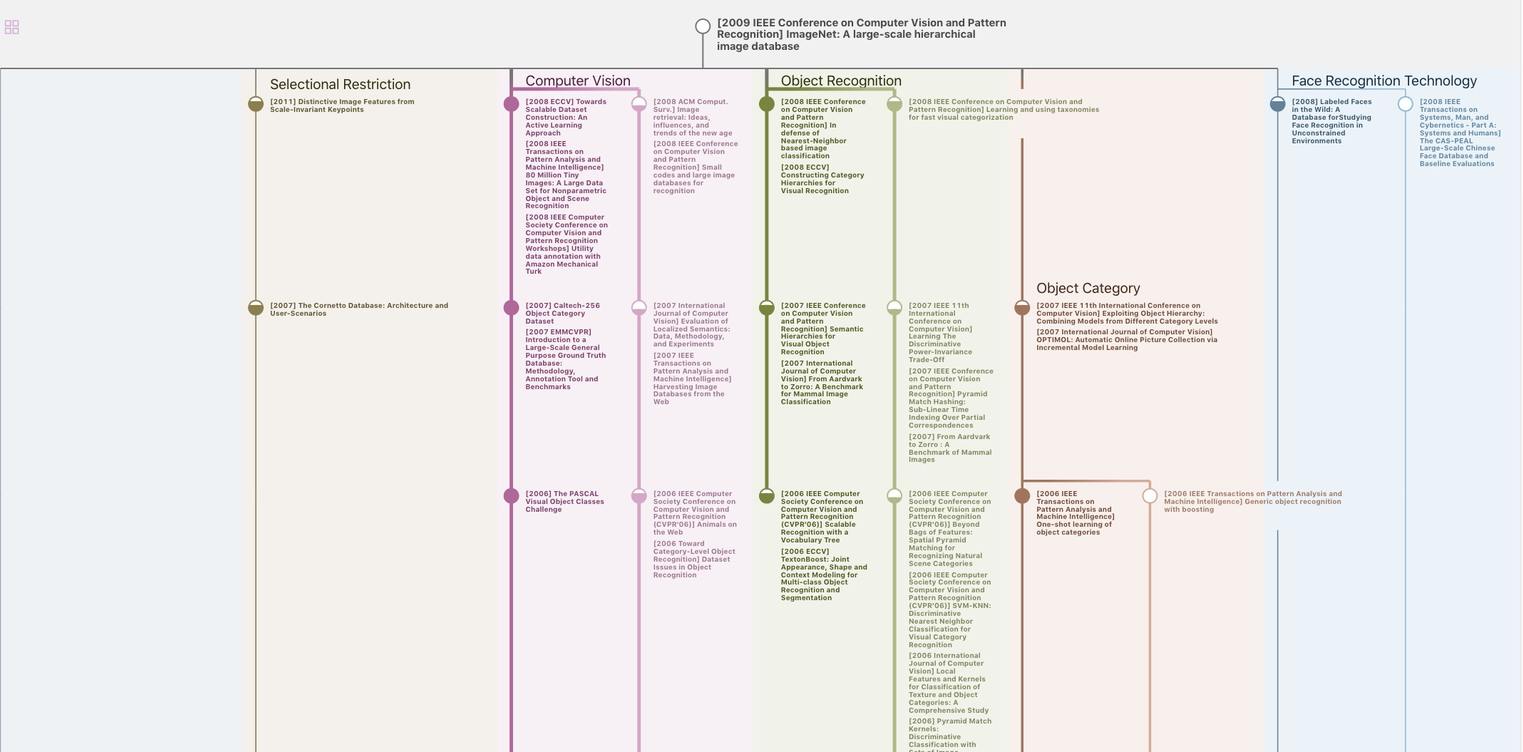
生成溯源树,研究论文发展脉络
Chat Paper
正在生成论文摘要