Intrusion Detection using hybridized Meta-heuristic techniques with Weighted XGBoost Classifier
Expert Systems with Applications(2023)
摘要
Due to the widespread global internet services, service providers and users face a primary problem defending their systems, specifically from a new category of attacks and breaches. Network Intrusion Detection system (NIDS) assesses the network packets and reports low-security violations to respective system administrators. In the case of large imbalance datasets with more non-relevant features, the accuracy in classifying ad predicting precise intrusions needs to be improved. Moreover, most state-of-art intrusion-detection models based on machine learning may face high false-positive rates, imbalanced data with low training performance, low accuracy in detection, and complexity in optimization of feature selection aiding classification for impersonation attacks. Hence to overcome those complications, the present study deliberates an efficient IDS Modified Wrapper-based Whale Sine–cosine algorithm (MWWSCA) with Weighted Extreme Gradient Boosting (XgBoost) Classifier. The proposed model hybridizes the modified wrapper Whale Optimization approach and Sine–Cosine algorithm feature selection method to pick out the most discriminative, associated, and approximate best optimal features, enhancing the quality of prediction, not to fall on towards optimal local solution. Moreover, it balances out the exploitation and exploration phase of the model. However, the algorithm’s performance may decline on classifying the multi-attack and binary attacks accurately and may be prone to an imbalance in classes; thus, a Weighted XGBoost classifier with regularization of the loss function is implemented in binary and multi-classification. It utilizes the best optimal features, assign high weights to weak minor class features, and handles class imbalance issues. Overfitting of the model is tackled through regularization in the loss function during the stage of feature classification. The experimental outcomes and comparative assessment, in multi-class and binary attack classification from UNSW-NB15 and CICIDS datasets, explicated outperforming results with high accuracy, Precision, Recall, and F1-Score metrics.
更多查看译文
关键词
weighted xgboost classifier,meta-heuristic
AI 理解论文
溯源树
样例
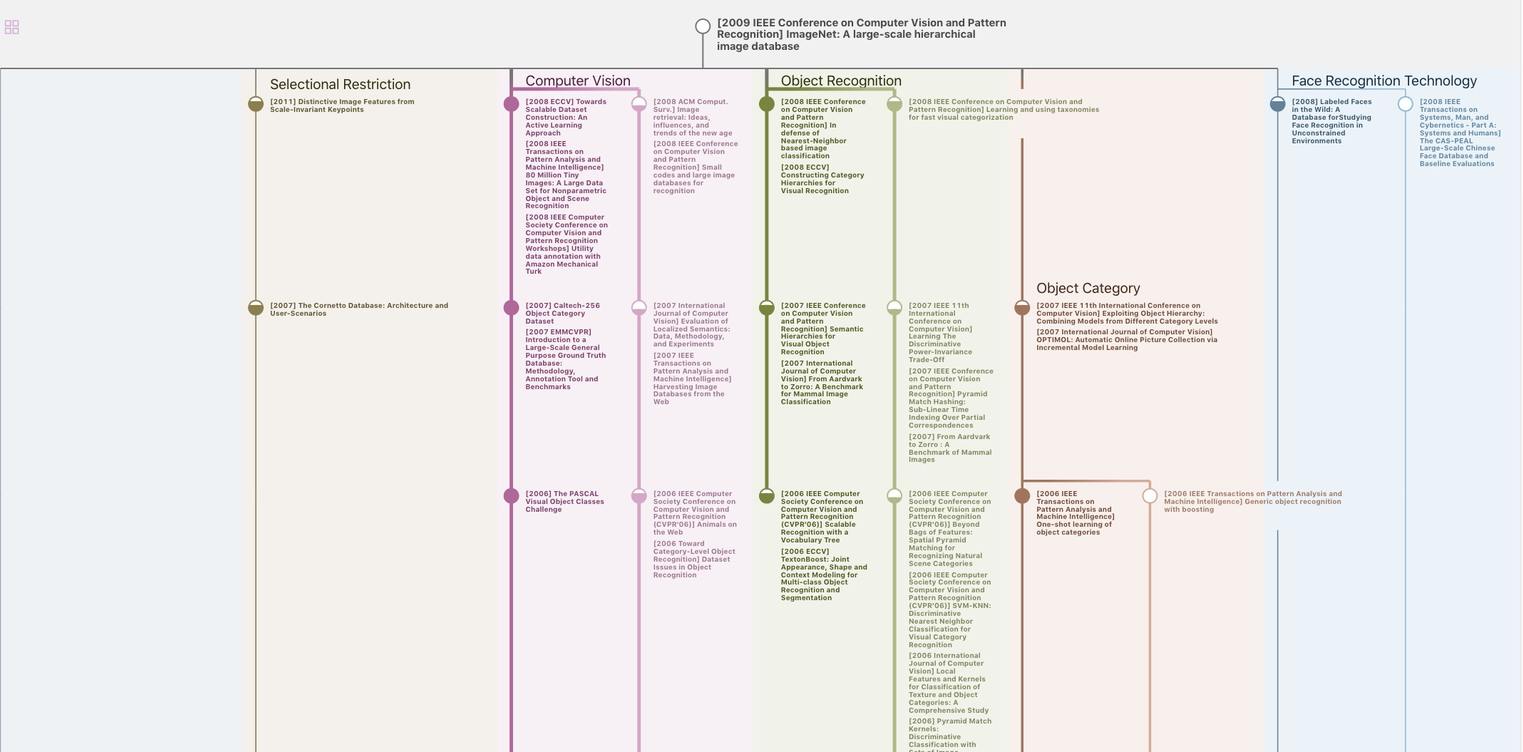
生成溯源树,研究论文发展脉络
Chat Paper
正在生成论文摘要