Expanded feature space-based gradient boosting ensemble learning for risk prediction of type 2 diabetes complications
Applied Soft Computing(2023)
摘要
As a metabolic disease, diabetes is a serious threat to human health, in which type 2 diabetes (T2D) constitutes about 90% of diabetic cases. As the disease progresses, T2D often comes with various complications such as kidney disease and retinopathy, which may cause huge threats to patients’ health. In recent years, complication prediction of T2D has gained wide research interest as early disease detection and management can effectively reduce the risk of death. In this paper, to break through the limitation of the available data and achieve higher overall prediction accuracy, an improved feature space-based gradient boosting regression tree ensemble (IFS-GBRTE) approach is proposed for the risk prediction of developing T2D complications. Specifically, the original feature space is expanded based on existing feature generation theories. Then the new feature space is refined by comparing the total contribution of each feature during the construction of a classification and regression tree (CART) under a cross-validation scheme. Finally, a gradient boosting ensemble algorithm using CART as base learners is utilized for model training. The prediction model is validated on the T2D data provided by the China National Clinical Medical Science Data Center. The experimental results show that the proposed IFS-GBRTE achieves 82.49% accuracy and realizes better generalization ability than compared single models and ensemble learning models, meanwhile it is helpful to obtain more effective predictive variables for improving the accuracy of risk prediction of T2D complications, which is of great significance for achieving the early prevention, screening and nursing of complications, and further reducing mortality and save medical resources.
更多查看译文
关键词
Diabetes complications,Risk prediction,Feature space expansion,Gini index,Gradient boosting
AI 理解论文
溯源树
样例
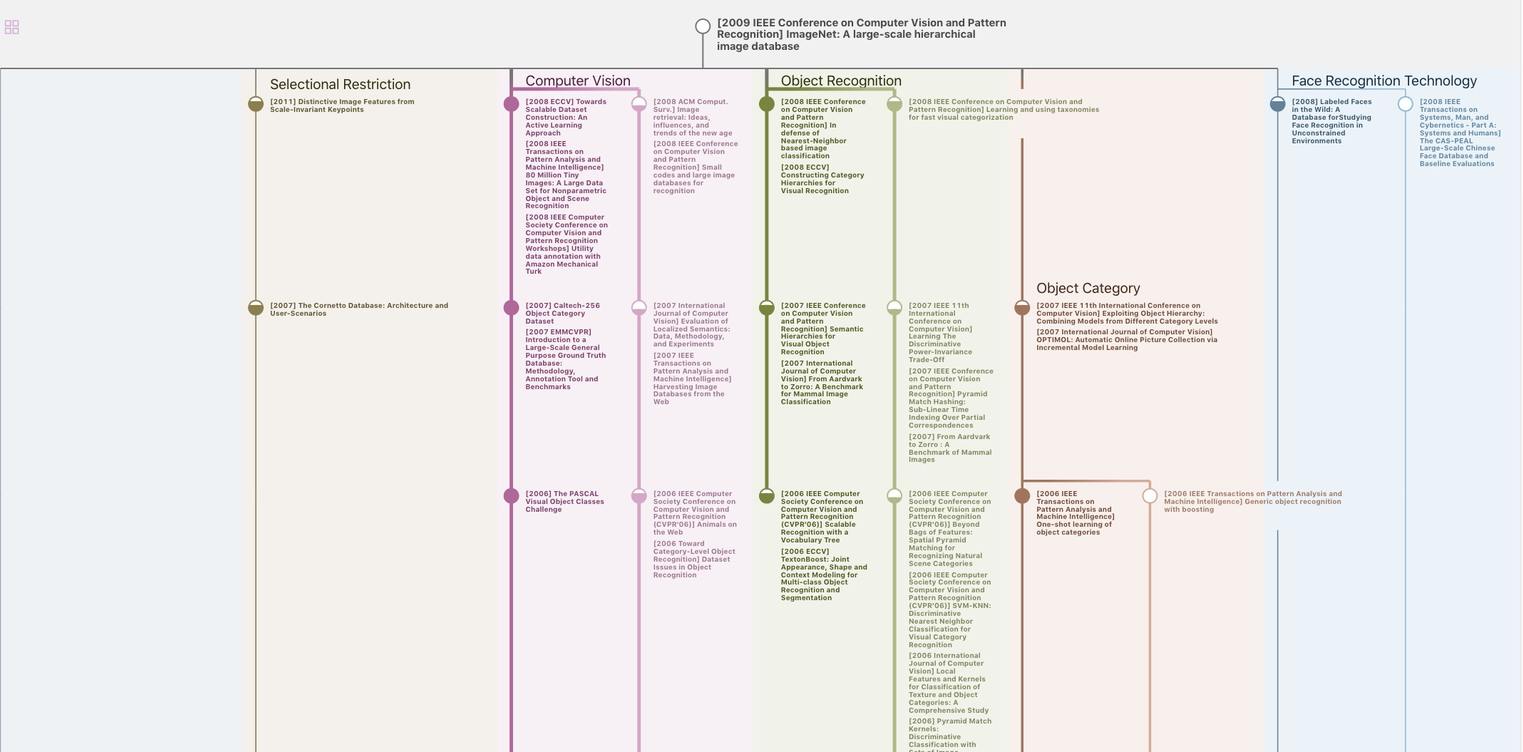
生成溯源树,研究论文发展脉络
Chat Paper
正在生成论文摘要