Risk-Averse Trajectory Optimization Via Sample Average Approximation
ICRA 2024(2024)
Abstract
Trajectory optimization under uncertainty underpins a wide range of applications in robotics. However, existing methods are limited in terms of reasoning about sources of epistemic and aleatoric uncertainty, space and time correlations, nonlinear dynamics, and non-convex constraints. In this work, we first introduce a continuous-time planning formulation with an average-value-at-risk constraint over the entire planning horizon. Then, we propose a sample-based approximation that unlocks an efficient and general-purpose algorithm for risk-averse trajectory optimization. We prove that the method is asymptotically optimal and derive finite-sample error bounds. Simulations demonstrate the high speed and reliability of the approach on problems with stochasticity in nonlinear dynamics, obstacle fields, interactions, and terrain parameters.
MoreTranslated text
Key words
Optimization and Optimal Control,Planning under Uncertainty,Probability and Statistical Methods
AI Read Science
Must-Reading Tree
Example
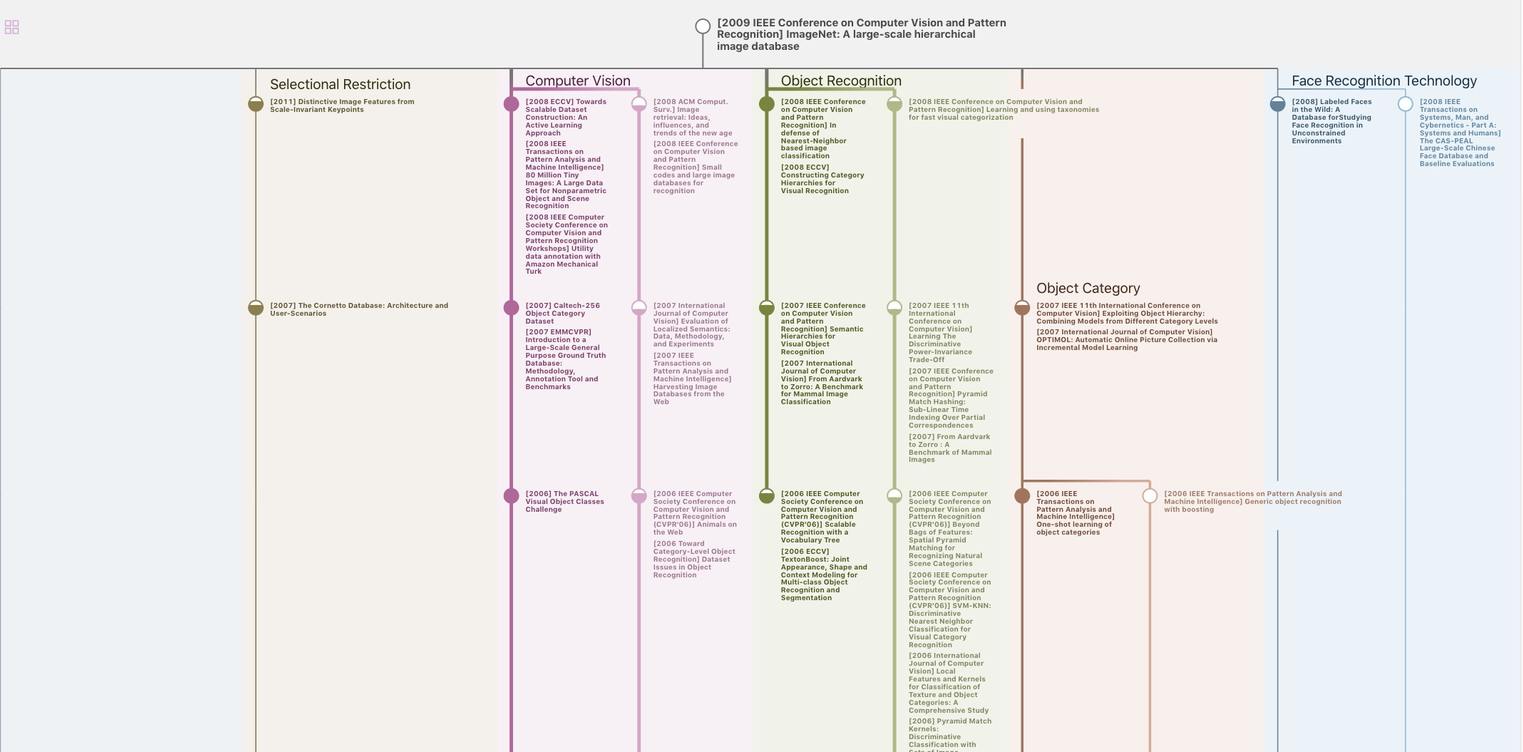
Generate MRT to find the research sequence of this paper
Chat Paper
Summary is being generated by the instructions you defined