Validation of machine learning models to predict dementia-related neuropsychiatric symptoms in real-world data
crossref(2020)
摘要
Abstract Background Neuropsychiatric symptoms (NPS) are the leading cause of the social burden of dementia but their role is underestimated. The objective of the study was to validate predictive models to separately identify psychotic and depressive symptoms in patients diagnosed with dementia using clinical databases representing the whole population (real-world data). Methods First, we searched the electronic health records of 4,003 patients with dementia to identify NPS. Second, machine learning (random forest) algorithms were applied to build in the training sample (N=3,003) separate predictive models for psychotic and depressive symptoms. In order to evaluate the classification ability of the models, the following statistics were calculated for each model: the area under the receiver operating curve (AUC), sensitivity, specificity, accuracy, no-information rate and Kappa index. Third, calibration and discrimination were assessed in the validation sample (N= 1,000) to assess the performance of the models. A calibration curve was drawn by plotting the predicted probabilities for groups on the x-axis and the mean observed values on the y-axis. Results Neuropsychiatric symptoms were noted in the electronic health record of 58% of patients. The AUC reached 0.80 for the psychotic symptoms model and 0.74 for the depressive symptoms model. The Kappa index and accuracy also showed better discrimination in the psychotic model. Calibration plots indicated that both types of model had less predictive accuracy when the probability of neuropsychiatric symptoms was < 25%. The most important variables in the psychotic symptom model were use of risperidone, level of sedation, quetiapine and haloperidol and the number of antipsychotics prescribed. In the depressive symptom model, the most important variable was number of antidepressants prescribed, use of escitalopram, level of sedation and age. Conclusions More than half of the sample had NPS as identified by the presence of key terms in the electronic health record. Although NPS are not encoded, they are treated with antipsychotics and antidepressants, which allows developing valid predictive models by joining machine learning tools and real-world data. Given their good performance, the predictive models can be used to estimate prevalence of NPS in population databases.
更多查看译文
AI 理解论文
溯源树
样例
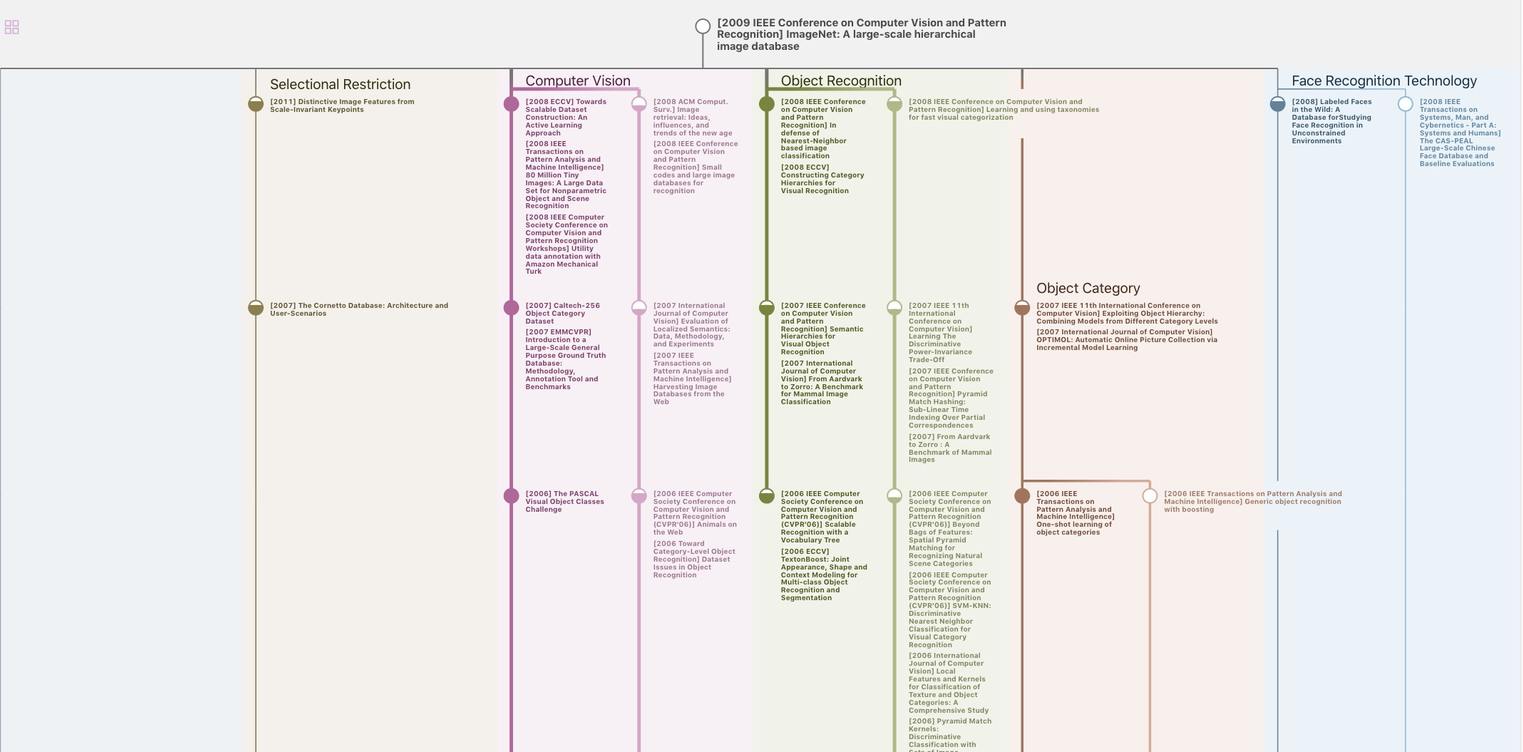
生成溯源树,研究论文发展脉络
Chat Paper
正在生成论文摘要