Mitigating Calibration Bias Without Fixed Attribute Grouping for Improved Fairness in Medical Imaging Analysis
MEDICAL IMAGE COMPUTING AND COMPUTER ASSISTED INTERVENTION, MICCAI 2023, PT III(2023)
摘要
Trustworthy deployment of deep learning medical imaging models into real-world clinical practice requires that they be calibrated. However, models that are well calibrated overall can still be poorly calibrated for a sub-population, potentially resulting in a clinician unwittingly making poor decisions for this group based on the recommendations of the model. Although methods have been shown to successfully mitigate biases across subgroups in terms of model accuracy, this work focuses on the open problem of mitigating calibration biases in the context of medical image analysis. Our method does not require subgroup attributes during training, permitting the flexibility to mitigate biases for different choices of sensitive attributes without re-training. To this end, we propose a novel two-stage method: Cluster-Focal to first identify poorly calibrated samples, cluster them into groups, and then introduce group-wise focal loss to improve calibration bias. We evaluate our method on skin lesion classification with the public HAM10000 dataset, and on predicting future lesional activity for multiple sclerosis (MS) patients. In addition to considering traditional sensitive attributes (e.g. age, sex) with demographic subgroups, we also consider biases among groups with different image-derived attributes, such as lesion load, which are required in medical image analysis. Our results demonstrate that our method effectively controls calibration error in the worst-performing subgroups while preserving prediction performance, and outperforming recent baselines.
更多查看译文
关键词
Fairness,Bias,Calibration,Uncertainty,Multiple Sclerosis,Skin Lesion,Disease activity prediction
AI 理解论文
溯源树
样例
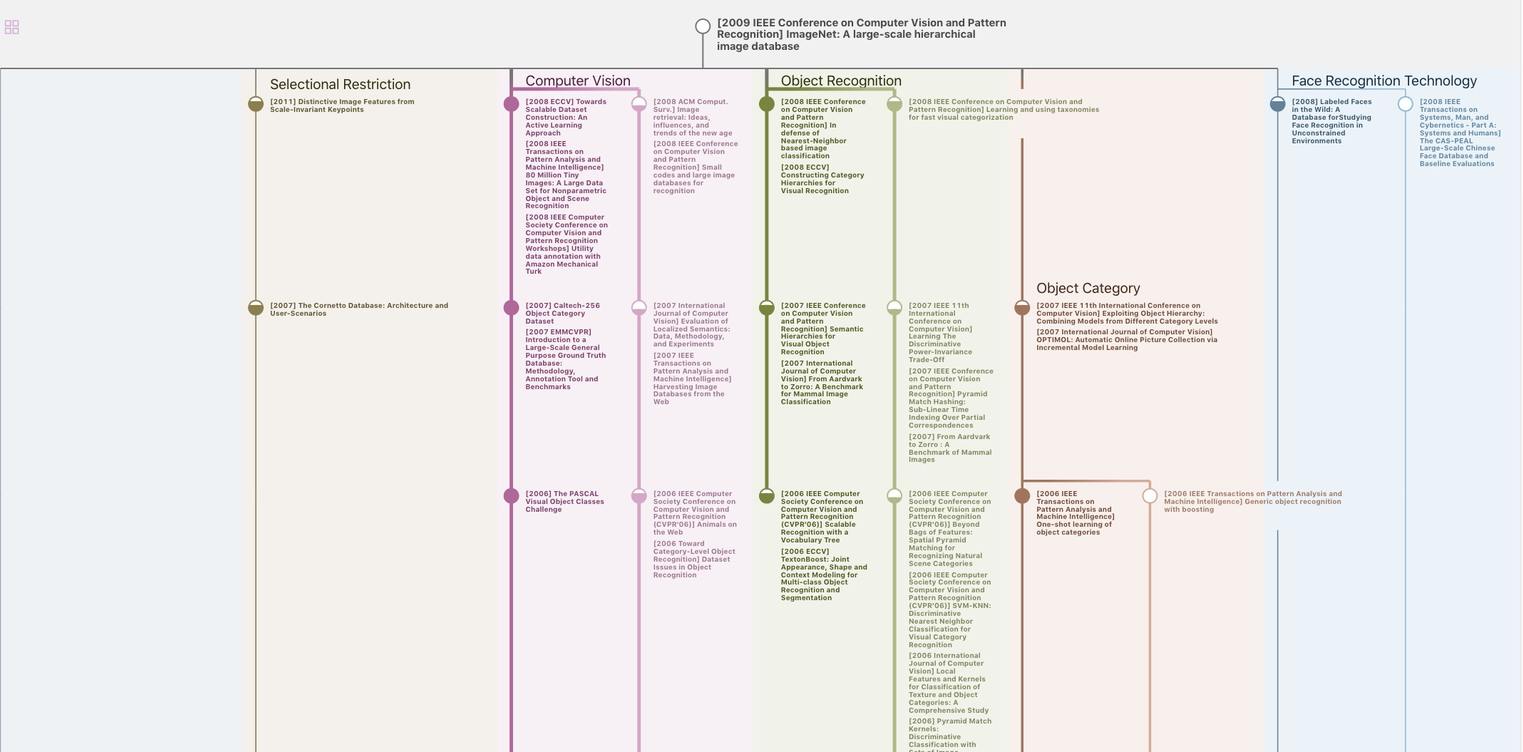
生成溯源树,研究论文发展脉络
Chat Paper
正在生成论文摘要