Protein Abundance Prediction Through Machine Learning Methods
bioRxiv (Cold Spring Harbor Laboratory)(2020)
摘要
Proteins are responsible for most physiological processes, and their abundance provides crucial information for systems biology research. However, absolute protein quantification, as determined by mass spectrometry, still has limitations in capturing the protein pool. Protein abundance is impacted by translation kinetics, which rely on features of codons. In this study, we evaluated the effect of codon usage bias of genes on protein abundance. Notably, we observed differences regarding codon usage patterns between genes coding for highly abundant proteins and genes coding for less abundant proteins. Analysis of synonymous codon usage and evolutionary selection showed a clear split between the two groups. Our machine learning models predicted protein abundances from codon usage metrics with remarkable accuracy, achieving R2 values higher than previously reported in the literature. Upon integration of the predicted protein abundance in enzyme-constrained genome-scale metabolic models, the simulated phenotypes closely matched experimental data, which demonstrates that our predictive models are valuable tools for systems metabolic engineering approaches.
更多查看译文
关键词
protein abundance prediction,machine learning
AI 理解论文
溯源树
样例
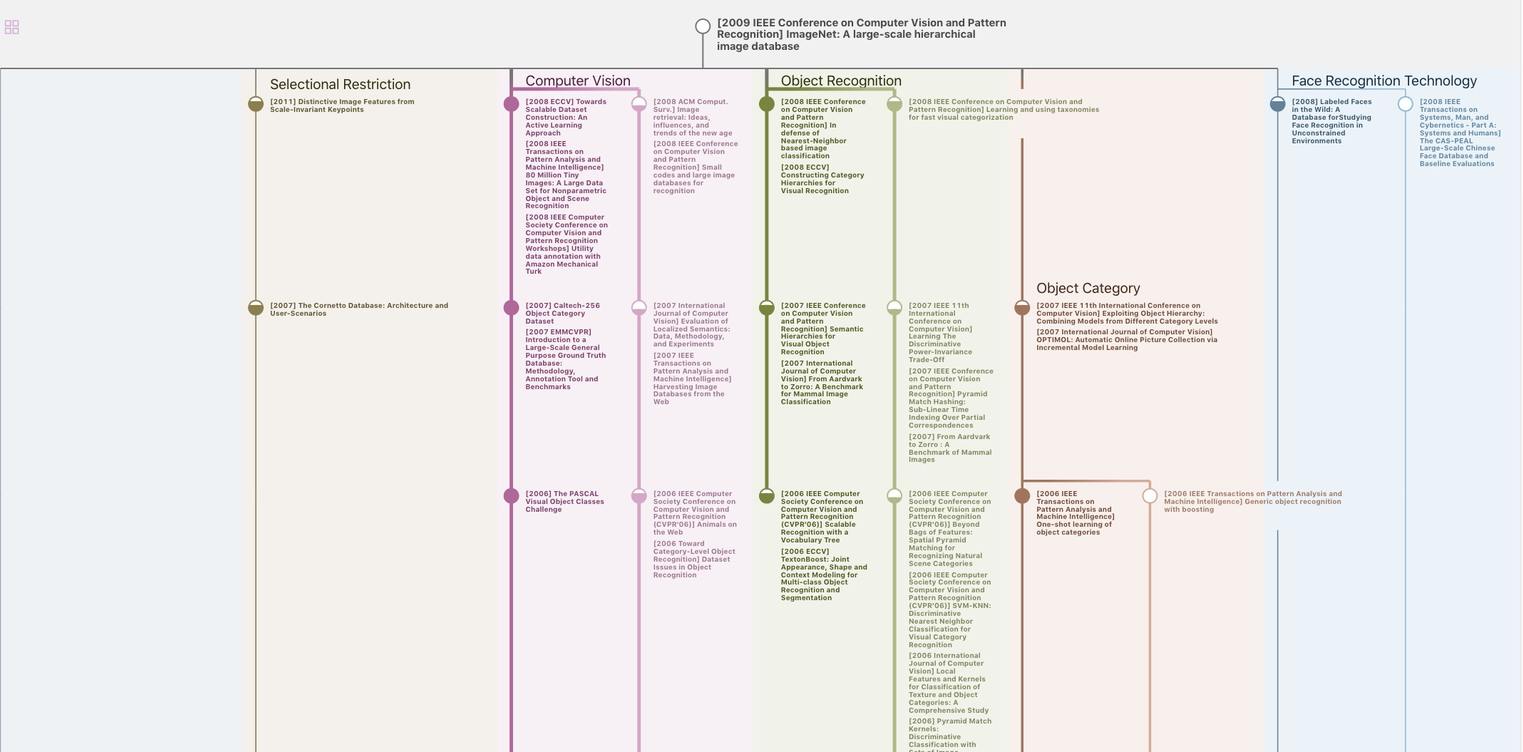
生成溯源树,研究论文发展脉络
Chat Paper
正在生成论文摘要