Scalable Computing of Betweenness Centrality based on Graph Reduction with a Case Study on Breast Cancer Analytics
Research Square (Research Square)(2020)
摘要
Abstract BackgroundGraph theory has been widely applied to the studies in biomedicine such as structural measures including betweenness centrality. However, if the network size is too large, the result of betweenness centrality would be difficult to obtain in a reasonable amount of time.ResultIn this paper, we describe an approach, 1+ɛ lossy graph reduction algorithm, to computing betweenness centrality on large graphs. The approach is able to guarantee a bounded approximation result. We use GSE48216, a breast cancer cell line co-expression network, to show that our algorithms can achieve a higher reduction rate with a trade-off of some bounded errors in query results. Furthermore, by comparing the betweenness centrality of the original graph and the reduced graph, it can be shown that a higher reduction rate does not sacrifice the accuracy of betweenness centrality when providing faster execution time.ConclusionsOur proposed 1+ɛ lossy graph reduction algorithm is validated by the experiment results which show that the approach achieves a faster execution within a bounded error rate.
更多查看译文
关键词
betweenness centrality,graph reduction,scalable computing,analytics
AI 理解论文
溯源树
样例
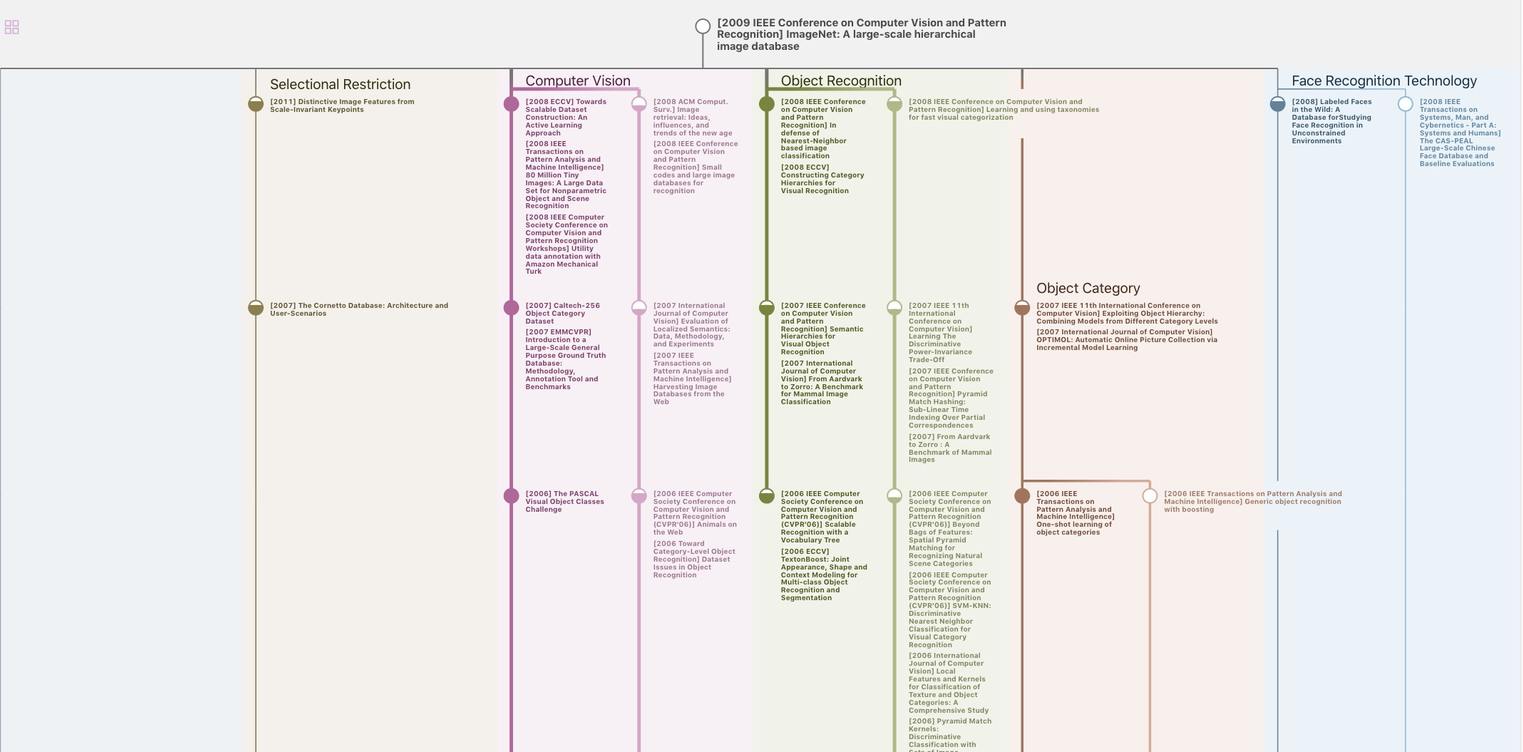
生成溯源树,研究论文发展脉络
Chat Paper
正在生成论文摘要