NMFNA: a non-negative matrix factorization network analysis method for identifying communities and characteristic genes from pancreatic cancer data
crossref(2020)
摘要
Abstract Background: Pancreatic cancer (PC) is a common type of digestive system disease. Comprehensive analysis of different types of PC genetic data plays a crucial role in understanding its biological mechanisms. Currently, Non-negative Matrix Factorization (NMF) based methods are widely used for data analysis. Nevertheless, it is a challenge for them to integrate and decompose different types of data simultaneously.Results: In this paper, a Non-negative Matrix Factorization Network Analysis method, NMFNA, is proposed, which introduces a graph regularized constraint to NMF, for identifying communities and characteristic genes from two-type PC data. Firstly, three PC networks, i.e., methylation network (ME), copy number variation network (CNV), and the bipartite network between them, are constructed by both ME and CNV data of PC downloaded from the TCGA database, using the Pearson Correlation Coefficient. Then, the NMFNA is proposed to detect core communities and characteristic genes from these three PC networks effectively due to its introduced graph regularized constraint, which is the highlight of NMFNA. Finally, both gene ontology enrichment analysis and pathway enrichment analysis are performed to deeply understand the biological functions of detected core communities.Conclusions: Experimental results demonstrated that the NMFNA facilitates the integration and decomposition of two types of PC data simultaneously and can serve as an alternative method for detecting communities and characteristic genes from multiple genetic networks. The data and demo codes of NMFNA are available online at https://github.com/CDMB-lab/NMFNA.
更多查看译文
AI 理解论文
溯源树
样例
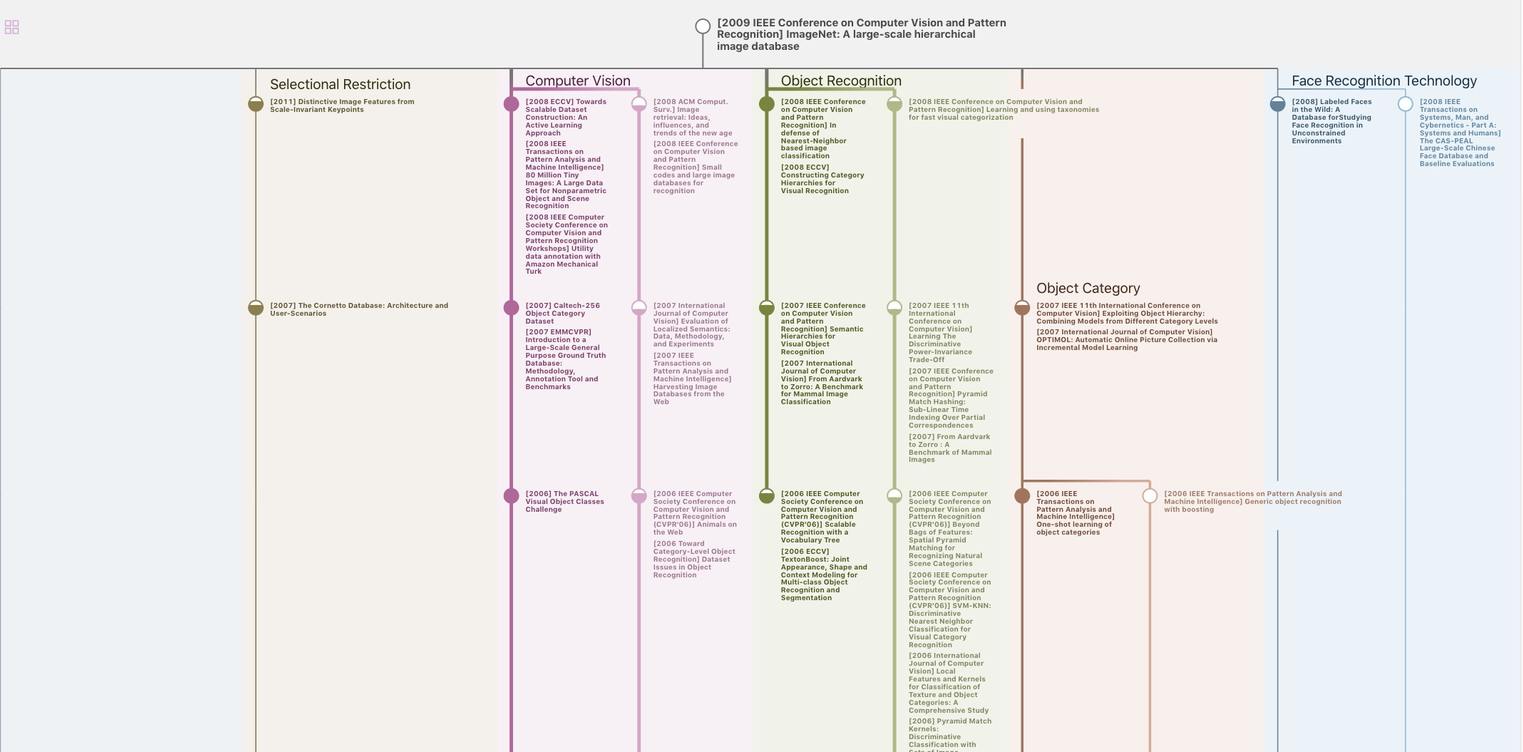
生成溯源树,研究论文发展脉络
Chat Paper
正在生成论文摘要