Using absorbance peak of carbonate to select suitable regression model before predicting soil inorganic carbon concentration by mid-infrared reflectance spectroscopy
crossref(2021)
摘要
<p><span>Mid-Infrared reflectance spectroscopy (MIRS, 4000 – 400 cm<sup>-1</sup>) is being considered to provide accurate estimations of soil inorganic carbon (SIC) contents. Usually, the prediction performances by MIRS are analyzed using figures of merit based on entire test datasets characterized by large SIC ranges, without paying attention to performances at sub-range scales. This work aims to <em>1)</em> evaluate the performances of MIR regression models for SIC prediction, for a large range of SIC test data (0-100 g/kg) and for several regular sub-ranges of SIC values (0-5, 5-10, 10-15 g/kg, etc.) and <em>2)</em> adapt the prediction model depending on sub-ranges of test samples, using the absorbance peak at 2510 cm<sup>-1</sup> for separating SIC-poor and SIC-rich test samples. This study used a Tunisian MIRS topsoil dataset including 96 soil samples, mostly rich in SIC, to calibrate and validate SIC prediction models; and a French MIRS topsoil dataset including 2178 soil samples, mostly poor in SIC, to test them. Two following regression models were used: a partial least squares regression (PLSR) using the entire spectra and a simple linear regression (SLR) using the height of the carbonate absorbance peak at 2150 cm<sup>-1</sup>.</span></p><p><span>First, our results showed that PLSR provided <em>1) </em>better performances than SLR on the Validation Tunisian dataset (R<sup>2</sup><sub>test</sub> of 0.99 vs. 0.86, respectively), but <em>2) </em>lower performances than SLR on the Test French dataset (R<sup>2</sup><sub>test</sub> of 0.70 vs. 0.91, respectively). Secondly, our results showed that on the Test French dataset, predicted SIC values were more accurate for SIC-poor samples (< 15 g/kg) with SLR (RMSE<sub>test</sub> from 1.5 to 7.1 g/kg, depending on the sub-range) than with PLSR prediction model (RMSE<sub>test </sub>from 7.3 to 14.8 g/kg, depending on the sub-range). Conversely, predicted SIC values were more accurate for carbonated samples (> 15 g/kg) with PLSR (RMSE<sub>test</sub> from 4.4 to 10.1 g/kg, depending on the sub-range) than with SLR prediction model (RMSE<sub>test</sub> from 6.8 to 14 g/kg, depending on the sub-range). Finally, our results showed that the absorbance peak at 2150 cm<sup>-1</sup> could be used before prediction to separate SIC-poor and SIC-rich test samples (452 and 1726 samples, respectevely). The SLR and PLSR regression methods applied to these SIC-poor and SIC-rich test samples, respectively, provided better prediction performances (<em>R²</em><sub><em>test </em></sub>of 0.95 and <em>RMSE</em><sub><em>test</em></sub> of 3.7 g/kg<sup></sup>). </span></p><p><span>Finally, this study demonstrated that the use of the spectral absorbance peak at 2150 cm<sup>-1</sup> provided useful information on Test samples and helped the selection of the optimal prediction model depending on SIC level, when using calibration and test sample sets with very different SIC distributions.</span></p>
更多查看译文
AI 理解论文
溯源树
样例
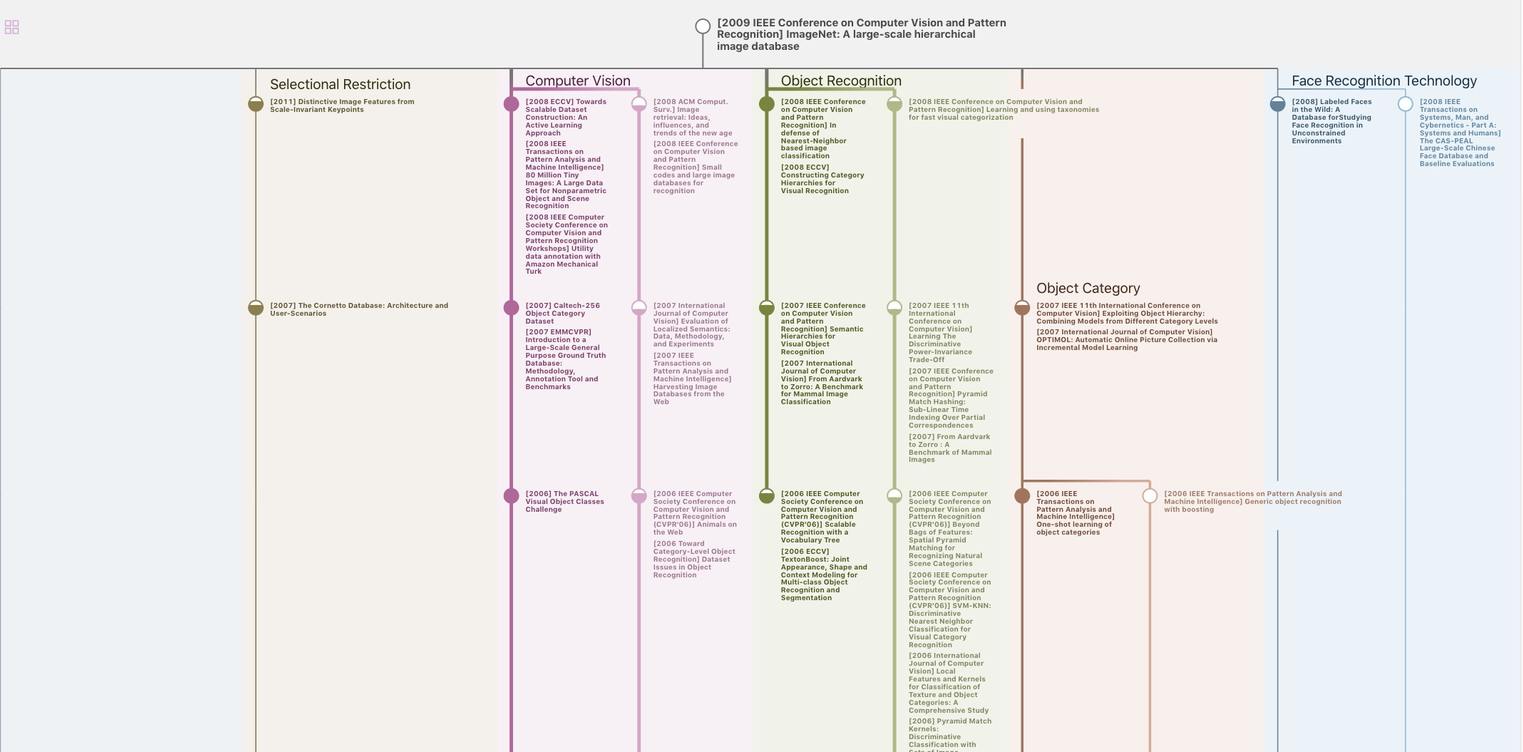
生成溯源树,研究论文发展脉络
Chat Paper
正在生成论文摘要