Identification of Markers that Distinguish Adipose Tissue and Glucose and Insulin Metabolism using a Multi-Modal Machine Learning Approach
Research Square (Research Square)(2021)
摘要
Abstract Background The study of metabolomics has improved our knowledge of the biology behind type 2 diabetes and its related metabolic dysregulations. We aimed to investigate markers of adipose tissue morphology dysfunction and insulin and glucose metabolism dysfunction in 53 non-obese male individuals.Methods The participants underwent extensive clinical, biochemical and magnetic resonance imaging phenotyping, and we also investigated non-targeted serum metabolites. We used a multi-modal machine learning approach to evaluate which serum metabolomic compounds predicted markers of glucose and insulin metabolism, adipose tissue morphology and distribution.Results Fasting glucose was associated with metabolites of intracellular insulin action and beta cell dysfunction, namely cysteine-s-sulphate and n-acetylgarginine. Fasting insulin was predicted by eugenol, a metabolite of beta-oxidation of fatty acids. OGTT glucose levels at 30 minutes were predicted by 7-Hoca and taurochenodeoxycholate, microbiota derived metabolites, as well as, fatty acid chains. Both insulin clamp and HOMA-IR were predicted by metabolites involved in beta-oxidation of fatty acids and biodegradation of triacylglycerol, namely tartrate and 3-phosphoglycerate, as well as pyruvate. OGTT glucose area under curve (AUC) was associated with bile acid metabolites and OGTT insulin AUC was predicted by subcutaneous adipocyte size as well as a metabolite of sphingolipid metabolism. Finally, subcutaneous adipocyte size was associated with several long chain fatty acids, metabolites involved in fatty acid biosynthesis, markers of beta-oxidation of fatty acids, as well as markers of sphingolipid metabolism. Lipid oxidation metabolites also predicted liver lipid accumulation, and cardiac lipid storage was predicted by a metabolite of branched chain amino acid (BCAA) turnover. Only adipocyte cell size, age and alpha-tocopherol were associated with visceral fat.Conclusions We identified several biomarkers associated with markers of dysfunction in adipose tissue and insulin and glucose metabolism using a multi-modal machine learning approach. Our approach demonstrated the relative importance of serum metabolites and they outperformed traditional clinical and biochemical variables for most endpoints.
更多查看译文
关键词
distinguish adipose tissue,adipose tissue,insulin metabolism,multi-modal
AI 理解论文
溯源树
样例
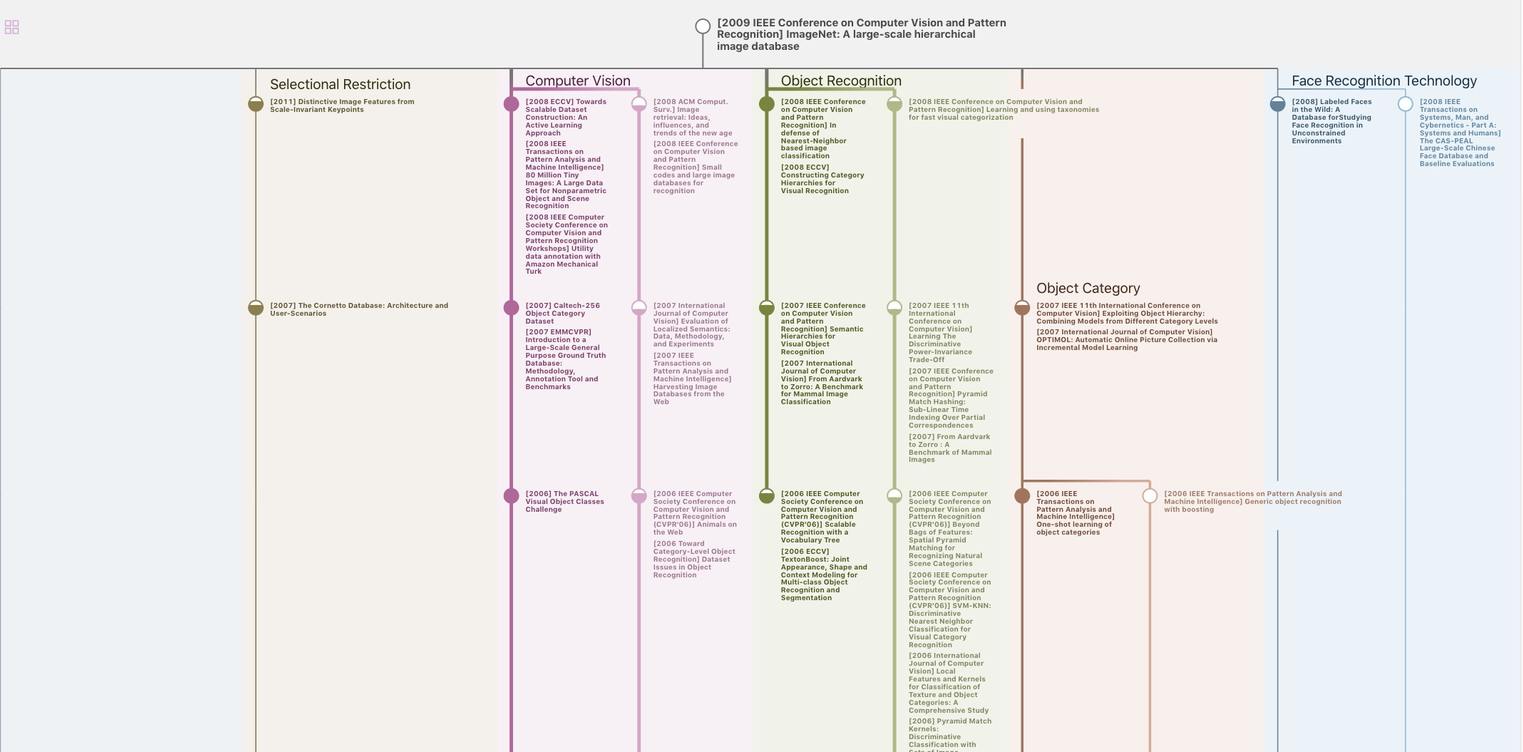
生成溯源树,研究论文发展脉络
Chat Paper
正在生成论文摘要