Intelligent Interpretation of Veterinary Musculoskeletal X-rays Trained with Human Thoracic Limb X-rays
crossref(2021)
摘要
Abstract Deep learning (DL) using convolutional neural networks (CNNs) is being widely applied to assist in the interpretation of medical images in modern healthcare but there is a paucity of ‘artificial intelligence’ being currently applied to veterinary medicine. Most veterinary musculoskeletal (MSK) x-ray imaging is done in a community setting and there has not been the development of large image repositories that are available in human healthcare. Domestic animals – cats and dogs – however, have similar skeletal anatomies to humans. Hence, we hypothesized that annotated human MSK x-rays (Xs) could perhaps be used as a surrogate for the lack of adequate veterinary data to develop an automated system to help interpret veterinary MSK Xs. We refer to this as ‘intelligent interpretation of Veterinary Musculoskeletal Xs (iiVetMSK-Xs). Our iiVetMSK-Xs has an x-ray classifier built on EfficientNet and a lesion localizer built on gradient-weight class activation mapping (Grad-CAM). We used the human Musculoskeletal Radiograph (MURA) dataset (40,005 thoracic limb Xs) and a small veterinary x-ray dataset (500 thoracic and pelvic limb Xs from 141 cats and dogs) downloaded from online case repositories. Our results show that using the human thoracic limb Xs and the veterinary dataset to train a CNN improved diagnostic accuracy threefold. We suggest that our iiVetMSK-Xs is an important first step in developing automated image interpretation for veterinary imaging.
更多查看译文
AI 理解论文
溯源树
样例
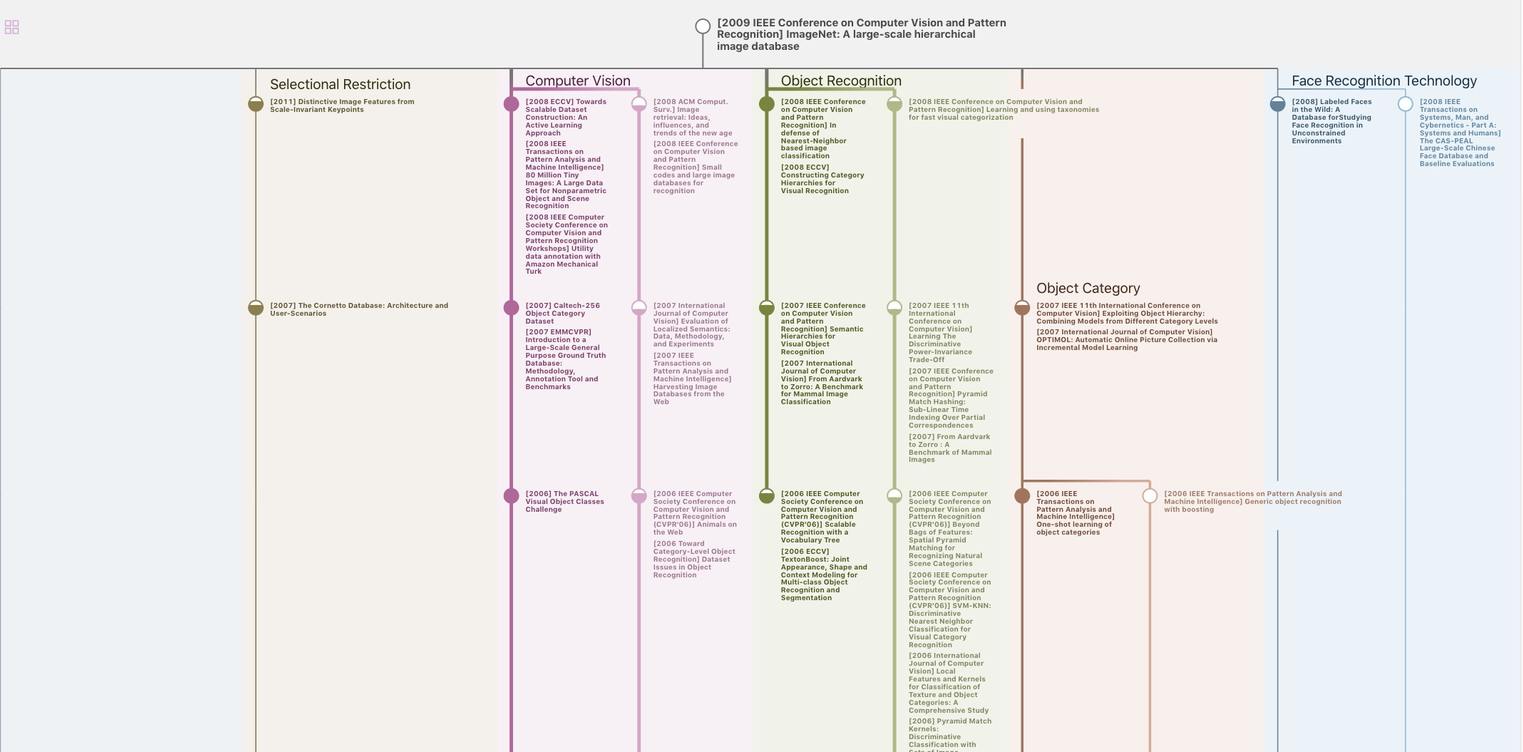
生成溯源树,研究论文发展脉络
Chat Paper
正在生成论文摘要